Abstract 抽象
With the opportunities and challenges stemming from the artificial intelligence developments and its integration into society, AI literacy becomes a key concern. Utilizing quality AI literacy instruments is crucial for understanding and promoting AI literacy development. This systematic review assessed the quality of AI literacy scales using the COSMIN tool aiming to aid researchers in choosing instruments for AI literacy assessment. This review identified 22 studies validating 16 scales targeting various populations including general population, higher education students, secondary education students, and teachers. Overall, the scales demonstrated good structural validity and internal consistency. On the other hand, only a few have been tested for content validity, reliability, construct validity, and responsiveness. None of the scales have been tested for cross-cultural validity and measurement error. Most studies did not report any interpretability indicators and almost none had raw data available. There are 3 performance-based scale available, compared to 13 self-report scales.
人工智能发展及其融入社会带来的机遇和挑战,人工智能素养成为一个关键问题。利用高质量的 AI 素养工具对于理解和促进 AI 素养发展至关重要。本系统评价使用 COSMIN 工具评估了 AI 素养量表的质量,旨在帮助研究人员选择用于 AI 素养评估的工具。本综述确定了 22 项研究,验证了针对不同人群的 16 个量表,包括普通人群、高等教育学生、中等教育学生和教师。总体而言,量表表现出良好的结构效度和内部一致性。另一方面,只有少数应用程序经过了内容有效性、可靠性、结构有效性和响应性测试。没有一个量表经过跨文化效度和测量误差的测试。大多数研究没有报告任何可解释性指标,几乎没有研究有可用的原始数据。有 3 种基于表现的量表可用,而 13 种自我报告量表可用。
Similar content being viewed by others
其他人正在查看的类似内容
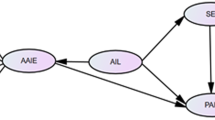
Introduction 介绍
The integration of Artificial Intelligence (AI) into various segments of society is increasing. In medicine, AI technologies can facilitate spine surgery procedures1, effectively operate healthcare management systems2,3, and provide accurate diagnosis based on medical imaging4. In education, AI systems contribute to effective teaching methods and enable accurate student assessments5. In science, AI plays a role in generating innovative hypotheses, surpassing the creative limits of individual researchers6 and aids scientific discovery7,8.
人工智能 (AI) 越来越多地融入社会的各个阶层。在医学方面,人工智能技术可以促进脊柱手术程序1,有效运行医疗保健管理系统2,3,并根据医学成像提供准确的诊断4。在教育领域,人工智能系统有助于采用有效的教学方法,并实现准确的学生评估5。在科学领域,人工智能在产生创新假设方面发挥着作用,超越了研究人员个人的创造性极限6,并有助于科学发现7,8。
With the increasing integration of AI in society, many new AI-related jobs are emerging, and many existing jobs now require AI re-skilling. Job postings requiring skills in machine learning and AI have significantly increased9,10. In the U.S., there was a dramatic rise in demand for AI skills from 2010 to 2019, surpassing the demand for general computer skills with AI proficiency providing a significant wage premium11. Furthermore, many companies have been reducing hiring in jobs not exposed to AI, suggesting a significant restructuring of the workforce around AI capabilities12.
随着 AI 在社会中的日益融合,许多与 AI 相关的新工作正在出现,许多现有工作现在需要 AI 再培训。需要机器学习和 AI 技能的职位发布显著增加 9,10。在美国,从 2010 年到 2019 年,对 AI 技能的需求急剧上升,超过了对一般计算机技能的需求,AI 熟练程度提供了显着的工资溢价11。此外,许多公司一直在减少未接触 AI 的工作的招聘,这表明围绕 AI 能力对员工队伍进行了重大重组12。
AI’s impact extends beyond the job market; it also alters the way people process information. It has enabled the production of deepfake audiovisual materials unrecognizable from reality with many websites casually offering services of face-swapping, voice-cloning, and deepfake pornography. Consequently, there has been a significant rise in fraud and cyberbullying incidents involving deepfakes13. The emergence of deepfakes has also led to a new generation of disinformation in political campaigns14. Research shows that people cannot distinguish deepfakes but their confidence in recognizing them is high, which suggests that they are unable to objectively assess their abilities15,16.
