100,000 H100 Clusters: Power, Network Topology, Ethernet vs InfiniBand, Reliability, Failures, Checkpointing
100,000 H100 集群:电源、网络拓扑、以太网与 InfiniBand、可靠性、故障、检查点
Frontier Model Scaling Challenges and Requirements, Fault Recovery through Memory Reconstruction, Rack Layouts
前沿模型扩展挑战与要求,通过内存重建进行故障恢复,机架布局
There is a camp that feels AI capabilities have stagnated ever since GPT-4’s release. This is generally true, but only because no one has been able to massively increase the amount of compute dedicated to a single model. Every model that has been released is roughly GPT-4 level (~2e25 FLOP of training compute). This is because the training compute dedicated to these models have also been roughly the same level. In the case of Google’s Gemini Ultra, Nvidia Nemotron 340B, and Meta LLAMA 3 405B, the FLOPS dedicated were of similar magnitude or even higher when compared to GPT-4, but an inferior architecture was utilized, resulting in these models falling short of unlocking new capabilities.
有一种观点认为,自从 GPT-4 发布以来,人工智能的能力停滞不前。这在一定程度上是正确的,但仅仅是因为没有人能够大幅增加专门用于单一模型的计算量。每个发布的模型大致都处于 GPT-4 水平(约 2e25 FLOP 的训练计算)。这是因为这些模型所专用的训练计算量也大致相同。在谷歌的 Gemini Ultra、英伟达的 Nemotron 340B 和 Meta 的 LLAMA 3 405B 的情况下,专用的 FLOPS 与 GPT-4 相比大致相当甚至更高,但使用了较差的架构,导致这些模型未能解锁新能力。
While OpenAI has gotten more compute, they have mostly directed it at bringing smaller, overtrained, cheaper to inference models such as GPT-4 Turbo and GPT-4o. OpenAI admits they only just started training the next tier of model recently.
虽然 OpenAI 获得了更多的计算能力,但他们主要将其用于开发更小、过度训练、推理成本更低的模型,如 GPT-4 Turbo 和 GPT-4o。OpenAI 承认他们最近才刚开始训练下一层级的模型。
The obvious next step for AI is to train a multi-trillion parameter multimodal transformer with massive amounts of video, image, audio, and text. No one has completed this task yet, but there is a flurry of activity in the race to be first.
AI 的明显下一步是训练一个多万亿参数的多模态变换器,使用大量的视频、图像、音频和文本。目前还没有人完成这个任务,但在争夺首位的竞赛中活动频繁。
Multiple large AI labs including but not limited to OpenAI/Microsoft, xAI, and Meta are in a race to build GPU clusters with over 100,000 GPUs. These individual training clusters cost in excess of $4 billion of server capital expenditures alone, but they are also heavily limited by the lack of datacenter capacity and power as GPUs generally need to be co-located for high-speed chip to chip networking. A 100,000 GPU cluster will require >150MW in datacenter capacity and guzzle down 1.59 terawatt hours in a single year, costing $123.9 million at a standard rate of $0.078/kWh.
多个大型人工智能实验室,包括但不限于 OpenAI/微软、xAI 和 Meta,正在竞相建立超过 100,000 个 GPU 的集群。这些单独的训练集群仅服务器资本支出就超过 40 亿美元,但由于缺乏数据中心容量和电力,它们也受到严重限制,因为 GPU 通常需要共同放置以实现高速芯片间网络。一个 100,000 个 GPU 的集群将需要超过 150MW 的数据中心容量,并在一年内消耗 1.59 太瓦时,按标准费率 0.078 美元/千瓦时计算,费用为 1.239 亿美元。
Today we will dive into large training AI clusters and the infrastructure around them. Building these clusters is a lot more complicated than just throwing money at the problem. Achieving high utilization with them is even more difficult due to the high failure rates of various components, especially networking. We will also walk through power challenges, reliability, checkpointing, network topology options, parallelism schemes, rack layouts, and total bill of materials for these systems. Over a year ago, we covered Nvidia’s InfiniBand problem which resulted in some companies choosing Spectrum-X Ethernet over InfiniBand. We will also cover the major flaw with Spectrum-X which has hyperscalers going with Broadcom’s Tomahawk 5.
今天我们将深入探讨大型训练 AI 集群及其周围的基础设施。构建这些集群远比仅仅投入资金要复杂得多。由于各种组件的高故障率,尤其是网络,达到高利用率更是困难。我们还将讨论电力挑战、可靠性、检查点、网络拓扑选项、并行方案、机架布局以及这些系统的总材料清单。一年多前,我们讨论了 Nvidia 的 InfiniBand 问题,这导致一些公司选择 Spectrum-X 以太网而非 InfiniBand。我们还将讨论 Spectrum-X 的主要缺陷,这使得超大规模公司选择了 Broadcom 的 Tomahawk 5。
To put in perspective how much compute a 100,000 GPU cluster can provide, OpenAI’s training BF16 FLOPS for GPT-4 was ~2.15e25 FLOP (21.5 million ExaFLOP), on ~20,000 A100s for 90 to 100 days. That cluster only had 6.28 BF16 ExaFLOP/second peak throughput. On a 100k H100 cluster, this number would soar to 198/99 FP8/FP16 ExaFLOP/second. This is a 31.5x increase in peak theoretical AI training FLOPs compared to the 20k A100 cluster.
为了让人们理解一个 10 万 GPU 集群能提供多少计算能力,OpenAI 在大约 20,000 个 A100 上训练 GPT-4 时的 BF16 FLOPS 约为 2.15e25 FLOP(2150 万 ExaFLOP),持续了 90 到 100 天。该集群的峰值吞吐量仅为 6.28 BF16 ExaFLOP/秒。在一个 10 万 H100 集群上,这个数字将飙升至 198/99 FP8/FP16 ExaFLOP/秒。这与 20,000 个 A100 集群相比,峰值理论 AI 训练 FLOP 增加了 31.5 倍。
On H100s, AI labs are achieving FP8 Model FLOPs Utilization (MFU) as high as 35% and FP16 MFU of 40% on trillion parameter training runs. As a recap, MFU is a measure of the effective throughput and utilization of the peak potential FLOPS after taking into account overhead and various bottlenecks such as (power limits, communication flakiness, recomputation, stragglers, and inefficient kernels). A 100,000 H100 cluster would only take four days using FP8 to train GPT-4. On a 100k H100 cluster training run for 100 days, you can achieve an effective FP8 Model FLOP of ~6e26 (600 million ExaFLOP). Note that the poor reliability of hardware reduces MFU significantly.
在 H100 上,人工智能实验室在万亿参数训练中实现了高达 35%的 FP8 模型 FLOPs 利用率(MFU)和 40%的 FP16 MFU。回顾一下,MFU 是有效吞吐量和峰值潜在 FLOPS 利用率的衡量标准,考虑了开销和各种瓶颈,如(功率限制、通信不稳定、重新计算、滞后和低效内核)。使用 FP8 训练 GPT-4 的 100,000 H100 集群仅需四天。在一个 100,000 H100 集群的训练运行中,持续 100 天,可以实现约 6e26(600 百万 ExaFLOP)的有效 FP8 模型 FLOP。请注意,硬件的低可靠性显著降低了 MFU。
Power Challenges 电力挑战
The critical IT power required for a 100k H100 cluster is ~150MW. While the GPU itself is only 700W, within each H100 server, CPUs, Network Interface Cards (NICs), Power Supply Units (PSUs), account for a further ~575W per GPU. Other than the H100 servers, an AI cluster requires a collection of storage servers, networking switches, CPU nodes, optical transceivers, and many other items that together account for another ~10% in IT power. Putting into perspective how much power ~150MW is, the largest national lab supercomputing, El Capitan only requires 30MW of critical IT power. Government supercomputers pale in comparison to industry.
一个 100k H100 集群所需的关键 IT 功率约为 150MW。虽然 GPU 本身仅为 700W,但在每个 H100 服务器中,CPU、网络接口卡(NIC)、电源单元(PSU)每个 GPU 还需约 575W。除了 H100 服务器,AI 集群还需要一系列存储服务器、网络交换机、CPU 节点、光收发器以及许多其他设备,这些设备共同占据了约 10%的 IT 功率。将 150MW 的功率放在一个更大的背景下,最大的国家实验室超级计算机 El Capitan 仅需 30MW 的关键 IT 功率。政府超级计算机与行业相比显得微不足道。
One major power challenge is that currently no single datacenter building has the capacity for a new ~150MW deployment. When people refer to 100k GPU clusters, generally they mean on a single campus, not building. The search for power is so dire, X.AI is even converting an old factory in Memphis Tennessee into a datacenter due to the lack of other options.
