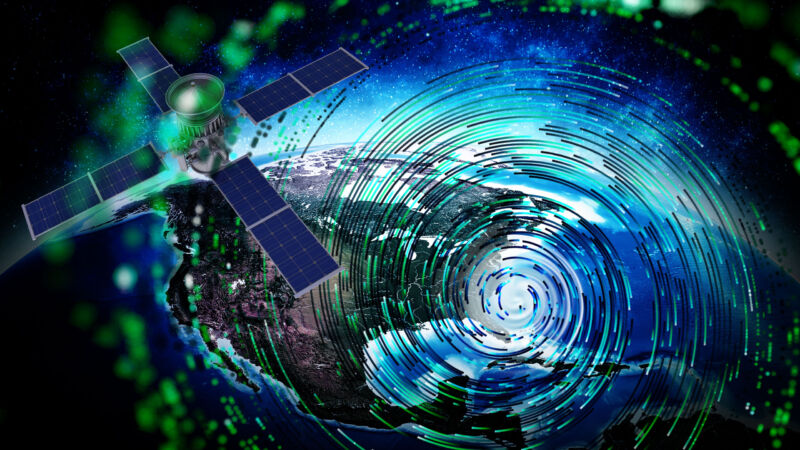
放大 / 人工智慧氣象模型於 2024 年大西洋颶風季及時到來。
Much like the invigorating passage of a strong cold front, major changes are afoot in the weather forecasting community. And the end game is nothing short of revolutionary: an entirely new way to forecast weather based on artificial intelligence that can run on a desktop computer.
就像強冷鋒帶來令人振奮的變化一樣,氣象預報界也正在發生重大變化。最終目標是一場徹底的革命:一種完全基於人工智能的全新天氣預報方式,可以在台式電腦上運行。
Today's artificial intelligence systems require one resource more than any other to operate—data. For example, large language models such as ChatGPT voraciously consume data to improve answers to queries. The more and higher quality data, the better their training, and the sharper the results.
今天的人工智能系統比任何其他系統都需要一種資源來運作——數據。例如,像 ChatGPT 這樣的大語言模型會大量消耗數據來改進查詢的答案。數據越多、質量越高,它們的訓練就越好,結果也就越精確。
However, there is a finite limit to quality data, even on the Internet. These large language models have hoovered up so much data that they're being sued widely for copyright infringement. And as they're running out of data, the operators of these AI models are turning to ideas such as synthetic data to keep feeding the beast and produce ever more capable results for users.
然而,即使在互聯網上,優質數據也是有限的。這些大型語言模型已經吸收了如此多的數據,以至於它們正被廣泛起訴侵犯版權。隨著數據的耗盡,這些人工智能模型的運營商正轉向合成數據等想法,以繼續餵養這個“巨獸”,並為用戶產生更強大的結果。
If data is king, what about other applications for AI technology similar to large language models? Are there untapped pools of data? One of the most promising that has emerged in the last 18 months is weather forecasting, and recent advances have sent shockwaves through the field of meteorology.
如果數據為王,那麼人工智能技術的其他應用(類似於大型語言模型)又如何呢?是否存在尚未開發的數據庫?在過去 18 個月中出現的最有希望的領域之一是天氣預報,最近的進展給氣象學界帶來了衝擊波。
That's because there's a secret weapon: an extremely rich data set. The European Centre for Medium-Range Weather Forecasts, the premiere organization in the world for numerical weather prediction, maintains a set of data about atmospheric, land, and oceanic weather data for every day, at points around the world, every few hours, going back to 1940. The last 50 years of data, after the advent of global satellite coverage, is especially rich. This dataset is known as ERA5, and it is publicly available.