AI 的影响不仅限于就业市场;它还改变了人们处理信息的方式。它使无法从现实中辨认的深度伪造视听资料的制作成为可能,许多网站随意提供换脸、语音克隆和深度伪造色情服务。因此,涉及深度伪造的欺诈和网络欺凌事件显著增加13。深度伪造的出现也导致了政治竞选活动中的新一代虚假信息14。研究表明,人们无法区分深度伪造,但他们识别它们的信心很高,这表明他们无法客观地评估自己的能力15,16。
In the context of AI permeating job market and the spread of deepfakes, AI literacy becomes a key concern. As a recent concept, AI literacy has not yet been firmly conceptualized. AI literacy is often viewed as an advanced form of digital literacy17. In its basic definition, AI literacy is the ability to understand, interact with, and critically evaluate AI systems and AI outputs. A review aimed at conceptualizing AI literacy based on the adaptation of classic literacies proposed four aspects crucial for AI literacy—know and understand, use, evaluate, and understanding of ethical issues related to the use of AI18. Research and practice differ in specific expectations of AI literacy based on age—most agree that it should be part of education from early childhood education with more complex issues taught in older ages. While some authors argue that technical skills like programming should be a part of AI literacy, most agree it should encompass more generalizable knowledge and interdisciplinary nature19,20. Many global initiatives to promote AI literacy are emerging20 and AI literacy is becoming a part of the curriculum in early childhood education21, K-12 education22,23,24, as well as in higher education18,19 in several educational systems. At the same time, however, both researchers and educators pay little attention to development and understanding of instruments to assess AI literacy at different educational levels22.
在 AI 渗透就业市场和 deepfake 蔓延的背景下,AI 素养成为一个关键问题。作为一个最近的概念,AI 素养尚未被牢固地概念化。人工智能素养通常被视为数字素养的一种高级形式17。在其基本定义中,AI 素养是理解 AI 系统和 AI 输出、与之交互和批判性评估的能力。一项旨在根据经典素养的改编来概念化人工智能素养的审查提出了对人工智能素养至关重要的四个方面——认识和理解、使用、评估和理解与人工智能使用相关的道德问题18.研究和实践对 AI 素养的具体期望因年龄而异——大多数人都认为它应该是幼儿教育的一部分,在老年人中教授更复杂的问题。虽然一些作者认为编程等技术技能应该成为 AI 素养的一部分,但大多数人都同意它应该包含更普遍的知识和跨学科性质19,20。许多促进人工智能素养的全球举措正在出现20,人工智能素养正在成为幼儿教育21、K-12 教育22、23、24 以及高等教育18、19 课程的一部分。然而,与此同时,研究人员和教育工作者都很少关注评估不同教育阶段人工智能素养的工具的开发和理解22。
Utilizing quality AI literacy instruments is crucial for understanding and promoting AI literacy development. This systematic review will aim to aid both researchers and educators involved in research and evaluation of level and development of AI literacy. This systematic review has the following objectives:
利用高质量的 AI 素养工具对于理解和促进 AI 素养发展至关重要。本系统评价旨在帮助参与人工智能素养水平和发展研究和评估的研究人员和教育工作者。本系统综述的目标如下:
-
to provide a comprehensive overview of available AI literacy scales
提供可用 AI 素养量表的全面概述 -
to critically assess the quality of AI literacy scales
批判性地评估 AI 素养量表的质量 -
to provide guidance for research which AI literacy scales to use considering the quality of the scales and the context they are suitable for.
为研究提供指导,考虑到量表的质量及其适合的背景,使用哪些 AI 素养量表。
Results 结果
Overview of AI literacy scales
AI 素养量表概述
The initial search yielded 5574 results. After removing duplicate references, a total of 5560 studies remained. Figure 1 presents an overview of the literature search, screening, and selection process. During the initial screening, I manually reviewed titles and abstracts. In this step, I excluded 5501 records, which did not meet the inclusion criteria outlined in Methods section. I assessed the full texts of the remaining 59 records for eligibility and I checked their reference lists for other potentially relevant studies. After the full-text screening, I excluded 44 records. Most studies were excluded because they did not perform any scale validation, e.g. 25,26,27 or did not touch upon the concept of AI literacy28. AI4KGA29 scale was excluded because the author did not provide the full item list and did not respond to my request for it, making it questionable whether the scale can be used by anyone else. While self-efficacy is somewhat a distinct construct from self-reported AI literacy, the distinction between the two is heavily blurred. I therefore decided to adopt a more inclusive approach when assessing the relevancy of the measured constructs and included Morales-García et al.’s GSE-6AI30 and Wang & Chuang’s31 AI self-efficacy scale as well. I added one publication from the reference lists of the included studies to the final selection and six studies from the reverse searches, yielding a total of 22 studies validating or revalidating 16 scales.