一个主要的电力挑战是,目前没有单一的数据中心建筑能够容纳新的约 150MW 的部署。当人们提到 10 万 GPU 集群时,通常是指在一个校园内,而不是在一栋建筑内。电力需求如此迫切,X.AI 甚至将田纳西州孟菲斯的一座旧工厂改造成数据中心,因为没有其他选择。
These clusters are networked with optical transceivers, which come on a sliding scale of cost vs reach. Longer range “single mode” DR and FR transceivers which can reliably transmit signals ~500 meters to ~2km but can cost 2.5x as much as “multi-mode” SR and AOC transceivers which only support only up to ~50 meters reach. Furthermore, campus level “coherent” 800G transceivers with over 2km of range also exist albeit at 10x+ higher pricing.
这些集群通过光收发器联网,这些收发器的成本与覆盖范围呈滑动比例。长距离的“单模”DR 和 FR 收发器可以可靠地传输信号,范围约为 500 米到 2 公里,但其成本可能是“多模”SR 和 AOC 收发器的 2.5 倍,而后者仅支持约 50 米的覆盖范围。此外,校园级的“相干”800G 收发器的范围超过 2 公里,尽管其价格高出 10 倍以上。
Small clusters of H100’s generally connect every GPU at 400G to every other GPU with only multi-mode transceivers through just a layer or two of switches. With large clusters of GPUs, more layers of switching must be added, and the optics becomes exorbitantly expensive. The network topology of such a cluster will differ broadly based on preferred vendor, current and future workloads, and capex.
小型 H100 集群通常通过仅一层或两层交换机,使用多模收发器以 400G 的速度将每个 GPU 连接到其他每个 GPU。对于大型 GPU 集群,必须增加更多的交换层,光学设备变得极其昂贵。此类集群的网络拓扑将根据首选供应商、当前和未来的工作负载以及资本支出有很大不同。
Each building will generally contain a pod or multiple pods of compute connected with cheaper copper cables or multi-mode transceivers. They will then use longer range transceivers in order to interconnect between “islands” of compute. The image below shows 4 islands of compute that have high bandwidth within the island but lower bandwidth outside the island. 155MW is challenging to deliver in a single location, but we are tracking over 15 Microsoft, Meta, Google, Amazon, Bytedance, X.AI, Oracle, etc data center build outs that will have that much space for AI servers and networking.
每栋建筑通常会包含一个或多个计算舱,通过更便宜的铜缆或多模收发器连接。然后,它们将使用更长范围的收发器在计算“岛”之间进行互联。下图显示了 4 个计算岛,岛内带宽高,但岛外带宽较低。155MW 在单一地点交付是一个挑战,但我们正在跟踪超过 15 个微软、Meta、谷歌、亚马逊、字节跳动、X.AI、甲骨文等数据中心的建设,这些数据中心将有足够的空间用于 AI 服务器和网络。
Different customers choose different network topologies based on several different factors such as data haul infrastructure, cost, maintainability, power, current, future workloads etc. As such, some customers are choosing Broadcom Tomahawk 5 based switches, others are sticking to Infiniband, while others are choosing NVIDIA Spectrum-X. We will dive into why below.
不同的客户根据多个不同因素选择不同的网络拓扑,例如数据传输基础设施、成本、可维护性、功率、当前和未来的工作负载等。因此,一些客户选择基于 Broadcom Tomahawk 5 的交换机,另一些则坚持使用 Infiniband,而其他客户则选择 NVIDIA Spectrum-X。我们将在下面深入探讨原因。
Parallelism Refresher 平行性复习
To understand network design, topology, reliability concerns, and checkpointing strategies, we will first do a quick refresher on the 3 different types of parallelism used in trillion parameter training - Data Parallelism, Tensor Parallelism, and Pipeline Parallelism. We have a comprehensive explanation of parallelism here, including Expert Parallelism.
要理解网络设计、拓扑结构、可靠性问题和检查点策略,我们首先将快速回顾在万亿参数训练中使用的三种不同类型的并行性——数据并行性、张量并行性和流水线并行性。我们在这里有关于并行性的全面解释,包括专家并行性。
Data Parallelism is the simplest form of parallelism in which each GPU holds the entire copy of the model weights and each GPU (rank) receives a different subset of the data. This type of parallelism has the lowest level of communication since just the gradients needs to be summed up (all reduce) between each GPU. Unfortunately, data parallelism only works if each GPU has enough memory to store the entire model weights, activations, optimizer state. For a 1.8 trillion parameter model like GPT-4, just the model weights and optimizer state can take as much as 10.8 Terabytes of memory for training.
数据并行是最简单的并行形式,其中每个 GPU 持有模型权重的完整副本,每个 GPU(排名)接收不同的数据子集。这种类型的并行通信级别最低,因为只需要在每个 GPU 之间对梯度进行求和(全归约)。不幸的是,数据并行仅在每个 GPU 有足够的内存来存储整个模型权重、激活和优化器状态时才有效。对于像 GPT-4 这样拥有 1.8 万亿参数的模型,仅模型权重和优化器状态在训练时就可能占用多达 10.8 TB 的内存。
In order to overcome these memory constraints, tensor parallelism is used. In tensor parallelism, every layer has its work and model weights distributed across multiple GPUs generally across the hidden dimension. Intermediate work is exchanged via all-reductions across devices multiple times across self-attention, feed forward network, and layer normalizations for each layer. This requires high bandwidth and especially needs very low latency. In effect, every GPU in the domain works together on every layer with every other GPU as if there were all one giant GPU. Tensor parallelism reduces the total memory used per GPU by the number of tensor parallelism ranks. For example, it is common to use 8 tensor parallelism ranks today across NVLink so this will reduce the used memory per GPU by 8.
为了克服这些内存限制,采用了张量并行。在张量并行中,每一层都有其工作和模型权重分布在多个 GPU 上,通常是在隐藏维度上。中间工作通过设备之间的全归约多次交换,涉及自注意力、前馈网络和每层的层归一化。这需要高带宽,特别需要非常低的延迟。实际上,领域内的每个 GPU 在每一层上与其他每个 GPU 协同工作,就好像它们都是一个巨大的 GPU。张量并行通过张量并行等级的数量减少每个 GPU 使用的总内存。例如,今天在 NVLink 上常用 8 个张量并行等级,因此这将使每个 GPU 使用的内存减少 8 倍。
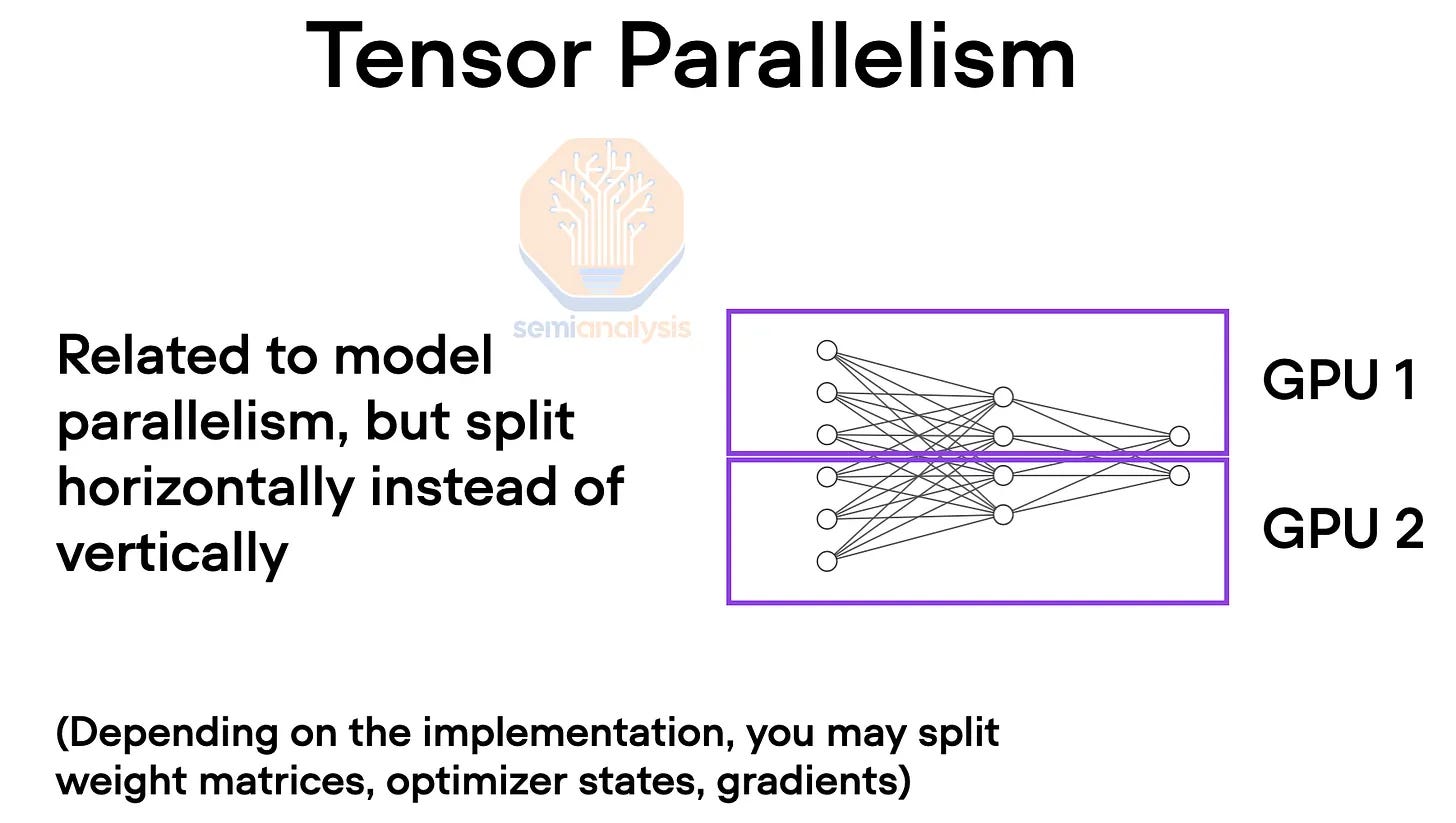
来源:加速 Pytorch 训练
Another technique to overcome the challenges of each GPU not having enough memory to fit the model weights and optimizer state is by using pipeline parallelism. With Pipeline Parallelism, each GPU only has a subset of the layers and only does the computation for that layer and passes the output other the next GPU. This technique reduces the amount of memory needed by the number of pipeline parallelism ranks. Pipeline parallelism has heavy communication volume requirements, but not as heavy as tensor parallelism.