這是因為有一個秘密武器:一個極其豐富的數據集。歐洲中期天氣預報中心 (ECMWF) 是世界上首屈一指的數值天氣預報機構,它維護著一個關於大氣、陸地和海洋天氣數據的數據集,這些數據涵蓋了全球各地每天每隔幾個小時從 1940 年至今的數據。在全球衛星覆蓋出現之後的過去 50 年的數據尤其豐富。這個數據集被稱為 ERA5,它是公開可用的。
It was not created to fuel AI applications, but ERA5 has turned out to be incredibly useful for this purpose. Computer scientists only really got serious about using this data to train AI models to forecast the weather in 2022. Since then, the technology has made rapid strides. In some cases, the output of these models is already superior to global weather models that scientists have labored decades to design and build, and they require some of the most powerful supercomputers in the world to run.
它不是為人工智能應用而創建的,但 ERA5 已被證明對此非常有用。計算機科學家直到 2022 年才真正開始認真地使用這些數據來訓練人工智能模型來預測天氣。從那時起,這項技術取得了飛速的進步。在某些情況下,這些模型的輸出已經優於科學家們花費數十年時間設計和構建的全球天氣模型,而這些模型需要一些世界上最強大的超級計算機才能運行。
"It is clear that machine learning is a significant part of the future of weather forecasting," said Matthew Chantry, who leads AI forecasting efforts at the European weather center known as ECMWF, in an interview with Ars.
“很明顯,機器學習是天氣預報未來的一個重要組成部分,”領導歐洲天氣預報中心 (ECMWF) 人工智能預報工作的 Matthew Chantry 在接受 Ars 採訪時表示。
It’s moving fast 它正在快速發展
John Dean and Kai Marshland met as undergraduates at Stanford University in the late 2010s. Dean, an electrical engineer, interned at SpaceX during the summer of 2017. Marshland, a computer scientist, interned at the launch company the next summer. Both graduated in 2019 and were trying to figure out what to do with their lives.
John Dean 和 Kai Marshland 在 2010 年代後期於史丹佛大學就讀大學時相識。Dean 是一名電機工程師,在 2017 年夏天於 SpaceX 實習。Marshland 是一名電腦科學家,則於次年夏天在這家發射公司實習。兩人皆於 2019 年畢業,並正在思考未來的人生方向。
"We decided we wanted to solve the problem of weather uncertainty," Marshland said, so they co-founded a company called WindBorne Systems.
「我們決定要解決天氣的不確定性問題,」Marshland 表示,因此他們共同創立了一家名為 WindBorne Systems 的公司。
The premise of the company was simple: For about 85 percent of the Earth and its atmosphere, we have no good data about weather conditions there. A lack of quality data, which establishes initial conditions, represents a major handicap for global weather forecast models. The company's proposed solution was in its name—wind borne.
這家公司的理念很簡單:地球及其大氣層約有 85% 的區域缺乏良好的天氣狀況數據。缺乏建立初始條件的品質數據是全球天氣預報模型的一大障礙。該公司的解決方案就在其名稱中:wind borne(風載)。
Dean and Marshland set about designing small weather balloons they could release into the atmosphere and which would fly around the world for up to 40 days, relaying useful atmospheric data that could be packaged and sold to large, government-funded weather models.
Dean 和 Marshland 著手設計可以釋放到大氣中並環繞世界飛行長達 40 天的小型氣象氣球,這些氣球會傳回有用的氣象數據,這些數據可以打包出售給大型、政府資助的天氣模型。
Weather balloons provide invaluable data about atmospheric conditions—readings such as temperature, dewpoints, and pressures—that cannot be captured by surface observations or satellites. Such atmospheric "profiles" are helpful in setting the initial conditions models start with. The problem is that traditional weather balloons are cumbersome and only operate for a few hours. Because of this, the National Weather Service only launches them twice daily from about 100 locations in the United States.
氣象氣球提供了關於大氣條件的寶貴數據,例如溫度、露點和氣壓等讀數,這些數據是地面觀測或衛星無法捕捉到的。這些大氣「剖面」有助於設定模型開始使用的初始條件。問題是傳統的氣象氣球既笨重又只能運行幾個小時。因此,美國國家氣象局每天只在美國約 100 個地點釋放兩次氣象氣球。
reader comments 讀者評論
181