初步检索产生了 5574 个结果。删除重复的参考文献后,总共保留了 5560 项研究。图 1 显示了文献检索、筛选和选择过程的概述。在初步筛选期间,我手动审查了标题和摘要。在此步骤中,我排除了 5501 条记录,这些记录不符合方法部分中概述的纳入标准。我评估了其余 59 条记录的全文是否合格,并检查了它们的参考文献列表以查找其他可能相关的研究。全文筛选后,我排除了 44 条记录。大多数研究被排除在外,因为它们没有进行任何规模验证,例如 25,26,27 或没有触及人工智能素养的概念28。AI4KGA29 量表被排除在外,因为作者没有提供完整的项目清单,也没有回应我的请求,这使得该量表是否可以被其他人使用成疑。虽然自我效能感在某种程度上与自我报告的 AI 素养不同,但两者之间的区别非常模糊。因此,我决定在评估测量结构的相关性时采用更具包容性的方法,并包括Morales-García等人的GSE-6AI30和Wang & Chuang的31 AI自我效能量表。我从纳入研究的参考文献列表中添加了 1 篇出版物到最终选择中,从反向检索中添加了 6 篇研究,总共产生了 22 项研究验证或重新验证了 16 个量表。
图 1:PRISMA 流程图。
Table 1 presents the studies’ basic descriptions. The included scales share several characteristics. Only a minority of the scales are performance-based32,33,34, with most scales relying on self-assessment-based Likert items30,31,35,36,37,38,39,40,41,42,43,44,45. Most scales have multiple factor structures. Constructing AI literacy scales has started only recently as all scales were constructed in the last three years, with the oldest being MAIRS-MS43 from 2021. MAIRS-MS43, SNAIL45, and AILS36 are also the only scales to this date, which have been revalidated by another study46,47,48,49,50,51. On the other hand, the scales vary by their target populations. Most of them target general population31,34,36,42,44,45,46,47 or higher education students30,32,37,38,39,43,48,49,50,51, with three of them targeting secondary education students33,35,41, and one targeting teachers40.
表 1 显示了研究的基本描述。包含的量表具有几个共同特征。只有少数量表是基于表现的32、33、34,大多数量表依赖于基于自我评估的李克特项目30、31、35、36、37、38、39、40、41、42、43、44、45。大多数尺度具有多因子结构。构建 AI 素养量表最近才开始,因为所有量表都是在过去三年中构建的,最古老的是 2021 年的 MAIRS-MS43。MAIRS-MS43、SNAIL45 和 AILS36 也是迄今为止唯一的量表,它们已被另一项研究46、47、48、49、50、51 重新验证。另一方面,规模因目标人群而异。他们中的大多数针对普通人群 31,34,36,42,44,45,46,47 或高等教育学生30,32,37,38,39,43,48,49,50,51,其中三个针对中学生33,35,41,一个针对中学生教师40.
表 1 秤特性
While the authors of the scales drew their conceptualizations of AI literacy from different sources and their scales target different populations, they largely overlap with core competencies comprising AI literacy. By looking at the authors’ conceptualizations of key competencies comprising AI literacy, virtually all scales recognize several core competencies as fundamental to AI literacy. First, they emphasize the technical understanding of AI, distinguishing it from mere general awareness about the technology. Secondly, they consider the societal impact of AI as a critical component. Lastly, AI ethics is acknowledged as an essential aspect. These competencies collectively form the foundational elements of AI literacy, and they are consistently present as factors across the various scales. There is a consensus among the authors of the scales about the three competencies being essential for both secondary and higher education students as well as general population and medical professionals. On the other hand, the authors of the scales differ in perceiving higher-order AI-related skills—creation and evaluation of AI—as components of AI literacy. In the original Ng et al.’s conceptualization18, creation and evaluation of AI are core components of AI literacy. MAILS42 drawing from the Ng et al.’s conceptualization18 identified creation of AI as a related, but separate construct from AI literacy. AILQ35, on the other hand, drawing from the same conceptualization includes creating AI as a core part of AI literacy. Several other scales also consider the ability to critically evaluate AI as a core part of AI literacy32,33,34,36,38,44. Considering the widespread integration of AI into daily and professional life, a question arises, whether the skills to create and critically evaluate AI will not have to be included as core competencies of AI literacy in near future, as those competencies might be crucial for functional AI literacy.