另一种克服每个 GPU 内存不足以容纳模型权重和优化器状态的挑战的技术是使用管道并行。通过管道并行,每个 GPU 仅拥有一部分层,并且只对该层进行计算,然后将输出传递给下一个 GPU。这种技术通过管道并行的等级数量减少了所需的内存量。管道并行对通信量的要求很高,但不如张量并行那么高。
In order to maximize Model FLOP Utilization (MFU), companies generally combine all three forms of parallelism to form 3D Parallelism. Then they apply Tensor Parallelism to GPUs within the H100 server, then use Pipeline Parallelism between nodes within the same Island. Since Data Parallelism has the lowest communication volume and the networking between islands is slower, data parallelism is used between islands.
为了最大化模型浮点运算利用率(MFU),公司通常将三种并行形式结合形成 3D 并行性。然后,他们在 H100 服务器的 GPU 上应用张量并行性,再在同一岛屿内的节点之间使用流水线并行性。由于数据并行性具有最低的通信量,而岛屿之间的网络速度较慢,因此在岛屿之间使用数据并行性。
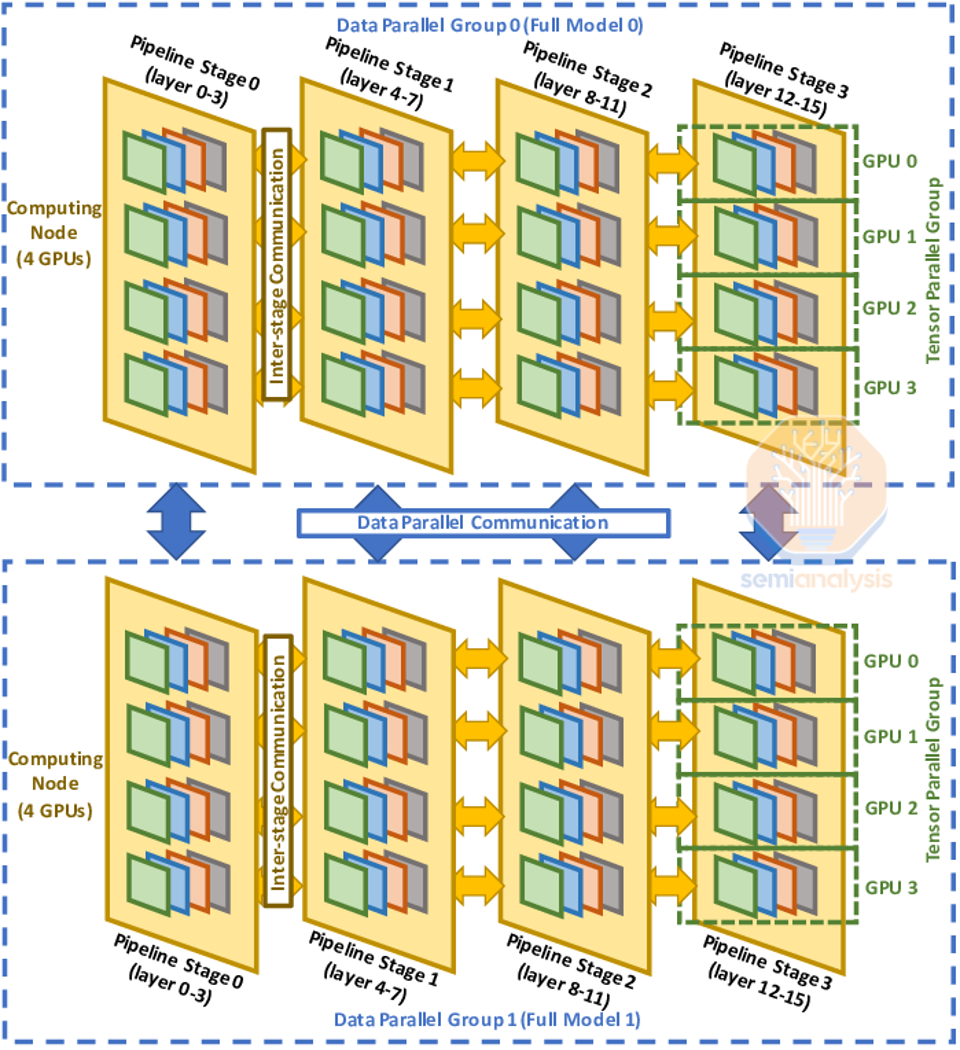
Whole techniques like FSDP are common at small GPU world sizes for very large models, it doesn't work. It's effectively incompatible with pipeline parallelism.
像 FSDP 这样的整体技术在小 GPU 世界规模下对于非常大的模型是常见的,但它并不奏效。它与流水线并行性实际上是不兼容的。
Network Design Considerations
网络设计考虑因素
Networks are designed with parallelism schemes in mind. If every GPU connected to every other GPU at max bandwidth in a fat tree topology, then costs would be exorbitant as 4 layers of switching would be required. The cost of optics would soar as every additional layer of networking requires optics in between.
网络的设计考虑了并行方案。如果每个 GPU 以最大带宽连接到每个其他 GPU,采用肥树拓扑结构,那么成本将会非常高,因为需要 4 层交换。光学的成本将飙升,因为每增加一层网络就需要在中间增加光学设备。
As such, noone deploys full fat tree architectures for large GPU clusters. Instead, they rely on making islands of compute that have full fat tree architectures alongside lesser bandwidth between these islands. There are a variety of ways to do this, but most firms are choosing to “oversubscribe” the top layer of networking. For example see Meta’s last generation architecture for GPU clusters up to 32,000. There are 8 total islands with full fat bandwidth between them, then another layer of switching on top that has 7:1 over subscription. The networking between islands is 7x slower compared to the networking within the island.
因此,没有人会为大型 GPU 集群部署全脂树架构。相反,他们依赖于创建计算岛,这些岛具有全脂树架构,而这些岛之间的带宽较低。有多种方法可以做到这一点,但大多数公司选择对网络的顶层进行“超额订阅”。例如,参见 Meta 的上一代 GPU 集群架构,支持高达 32,000 个节点。总共有 8 个岛屿,岛屿之间具有全脂带宽,然后在其上方还有一层交换,具有 7:1 的超额订阅。岛屿之间的网络速度比岛屿内部的网络慢 7 倍。
GPU deployments have multiple networks, frontend, backend, and scale up (NVLink). In some cases, you will run different parallelism schemes across each. The NVLink network may be the only one fast enough for the bandwidth requirements of tensor parallelism. Your backend can generally handle most other types of parallelism easily, but if there is oversubscription, one often is relegated to data parallelism only.
GPU 部署有多个网络,包括前端、后端和扩展 (NVLink)。在某些情况下,您将在每个网络上运行不同的并行方案。NVLink 网络可能是唯一一个足够快以满足张量并行性带宽要求的网络。您的后端通常可以轻松处理大多数其他类型的并行性,但如果出现超额订阅,通常只能使用数据并行性。
Furthermore, some folks do not even have islands with bandwidth oversubscribed at the top layer. Instead, they move out of the backend network into frontend networking for island to island communications.
此外,一些人甚至没有在顶层带宽超额分配的岛屿。相反,他们从后端网络转移到前端网络进行岛屿之间的通信。
Hybrid InfiniBand and Frontend Ethernet Fabric
混合型 InfiniBand 和前端以太网架构
One major firm trains across multiple InfiniBand islands with frontend Ethernet. This is because the cost for frontend networking is much cheaper and can utilize existing datacenter campus networking between buildings and region routing.
一家大型公司在多个 InfiniBand 岛屿之间使用前端以太网进行训练。这是因为前端网络的成本要便宜得多,并且可以利用现有的数据中心校园网络在建筑物之间和区域路由。
Unfortunately, as model size grow faster due to sparse techniques like MoE, the amount of communication volume that the frontend network needs to handle grows too. This tradeoff must be carefully optimized or else you will end up having two networks that cost the same, as the frontend networking bandwidth will ending up growing so large that it may match the backend networking bandwidth.
不幸的是,随着模型规模因稀疏技术(如 MoE)而快速增长,前端网络需要处理的通信量也在增加。这个权衡必须经过仔细优化,否则你将最终拥有两个成本相同的网络,因为前端网络带宽将增长到可能与后端网络带宽相匹配的程度。
It should be noted that Google exclusively uses front end networking for their multi-TPU pod training runs. Their “compute fabric” known as ICI only scales up to a maximum of 8960 chips with costly 800G optics and optical circuit switches connecting each 64 TPU watercooled rack. As such Google must compensate by making the TPU front end network beefier than most GPU front end networks.
需要注意的是,谷歌在其多 TPU 集群训练运行中专门使用前端网络。他们的“计算架构”称为 ICI,最多只能扩展到 8960 个芯片,使用昂贵的 800G 光学设备和光电路交换机连接每个 64 个 TPU 水冷机架。因此,谷歌必须通过使 TPU 前端网络比大多数 GPU 前端网络更强大来进行补偿。
When frontend networks are utilized during training, network topology aware global all-reduce between islands must be done. First each pod or island will perform a local reduce-scatter within the pod InfiniBand or ICI networking, this will leave each GPU/TPU having the sum for a subsection of the gradient. Next, a cross pod all-reduce between each host rank will be performed using a frontend ethernet networking, then finally each pod would perform a pod-level all-gather.
在训练过程中使用前端网络时,必须在各个岛屿之间进行网络拓扑感知的全局全规约。首先,每个 pod 或岛屿将在 pod 的 InfiniBand 或 ICI 网络内执行本地规约-散布,这将使每个 GPU/TPU 拥有梯度子部分的总和。接下来,将使用前端以太网网络在每个主机排名之间执行跨 pod 全规约,最后每个 pod 将执行 pod 级别的全收集。
The frontend networking is also responsible for loading in data. As we move towards multimodal image and video training data, front-end networking requirements will increase exponentially. In such a situation, the front-end networking bandwidth will be fighting between loading large video files and doing all reductions. Furthermore, your straggler problem increases as if there is irregular storage network traffic, it would cause your entire all-reduces to slow down and not be predictively modeled.
前端网络还负责加载数据。随着我们向多模态图像和视频训练数据的转变,前端网络的需求将呈指数级增长。在这种情况下,前端网络带宽将面临加载大型视频文件和进行所有归约之间的竞争。此外,您的滞后问题会增加,因为如果存储网络流量不规则,它会导致您整个的所有归约变慢,并且无法进行预测建模。
The alternative would be a 4 tier InfiniBand network with a 7:1 oversubscription with 4 pods with each pod having 24,576 H100s with a non-blocking 3 tier system. This allows for much greater flexibility for future bandwidth increases compared to using your frontend networking as it is way easier to add more fiber optics transceivers from a switch in building A to another switch in building B compared to doing a full frontend network NIC upgrade in every single chassis of the cluster to upgrade it from 100G to 200G, etc.
替代方案是一个 4 层 InfiniBand 网络,具有 7:1 的超额订阅,包含 4 个舱,每个舱有 24,576 个 H100,采用非阻塞的 3 层系统。这相比于使用前端网络,允许未来带宽增加时具有更大的灵活性,因为从 A 楼的交换机到 B 楼的另一个交换机添加更多光纤收发器要容易得多,而不是在集群的每个机箱中进行全面的前端网络 NIC 升级,以将其从 100G 升级到 200G 等。
This creates a more stable network pattern as your frontend network can solely focus on loading data and checkpointing, and your backend network can solely focus on GPU to GPU communication. This also helps with the stragglers problem. But unfortunately, a 4 tier Infiniband network is extremely expensive due to all the additional switches and transceivers needed.
这创建了一个更稳定的网络模式,因为您的前端网络可以专注于加载数据和检查点,而您的后端网络可以专注于 GPU 到 GPU 的通信。这也有助于解决滞后问题。但不幸的是,四层 Infiniband 网络由于需要所有额外的交换机和收发器而极其昂贵。
Rail Optimized vs Middle of Rack
轨道优化与机架中间
To improve maintainability and increase the use of copper networking (< 3 meters) and multimode networking (< 50 meters), some customers are opting to ditch the NVIDIA recommended rail optimized design and instead of opting to do a Middle of Rack designs.
为了提高可维护性并增加铜网络(< 3 米)和多模网络(< 50 米)的使用,一些客户选择放弃 NVIDIA 推荐的轨道优化设计,而选择中间机架设计。
Rail optimized is a technique to have each H100 server connect to 8 different leaf switches (instead of all connecting to the same middle of rack switch) such that each GPU can talk to more distant GPUs with only 1 switch hop. This allows an increase in real world all to all collective performance increase. All-to-All collective communication is used heavily in mixture of experts (MoE) expert parallelism.
铁路优化是一种技术,使每个 H100 服务器连接到 8 个不同的叶子交换机(而不是全部连接到同一个机架中间交换机),这样每个 GPU 可以仅通过 1 个交换机跳跃与更远的 GPU 进行通信。这允许在实际的全到全集合性能上实现提升。全到全集合通信在专家混合(MoE)专家并行中被广泛使用。
The downside of rail optimized designs is that you must connect to different leaf switches of varying distance as opposed to one middle of rack switch in close proximity to all 8 GPUs in the server. When the switch can be placed in the same rack, Passive Direct Attached Cable (DAC) and Active Electrical Cables (AEC) can be used, but in rail optimized designs where switches are not necessarily in the same rack, optics must be used. Moreover, the leaf to spine distance may be greater than the 50-meter distance, forcing the use of single-mode optical transceivers.
轨道优化设计的缺点是必须连接到不同的叶子交换机,这些交换机的距离各不相同,而不是连接到靠近服务器中所有 8 个 GPU 的一个中间机架交换机。当交换机可以放置在同一个机架时,可以使用被动直连电缆(DAC)和主动电缆(AEC),但在轨道优化设计中,交换机不一定在同一个机架时,必须使用光纤。此外,叶子到脊的距离可能大于 50 米,这迫使使用单模光收发器。
By using a non rail-optimized design, you can replace 98,304 optical transceivers connecting GPUs to leaf switches with cheap direct attached copper, leading to 25-33% of your GPU fabric being copper. As you can see from the below rack diagram, instead of each GPU to leaf switch connection going up to the cable tray then sideways 9 racks to a dedicated rail optimized leaf switch rack, the leaf switches are now in the middle of the rack allowing for each GPU to use DAC copper cables.