虽然量表的作者从不同的来源得出了他们对人工智能素养的概念,并且他们的量表针对不同的人群,但它们在很大程度上与构成人工智能素养的核心能力重叠。通过查看作者对构成 AI 素养的关键能力的概念化,几乎所有量表都认为几个核心能力是 AI 素养的基础。首先,他们强调对 AI 的技术理解,将其与仅仅对技术的一般认识区分开来。其次,他们将 AI 的社会影响视为一个关键组成部分。最后,人工智能伦理被认为是一个重要方面。这些能力共同构成了 AI 素养的基本要素,并且它们始终作为各种尺度的因素存在。量表的作者一致认为这三种能力对中学生和高等教育学生以及普通人群和医疗专业人员都是必不可少的。另一方面,量表的作者在将高阶 AI 相关技能(AI 的创建和评估)视为 AI 素养的组成部分方面存在差异。在最初的 Ng 等人的概念化18 中,AI 的创建和评估是 AI 素养的核心组成部分。MAILS42 借鉴了 Ng 等人的概念化18 将 AI 的创建确定为与 AI 素养相关但独立的结构。另一方面,AILQ35 借鉴了相同的概念,包括将 AI 作为 AI 素养的核心部分。其他几个量表也将批判性评估 AI 的能力视为 AI 素养的核心部分32,33,34,36,38,44。 考虑到人工智能广泛融入日常生活和职业生活,出现了一个问题,即在不久的将来,创造和批判性评估人工智能的技能是否不必被列为人工智能素养的核心能力,因为这些能力对于功能性人工智能素养可能至关重要。
Quality assessment 质量评估
I assessed the quality of the scales based on the COSMIN52,53,54,55,56 measurement properties and additionally on interpretability and feasibility. The Methods section provides a detailed explanation of these individual properties. Table 2 shows quality assessment of the scales based on the COSMIN52,53,54,55,56 and GRADE57 criteria. Overall, the scales demonstrated good structural validity and internal consistency. On the other hand, only a few have been tested for content validity, reliability, construct validity, and responsiveness. None of the scales have been tested for cross-cultural validity and measurement error. Most studies did not report any interpretability indicators and almost none reported scales’ average completion time (Tables 3 and 4).
我根据 COSMIN52、53、54、55、56 测量特性以及可解释性和可行性评估了量表的质量。的 Methods 部分提供了这些各个属性的详细说明。表 2 显示了基于 COSMIN52、53、54、55、56 和 GRADE57 标准的量表质量评估。总体而言,量表表现出良好的结构效度和内部一致性。另一方面,只有少数应用程序经过了内容有效性、可靠性、结构有效性和响应性测试。没有一个量表经过跨文化效度和测量误差的测试。大多数研究没有报告任何可解释性指标,几乎没有研究报告量表的平均完成时间(表 3 和 4)。
表 2 基于 COSMIN 和 GRADE 标准的量表质量评估
表 3 量表的可解释性指标
表 4 Scales 的可行性指标
AI literacy test32
AI 素养测试32
This is a performance-based scale assessing AI-related knowledge through 30 multiple-choice questions, each with a single correct option, and includes one sorting question. The authors used item response theory (IRT) models to confirm the scale’s single-factor structure. The authors drew from Long & Magerko’s58 conceptualization of AI literacy, which works with a set of 17 AI competencies grouped into five overarching areas: What is AI?, What can AI do?, How does AI work?, How should AI be used?, and How do people perceive AI?. The authors developed the scale primarily for higher-education students—the scale comprises both items which could be considered specialized advanced knowledge (e.g., distinguishing between supervised and unsupervised learning), but also basic general knowledge (e.g., recognizing areas of daily life where AI is used). However, the scale is arguably also suitable for any professionals who encounter AI in their work. There is some limited evidence for the scale’s content validity and high evidence for the scale’s structural validity, internal consistency, and construct validity. It is currently available in German and English, although English version has not yet been revalidated. It is possible that the content of some questions—especially those dealing with a typical use of AI in practice—will need to be changed in the future due to developments in AI, rendering some of the present items obsolete.
这是一个基于表现的量表,通过 30 道多项选择题评估 AI 相关知识,每道题都有一个正确的选项,并包括一个排序问题。作者使用项目反应理论 (IRT) 模型来确认量表的单因素结构。作者借鉴了Long & Magerko的58个AI素养概念化,该概念化涉及17项AI能力,分为五个总体领域::什么是AI,AI能做什么,AI如何工作,AI应该如何使用,以及人们如何感知AI)。作者主要为高等教育学生开发了该量表——该量表既包括可以被视为专业高级知识的项目(例如,区分监督学习和无监督学习),也包括基本一般知识(例如,识别日常生活中使用 AI 的领域)。然而,该量表可以说也适用于在工作中遇到 AI 的任何专业人士。量表的内容效度有一些有限的证据,而量表的结构效度、内部一致性和结构效度的证据量很高。它目前提供德语和英语版本,但英语版本尚未重新验证。由于 AI 的发展,一些问题的内容(尤其是那些涉及 AI 在实践中的典型使用的问题)在未来可能需要更改,从而使目前的一些项目过时。
AI-CI—AI literacy concept inventory assessment33
AI-CI—AI 素养概念量表评估33
AI-CI is a performance-based concept inventory scale for middle school students assessing AI-related knowledge through 20 multiple-choice questions. The authors used their own AI literacy curriculum59 to design the scale’s content. IRT was used for validation. There is good evidence for the scale’s content validity and structural validity, and high evidence for the scale’s internal consistency and responsiveness. It is currently available in English. The content of the items appears to be more general and less dependent on the context of AI developments compared to the AI literacy test32.