通过使用非轨道优化设计,您可以用便宜的直连铜缆替换连接 GPU 到叶子交换机的 98,304 个光收发器,从而使您的 GPU 结构中有 25-33%是铜缆。正如下面的机架图所示,GPU 到叶子交换机的每个连接不再需要上升到电缆托盘,然后横向穿过 9 个机架到专用的轨道优化叶子交换机机架,而是将叶子交换机放置在机架的中间,使每个 GPU 都可以使用 DAC 铜缆。
DAC copper cables run cooler, use less power, and are much cheaper compared to optics. Since DAC cables run coolers, use less power, and are more reliable, this leads to less flapping (a network link going down intermittently) and failures, which is a major problem for all high-speed interconnects using optics. A Quantum-2 IB spine switch uses 747 Watts when using DAC copper cables. When using multimode optical transceivers, power consumption increases to up to 1,500 Watts.
DAC 铜缆运行更凉爽,使用更少的电力,并且相比光纤更便宜。由于 DAC 电缆运行更凉爽,使用更少的电力,并且更可靠,这导致了更少的波动(网络链接间歇性中断)和故障,这对所有使用光纤的高速互连来说是一个主要问题。Quantum-2 IB 脊交换机在使用 DAC 铜缆时消耗 747 瓦特。当使用多模光收发器时,功耗增加到高达 1,500 瓦特。
Furthermore, initial cabling for a rail optimized design is extremely time consuming for datacenter technicians since the ends of each link are up to 50 meters away and not on the same rack. Compared to a middle of rack design where you have your leaf switch in the same rack as all of your GPUs that connect to the leaf switch. In a middle of rack design, you can even test the compute node to leaf switch links at the integration factory since it is all within the same rack.
此外,针对铁路优化设计的初始布线对数据中心技术人员来说非常耗时,因为每个链路的两端相距可达 50 米,并且不在同一机架上。与中间机架设计相比,后者的叶子交换机与所有连接到叶子交换机的 GPU 位于同一机架。在中间机架设计中,您甚至可以在集成工厂测试计算节点与叶子交换机之间的链路,因为它们都在同一机架内。
Reliability and Recovery 可靠性与恢复
Reliability is one of the most important operational concerns with these giant clusters due to the synchronous nature of current frontier training techniques. The most common reliability problems are GPU HBM ECC error, GPUs Drivers being stuck, optical transceivers failing, NICs overheating, etc. Nodes are constantly going down or spitting out errors.
可靠性是这些大型集群最重要的操作问题之一,因为当前前沿训练技术的同步特性。最常见的可靠性问题包括 GPU HBM ECC 错误、GPU 驱动程序卡住、光收发器故障、网络接口卡过热等。节点不断出现故障或输出错误。
To keep the mean time for fault recovery low and training continuing, datacenters must keep hot spare nodes and cold spare components on site. When a failure happens, instead of stopping the whole training run, it is best to swap in a working spare node that is already on, and continuing training. A lot of the downtime for these servers is simply power cycling/restarting the node and that fixing whatever issue arose.
为了保持故障恢复的平均时间较低并持续进行训练,数据中心必须在现场保留热备份节点和冷备份组件。当发生故障时,最好是更换一个已经开启的工作备用节点,而不是停止整个训练过程,继续进行训练。这些服务器的大部分停机时间仅仅是电源循环/重启节点以及修复出现的任何问题。
A simple restart doesn’t fix every problem though, and in many cases it requires a datacenter technician to physically diagnosis and replace equipment. In the best case situation, it will take multiple hours for a datacenter technician to fix a broken GPU server, but in many cases it can take days before a broken node can be brought back into to the training run. Broken nodes and spare hot nodes are GPUs that are not actively contributing to the model, despite theoretically having FLOPS to deliver.
简单的重启并不能解决所有问题,在许多情况下,需要数据中心技术人员进行物理诊断和更换设备。在最佳情况下,数据中心技术人员修复一台损坏的 GPU 服务器需要几个小时,但在许多情况下,损坏的节点可能需要几天才能重新投入训练。损坏的节点和备用热节点是指那些尽管理论上有 FLOPS 可供使用,但并未积极参与模型计算的 GPU。
As a model is trained, frequent checkpointing of the model to CPU memory or NAND SSDs is needed in case errors such as HBM ECC happen. When an error occurs, you must reload the weights of the model and the optimizer from the slower tier of memory and restart training. Fault tolerance training techniques, such as Oobleck, can be used to provide user level application driven approaches to deal with GPU and network failures.
随着模型的训练,需要频繁将模型检查点保存到 CPU 内存或 NAND SSD,以防发生如 HBM ECC 这样的错误。当发生错误时,必须从较慢的内存层重新加载模型和优化器的权重,并重新开始训练。容错训练技术,如 Oobleck,可以用于提供用户级应用驱动的方法来应对 GPU 和网络故障。
Unfortunately, frequent checkpointing and fault tolerance training techniques hurt the overall MFU of the system. The cluster needs to constantly pause to save its current weights to persistent memory or CPU memory. Furthermore, when you reload from checkpoints, you usually only save once every 100 iterations. This means you can only losing at max 99 steps of useful work. On an 100k cluster, if each iteration took 2 seconds, you lose up to 229 GPU days of work from a failure at iteration 99.
不幸的是,频繁的检查点和容错训练技术损害了系统的整体 MFU。集群需要不断暂停以将当前权重保存到持久内存或 CPU 内存。此外,当您从检查点重新加载时,通常每 100 次迭代只保存一次。这意味着您最多只能损失 99 步有用的工作。在一个 10 万的集群中,如果每次迭代耗时 2 秒,您将在第 99 次迭代时因故障损失多达 229 个 GPU 天的工作。
The other approach to fault recovery is to have your spare nodes just RDMA copy from other GPUs over the backend fabric. Since the backend GPU fabric is approximately 400Gbps and there are 80GB of HBM memory per GPU, it will take approximately ~1.6 seconds to copy the weights. With this approach, at max you will only lose 1 step (since more GPU HBM will have the most recent copy of the weights), so that would be only 2.3 GPU days’ worth of computation + another 1.85 GPU days to RDMA copy the weights from other GPUs HBM memory.
另一种故障恢复的方法是让备用节点通过后端网络从其他 GPU 进行 RDMA 复制。由于后端 GPU 网络的带宽约为 400Gbps,每个 GPU 有 80GB 的 HBM 内存,因此复制权重大约需要 1.6 秒。采用这种方法,最多只会损失 1 步(因为更多的 GPU HBM 将拥有最新的权重副本),因此这将仅相当于 2.3 个 GPU 天的计算 + 另加 1.85 个 GPU 天用于从其他 GPU 的 HBM 内存中进行 RDMA 复制权重。
Most leading AI labs have implemented this, but many smaller firms still stick to using the heavy, slow, inefficient technique of restarting from checkpoints for all failures due to simplicity. Implementing fault recovery through memory reconstruction can add multiple percentage points to MFU for a large training run.
大多数领先的人工智能实验室已经实施了这一点,但许多较小的公司仍然坚持使用简单的从检查点重启的繁重、缓慢、低效的技术来应对所有故障。通过内存重建实现故障恢复可以为大规模训练运行增加多个百分点的 MFU。
One of the most common problems encountered is Infiniband/RoCE link failure. Even if each NIC to leaf switch link had a mean time to failure rate of 5 years, due to the high number of transceivers, it would only take 26.28 minutes for the first job failure on a brand new, working cluster. Without fault recovery through memory reconstruction, more time would be spent restarting the training run in 100,000 GPU clusters due to optics failures, than it would be advancing the model forward.
最常见的问题之一是 Infiniband/RoCE 链接故障。即使每个 NIC 到叶子交换机的链接平均故障时间为 5 年,由于收发器数量众多,在一个全新且正常工作的集群中,第一次作业故障只需 26.28 分钟。没有通过内存重建进行故障恢复,因光学故障而在 100,000 个 GPU 集群中重新启动训练运行所花费的时间,将比推进模型所需的时间更长。
Since each GPU is directly attached to a ConnectX-7 NIC (through a PCIe switch), there is no fault tolerance at the network architecture level thus the fault must be handled at the user training code, directly adding to complexity of the codebase. This is one of the main challenges of current GPU network fabrics from NVIDIA and AMD, where even if one NIC fails, that GPU then has no alternative paths to talk to other GPUs. Due to the way current LLMs use tensor parallelism within the node, if even one NIC, one transceiver or one GPU fails, the whole server is considered down.
由于每个 GPU 直接连接到 ConnectX-7 网卡(通过 PCIe 交换机),因此在网络架构层面没有容错机制,因此故障必须在用户训练代码中处理,直接增加了代码库的复杂性。这是当前 NVIDIA 和 AMD 的 GPU 网络结构面临的主要挑战之一,即使一个网卡发生故障,该 GPU 也没有其他路径与其他 GPU 通信。由于当前LLMs在节点内使用张量并行的方式,如果即使一个网卡、一个收发器或一个 GPU 发生故障,整个服务器将被视为宕机。
There is a lot of work being done to make networks reconfigurable and not have nodes be so fragile. This work is critical as the status quo means an entire GB200 NVL72 would go down with just 1 GPU failure or 1 optical failure. A multi-million-dollar 72 GPU rack going down is far more catastrophic than an 8 GPU server worth a few hundred thousand dollars.
正在进行大量工作,以使网络可重新配置,并且节点不那么脆弱。这项工作至关重要,因为现状意味着整个 GB200 NVL72 只需 1 个 GPU 故障或 1 个光学故障就会崩溃。一个价值数百万美元的 72 GPU 机架的故障远比一个价值几十万美元的 8 GPU 服务器的故障更具灾难性。
Nvidia has noticed this major problem and added a dedicated engine for reliability, availability and serviceability (RAS). We believe RAS engine analyzes chip level data such temperature, number of recovered ECC retries, clocks speed, voltages to predict when the chip will likely failure and alert the datacenter technician. This will allow them to do proactive maintenance such as using a higher fan speed profile to maintain reliability, take the server out of service for furthermore physical inspection at a later maintenance window. Furthermore, before starting a training job, each chip’s RAS engine will perform a comprehensive self-check such as running matrix multiplication with known results to detect silent data corruptions (SDC).
英伟达注意到了这个重大问题,并增加了一个专用的可靠性、可用性和可维护性(RAS)引擎。我们相信,RAS 引擎分析芯片级数据,如温度、恢复的 ECC 重试次数、时钟速度、电压,以预测芯片何时可能发生故障并提醒数据中心技术人员。这将使他们能够进行主动维护,例如使用更高的风扇速度配置来保持可靠性,在后续的维护窗口中将服务器下线进行进一步的物理检查。此外,在开始训练作业之前,每个芯片的 RAS 引擎将执行全面的自检,例如运行已知结果的矩阵乘法,以检测静默数据损坏(SDC)。
Cedar-7 雪松-7
Another cost optimization that some customers like Microsoft/Openai are doing is by using the Cedar Fever-7 networking module per server instead of using 8 PCIe form factor ConnectX-7 networking cards. One of the main benefits of using a Cedar Fever module is that it allows for just using 4 OSFP cages instead of 8 OSFP cages thus allowing for the use of twin port 2x400G transceivers on the compute node end too, instead of just the switch end. This reduces the transceiver count to connect to the leaf switches from 8 transceivers to 4 transceivers per H100 node. The total compute node end transceiver counts to connect GPUs to leaf switch reduces from 98,304 to 49,152.
另一个一些客户如微软/Openai 正在进行的成本优化是每台服务器使用 Cedar Fever-7 网络模块,而不是使用 8 个 PCIe 形式因素的 ConnectX-7 网络卡。使用 Cedar Fever 模块的主要好处之一是它只需要使用 4 个 OSFP 机箱,而不是 8 个 OSFP 机箱,从而允许在计算节点端使用双端口 2x400G 收发器,而不仅仅是在交换机端。这将连接到叶子交换机的收发器数量从每个 H100 节点的 8 个收发器减少到 4 个收发器。连接 GPU 到叶子交换机的计算节点端收发器总数从 98,304 减少到 49,152。
Since the GPU to leaf switch links are cut in half, this also helps with the estimated time to first job failure. We estimate that each twin port 2x400G link has a mean time to failure per link of 4 years (vs. 5 years of single port 400G link) and this will bring the estimated time to first job failure to 42.05 minutes, this is way better than the 26.28 minutes without Cedar-7 modules.
由于 GPU 到叶子交换机的链接被减半,这也有助于估算首次作业失败的时间。我们估计每个双端口 2x400G 链接的平均故障时间为 4 年(相比单端口 400G 链接的 5 年),这将使首次作业失败的估算时间缩短至 42.05 分钟,这比没有 Cedar-7 模块的 26.28 分钟要好得多。
Spectrum-X NVIDIA 光谱-X NVIDIA
There is currently a 100k H100 cluster being deployed and will be operational by end of the year that is using NVIDIA Spectrum-X Ethernet.
目前正在部署一个 100k H100 集群,预计将在年底前投入使用,该集群使用 NVIDIA Spectrum-X 以太网。
Last year we covered a variety of advantages Spectrum-X has over InfiniBand in large networks. Even outside the performance and reliability advantages, Spectrum-X also has a huge cost advantage. Spectrum-X Ethernet is that each SN5600 switch has 128 ports of 400G while the InfiniBand NDR Quantum-2 switch only has 64 ports of 400G. Note that Broadcom’s Tomahawk 5 switch ASIC also supports 128 400G ports, putting the current generation of InfiniBand at a big disadvantage.
去年我们讨论了 Spectrum-X 在大型网络中相对于 InfiniBand 的多种优势。即使在性能和可靠性优势之外,Spectrum-X 也具有巨大的成本优势。Spectrum-X 以太网的每个 SN5600 交换机有 128 个 400G 端口,而 InfiniBand NDR Quantum-2 交换机只有 64 个 400G 端口。请注意,博通的 Tomahawk 5 交换机 ASIC 也支持 128 个 400G 端口,这使得当前一代 InfiniBand 处于很大的劣势。
A fully interconnected 100k cluster can be 3 tiers instead of 4 tiers. Having 4 tiers instead of 3 tiers means that there are 1.33x more transceivers required. Due to the lower radix of the Quantum-2 switch, the maximum number of fully interconnected GPUs on a 100k cluster is limited to 65,536 H100s. The next generation of InfiniBand switches called Quantum-X800 solves this by having 144 800G ports, though as one can tell from the number “144”, this is designed for use with the NVL72 and NVL36 systems and is not expected to be used much in B200 or B100 clusters. Even though there is cost savings of not having to do 4 tiers with Spectrum-X, the unfortunate downside is that you still need to buy highly marked up transceivers from Nvidia LinkX product line as other transceivers may not work or be validated by Nvidia.
一个完全互联的 10 万集群可以是 3 层而不是 4 层。拥有 4 层而不是 3 层意味着需要 1.33 倍的收发器。由于 Quantum-2 交换机的较低基数,10 万集群上完全互联的 GPU 数量限制为 65,536 个 H100。下一代 InfiniBand 交换机 Quantum-X800 通过拥有 144 个 800G 端口解决了这个问题,尽管从数字“144”可以看出,这个设计是用于 NVL72 和 NVL36 系统,并不预计在 B200 或 B100 集群中广泛使用。尽管使用 Spectrum-X 不需要进行 4 层可以节省成本,但不幸的是,您仍然需要从 Nvidia LinkX 产品线购买高价收发器,因为其他收发器可能无法正常工作或未经过 Nvidia 验证。
The main advantage of Spectrum-X over other vendors is that Spectrum-X is supported first class by NVIDIA libraries such as NCCL and Jensen pushes you up the allocation queue to being one of the first customers for their new product line vs. with the Tomahawk 5 chips, you need a lot of in house engineering effort to optimize your network with NCCL in order to achieve max throughput.
Spectrum-X 相较于其他供应商的主要优势在于,Spectrum-X 得到了 NVIDIA 库(如 NCCL)的一级支持,而 Jensen 会将您推到分配队列的前列,使您成为其新产品线的首批客户。相比之下,使用 Tomahawk 5 芯片时,您需要大量内部工程工作来优化您的网络与 NCCL,以实现最大吞吐量。
An unfortunate downside of using Ethernet instead of InfiniBand for your GPU fabric is that Ethernet does not currently support SHARP in network reductions. In-network reductions work by performing the summation of each GPU by having the network switch run those computations. The theoretical network bandwidth increase of SHARP is 2x as it reduces the number of sends and writes each GPU has to do by 2x.
使用以太网而不是 InfiniBand 作为 GPU 网络的一个不幸缺点是,以太网目前不支持网络归约中的 SHARP。网络归约通过让网络交换机执行这些计算来对每个 GPU 进行求和。SHARP 的理论网络带宽增加为 2 倍,因为它将每个 GPU 需要进行的发送和写入次数减少了 2 倍。
Another downside of Spectrum-X is that for the first generation of 400G Spectrum-X, Nvidia uses Bluefield3 instead of ConnectX-7 as a bandaid solution. For the future generations, we expect ConnectX-8 to work perfectly fine with 800G Spectrum-X though. The price delta between a Bluefield-3 and a ConnectX-7 card is around $300 ASP for hyperscaler volumes, with the other downside being that card uses 50 watts more compared to ConnectX-7. Thus, for each node, 400W of additional power is needed, lowering the “intelligence per picojoule” of the overall training server. The datacenter that you put Spectrum X in now requires an additional 5MW for a 100,000 GPU deployment versus a Broadcom Tomahawk 5 deployment with the exact same network architecture.
Spectrum-X 的另一个缺点是,对于第一代 400G Spectrum-X,Nvidia 使用 Bluefield3 作为临时解决方案,而不是 ConnectX-7。对于未来的几代产品,我们预计 ConnectX-8 将与 800G Spectrum-X 完美兼容。Bluefield-3 和 ConnectX-7 卡之间的价格差异约为每个超大规模客户 300 美元的平均销售价格,另一个缺点是该卡比 ConnectX-7 多消耗 50 瓦特。因此,每个节点需要额外的 400W 电力,降低了整体训练服务器的“每皮焦耳智能”。您在其中放置 Spectrum X 的数据中心现在需要额外的 5MW 电力,以支持 10 万个 GPU 的部署,而与具有完全相同网络架构的 Broadcom Tomahawk 5 部署相比。
Broadcom Tomahawk 5 博通汤姆霍克 5
In order to avoid paying the massive Nvidia tax, a lot of customers are deploying with Broadcom Tomahawk 5 based switches. Each Tomahawk 5 based switch has the same number of ports as the Spectrum-X SN5600 switch at 128 400G ports and achieves similar performance if your firm has good network engineers. Furthermore, you can buy any generic transceivers and copper cables from any vendor in the world and mix and match.
为了避免支付巨额的 Nvidia 税,许多客户正在部署基于 Broadcom Tomahawk 5 的交换机。每个基于 Tomahawk 5 的交换机与 Spectrum-X SN5600 交换机具有相同数量的端口,均为 128 个 400G 端口,并且如果您的公司有优秀的网络工程师,性能相似。此外,您可以从全球任何供应商购买任何通用的收发器和铜缆,并进行混合搭配。
Most customers are partnering directly with ODMs such as Celestica for switches using Broadcom based switch ASICs and firms like Innolight and Eoptolink for transceivers. Based on the switch cost and the generic transceiver cost, Tomahawk 5 is way cheaper compared to Nvidia InfiniBand and is also cheaper when compared to Nvidia Spectrum-X.
大多数客户直接与 ODM 合作,例如 Celestica,使用基于 Broadcom 的交换机 ASIC,以及像 Innolight 和 Eoptolink 这样的公司用于收发器。根据交换机成本和通用收发器成本,Tomahawk 5 相比于 Nvidia InfiniBand 便宜得多,与 Nvidia Spectrum-X 相比也更便宜。
The unfortunate downside of this is that you need to have enough engineering capacity to patch and optimize NCCL communication collectives for the Tomahawk 5. Out of the box, NCCL communication collectives is only optimized for Nvidia Spectrum-X and Nvidia InfiniBand. The good news is that if you have 4 billion dollars for a 100k cluster, you have enough engineering capacity to patch NCCL and write the optimizations. Of course software is hard, and Nvidia is always on the bleeding edge, but generally we expect every hyperscaler to make these optimizations and switch away from InfiniBand.
不幸的是,这意味着您需要有足够的工程能力来修补和优化 Tomahawk 5 的 NCCL 通信集合。开箱即用的 NCCL 通信集合仅针对 Nvidia Spectrum-X 和 Nvidia InfiniBand 进行了优化。好消息是,如果您有 40 亿美元用于 10 万个集群,您就有足够的工程能力来修补 NCCL 并编写优化。当然,软件开发是困难的,Nvidia 始终处于前沿,但一般来说,我们预计每个超大规模云服务商都会进行这些优化并转向其他技术,而不是继续使用 InfiniBand。
We will now talk through the bill of materials for 4 different 100k GPU cluster network designs, the switch and transceiver costs associated with them showing the advantages of different network designs, and a physical floorplan of a GPU cluster optimized for reduced optics.
我们现在将讨论四种不同的 10 万 GPU 集群网络设计的物料清单,相关的交换机和收发器成本,展示不同网络设计的优势,以及一个针对减少光学优化的 GPU 集群的物理平面图。
Bill of Materials 物料清单
The total is approximately $4B of capex per 100k H100 Cluster, but it varies based on the type of networking chosen. We present 4 different options.
总计约为每 10 万个 H100 集群 4 亿美元的资本支出,但根据选择的网络类型有所不同。我们提供 4 种不同的选项。
4 tier InfiniBand network with 32,768 GPU islands, rail optimized, 7:1 oversubscription
四层 InfiniBand 网络,拥有 32,768 个 GPU 岛,铁路优化,7:1 超额订阅3 tier Spectrum X network with 32,768 GPU islands, rail optimized, 7:1 oversubscription
三层光谱 X 网络,拥有 32,768 个 GPU 岛,铁路优化,7:1 超额订阅3 tier InfiniBand network with 24,576 GPU islands, non-rail optimized, front end network for inter-pod
三层 InfiniBand 网络,拥有 24,576 个 GPU 岛屿,非轨道优化,前端网络用于跨 Pod 连接3 tier Broadcom Tomahawk 5 Ethernet network with 32,768 GPU islands, rail optimized, 7:1 oversubscription
三层 Broadcom Tomahawk 5 以太网网络,拥有 32,768 个 GPU 岛,铁路优化,7:1 超额订阅
Comparing between all four different types of network topologies that various firms are building, the 4 tier InfiniBand network is 1.3-1.6x more expensive compared to the other options. This is why no one is actually going with large InfiniBand networks going forward. The handicap option #3 is possible with InfiniBand, but it severely reduces the flexibility in parallelism schemes.
比较各家公司正在构建的四种不同类型的网络拓扑,4 层 InfiniBand 网络的成本比其他选项高出 1.3-1.6 倍。这就是为什么未来没有人会选择大型 InfiniBand 网络。选项#3 的残疾方案在 InfiniBand 上是可行的,但它严重降低了并行方案的灵活性。
Spectrum X provides bigger islands, more bandwidth between islands, and similar cost, but it also comes with the huge penalty of requiring far more power versus the best option.
Spectrum X 提供更大的岛屿、岛屿之间更大的带宽,以及类似的成本,但它也带来了一个巨大的惩罚,即相比最佳选项需要更多的电力。
We believe Broadcom Tomahawk 5 based 32k Island with 7:1 oversubscription at the top layer is the most cost effective option which is why multiple firms have similar networks being built. It offers highest network performance per total cost of ownership (TCO) due to not having to pay the NVIDIA tax, and it has been shipping into the market to major firms like Meta longer than Spectrum X has been available.
我们相信基于 Broadcom Tomahawk 5 的 32k Island 在顶层具有 7:1 超额订阅是最具成本效益的选择,这也是为什么多家公司正在建设类似网络的原因。由于不需要支付 NVIDIA 税,它在总拥有成本(TCO)方面提供了最高的网络性能,并且它已经向 Meta 等主要公司发货的时间比 Spectrum X 上市的时间更长。
Floor Plan 平面图
It is important to optimize your rack layout and floor plan such that you can use as much copper and multimode fiber optics. Below we have a floor plan for a 32k island using rail optimized Spectrum-X / Tomahawk 5. As you can see, for some rows, the leaf switches are not in the same row but instead in another row. This is to optimize for using 50m multimode fiber as if you place the multimode transceivers at the end of the row, the spine switches in the middle will clearly not be within distance.
优化机架布局和楼层计划非常重要,以便尽可能多地使用铜缆和多模光纤。下面是一个使用轨道优化的 Spectrum-X / Tomahawk 5 的 32k 岛屿的楼层平面图。如您所见,对于某些行,叶子交换机不在同一行,而是在另一行。这是为了优化使用 50 米多模光纤,因为如果将多模收发器放置在行的末端,中间的脊交换机显然将不在距离范围内。
The above shows the 4 node rack design. This can fit into Microsoft’s standard datacenter physical design.
上述显示了 4 节点机架设计。这可以适应微软的标准数据中心物理设计。
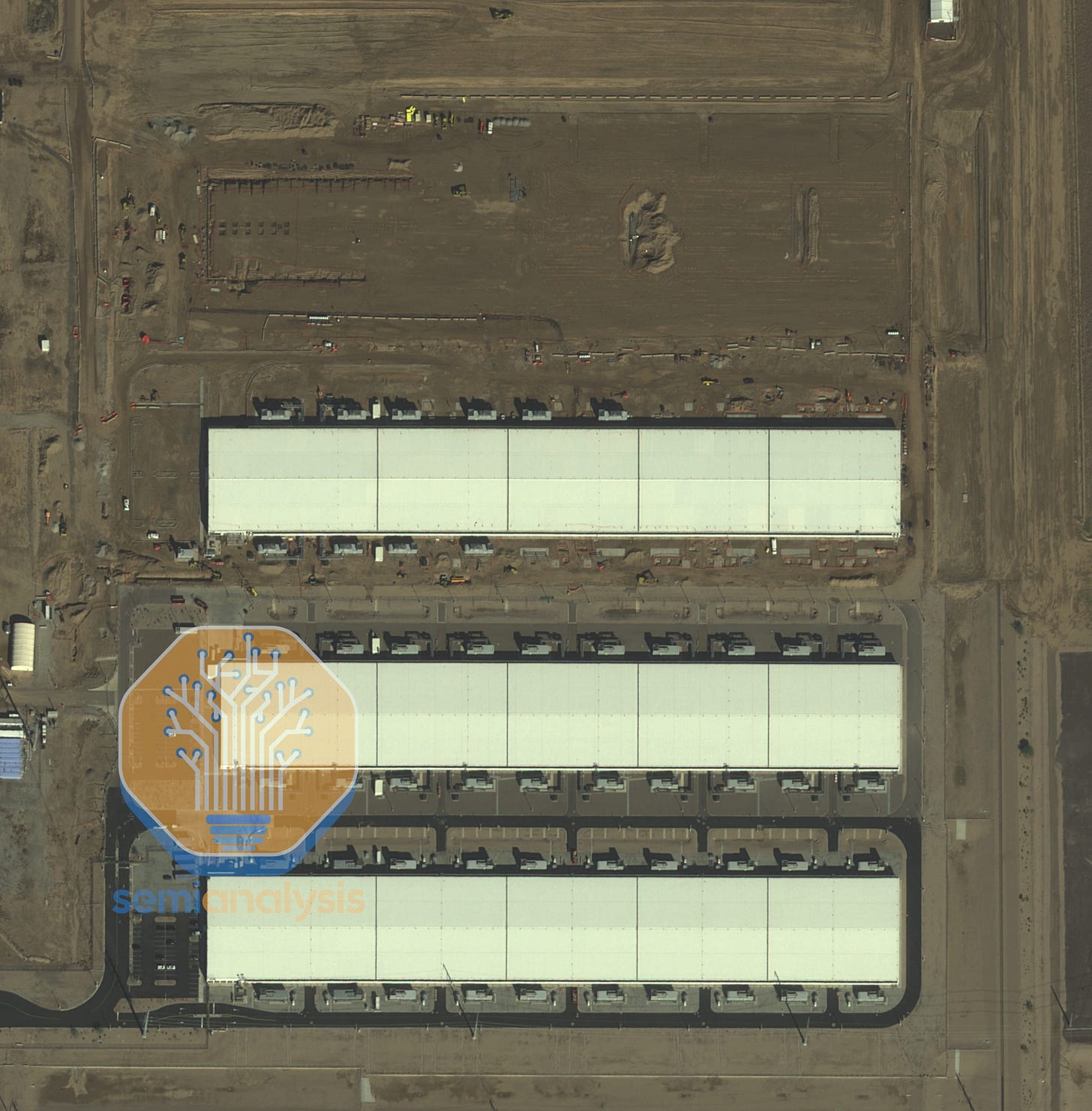
来源:SemiAnalysis,注:目前为止,这个 10 万集群中只有 4 栋建筑中的 3 栋已建成
In this Microsoft development cluster, each rack supports up to 40kW of power density, housing four H100 nodes per rack. This infrastructure features a distinct cabling setup where copper cables (notably the big black cable at the end of each row) are used for switch-to-switch connections within the rack. In contrast, the connections from the H100 servers to the leaf switches utilize multimode AOC fiber, identifiable by the blue cables.
在这个微软开发集群中,每个机架支持高达 40kW 的功率密度,每个机架容纳四个 H100 节点。该基础设施具有独特的布线设置,其中铜缆(特别是每排末端的大黑缆)用于机架内的交换机到交换机连接。相比之下,H100 服务器与叶子交换机之间的连接使用多模 AOC 光纤,可以通过蓝色电缆识别。
At the end of the day, the number one winner of these multiple 100k H100 clusters will be NVIDIA, as they make up the larger share of the BoM. In our accelerator model, we break down GPU and ASIC production/ASP by SKU, shipment by company. Going forward, with Broadcom dominating almost every hyperscaler clusters, their networking revenue will continue to soar. Nvidia will continue to have networking growth because many neoclouds, sovereigns, and entreprises will chose Nvidia’s reference design.
最终,这些多个 100k H100 集群的头号赢家将是 NVIDIA,因为它们占据了更大比例的物料清单。在我们的加速器模型中,我们按 SKU 分解 GPU 和 ASIC 的生产/平均销售价格,以及按公司分解的出货量。展望未来,随着博通几乎主导了每个超大规模集群,他们的网络收入将继续飙升。Nvidia 将继续实现网络增长,因为许多新云、主权国家和企业将选择 Nvidia 的参考设计。
SemiAnalysis is a multinational research and consulting firm across the US, Japan, Taiwan, Singapore, and France, with deep experience from engineering at ASML to building datacenters to training LLMs to hedge funds. Our research, data, and consulting serves the largest semiconductor, hyperscale, and AI companies in the industry. We also serve the private equity and public equity investment sectors. In addition to more offerings in the research business, we will also be launching an accelerator fund for early stage investments in AI, semiconductors, and the software infrastructure for both. We like people who have an immense amount of curiosity and figuring out how things truly work. Non-traditional backgrounds are okay. We have a high bar for quality, offer competitive pay, and great benefits.
SemiAnalysis 是一家跨国研究和咨询公司,业务遍及美国、日本、台湾、新加坡和法国,拥有从 ASML 的工程到数据中心建设再到对冲基金培训的丰富经验。我们的研究、数据和咨询服务于行业内最大的半导体、超大规模和人工智能公司。我们还为私募股权和公共股权投资领域提供服务。除了在研究业务中提供更多产品外,我们还将推出一个加速基金,专注于对人工智能、半导体及其软件基础设施的早期投资。我们喜欢那些充满好奇心并能真正理解事物运作方式的人。非传统背景也可以。我们对质量有很高的要求,提供具有竞争力的薪酬和优厚的福利。
We are hiring for the following roles to work on different parts of the research, consulting, and venture businesses.
我们正在招聘以下职位,以在研究、咨询和风险投资业务的不同部分工作。
AI analyst 人工智能分析师
Technical semiconductor analyst
技术半导体分析师Software analyst 软件分析师
Infrastructure and energy analyst
基础设施和能源分析师Auto, industrial, IOT semiconductor analyst
汽车、工业、物联网半导体分析师Memory, consumer semi, and consumer hardware analyst
内存、消费类半导体和消费类硬件分析师Robotics analyst 机器人分析师
Biotech and Healthcare analyst
生物技术和医疗保健分析师Venture analyst 风险分析师
Reach out at hello@semianalysis.com if you are interested.
如果您感兴趣,请通过 hello@semianalysis.com 联系我们。
So Cohere is not a massive long going forward then...?
所以 Cohere 未来不会是一个大规模的长期投资吗...?
4 条回复来自 Dylan Patel 及其他人
Love the power figures. Basically, anyone that chose to run it on solar would cut the cost by $80 million per year (with the cost staying flat.). Granted, for 150 MW you might need 1,500 acres (1MW for every ten acres in solar). That's inching to 3 square miles. But look at it this way. Upton County, Rex--home to the 497MW Roadrunner solar farm--covers 1241 square miles and 3,152 residents. The room exists.
喜欢这些电力数据。基本上,任何选择使用太阳能的人每年将节省 8000 万美元的成本(成本保持不变)。当然,对于 150 MW,你可能需要 1500 英亩(每 10 英亩 1 MW 的太阳能)。这接近 3 平方英里。但换个角度看。厄普顿县,雷克斯——497 MW 的 Roadrunner 太阳能农场的所在地——覆盖 1241 平方英里,居民 3152 人。空间是存在的。