AI-CI 是一种基于表现的概念量表,面向中学生通过 20 道多项选择题评估 AI 相关知识。作者使用他们自己的 AI 素养课程59 来设计量表的内容。IRT 用于验证。该量表的内容效度和结构效度有充分的证据,量表的内部一致性和响应性也有充分的证据。它目前提供英文版本。与人工智能素养测试32 相比,这些项目的内容似乎更笼统,对人工智能发展背景的依赖性更小。
AILQ—AI literacy questionnaire35
AILQ — AI 素养问卷35
AILQ is aimed at secondary education students. The scale employs authors’ conceptualization of cognitive domains of AI literacy stemming from their exploratory review18 adding affective, behavioural, and ethical learning domains. The authors employed a CFA which resulted in identification of the scale’s four-factor structure paralleling the four learning domains. There is moderate positive evidence for the scale’s content validity, high positive evidence for the scale’s structural validity and internal consistency, and very low positive evidence for the scale’s responsiveness. It is currently available in English.
AILQ 面向中学生。该量表采用了作者对 AI 素养认知领域的概念化,该概念源于他们的探索性审查18 增加了情感、行为和道德学习领域。作者采用了 CFA,从而确定了量表的四因素结构与四个学习领域平行。量表的内容效度有中等的正面证据,量表的结构效度和内部一致性有很高的正面证据,而量表的反应性有极低的正面证据。它目前提供英文版本。
AILS—AI literacy scale36
AILS—AI 素养量表36
AILS scale targets general population in the context of human–AI interaction (HAII). The authors drew from their own conceptualization of AI literacy grounded in their literature review resulting in four constructs of AI literacy: awareness, use, evaluation, and ethics. The four constructs are parallel to the scale’s four factors confirmed by a CFA. The scale has since been revalidated in Turkish language46,47, however, no direct cross-cultural validation has been performed. There is very low positive evidence for the scale’s content validity, high positive evidence for the scale’s structural validity and internal consistency, low evidence for reliability, and high positive evidence for construct validity.
AILS 量表针对人机交互 (HAII) 背景下的普通人群。作者基于他们的文献综述,从他们自己对 AI 素养的概念化中汲取灵感,产生了 AI 素养的四种结构:意识、使用、评估和道德。这四个结构与 CFA 确认的量表的四个因素平行。此后,该量表已在土耳其语46,47 中重新验证,但是,尚未进行直接的跨文化验证。量表内容效度的正面证据非常低,量表的结构效度和内部一致性的正面证据很高,可靠性证据低,结构效度的正面证据很高。
AISES—AI self-efficacy scale (AISES)31
AISES—AI 自我效能量表 (AISES)31
AISES is aimed at assessing AI self-efficacy of general population. The scale’s conceptualization is grounded in previous technology-related self-efficacy research60,61. A CFA confirmed the scale’s four-factor structure. There is high positive evidence for the scale’s structural validity and internal consistency, however, content validation on the target population was not performed. It is currently available in English.
AISES 旨在评估普通人群的 AI 自我效能感。该量表的概念化基于先前与技术相关的自我效能研究60,61。CFA 证实了该量表的四因素结构。该量表的结构效度和内部一致性有很高的积极证据,但是,没有对目标人群进行内容验证。它目前提供英文版本。
Chan & Zhou’s EVT based instrument for measuring student perceptions of generative AI (knowledge of generative AI subscale)37
Chan & 周 基于 EVT 的工具,用于测量学生对生成式 AI 的看法(生成式 AI 分量表的知识)37
This subscale is part of a larger instrument aimed at assessing perceptions of generative AI of university students. Here, I reviewed only the subscale dealing with the self-perceived AI literacy. The authors drew from their own conceptualization of AI literacy grounded in their literature review. The items revolve around generative AI’s limitations and potential biases. CFA confirmed the subscale’s single-factor structure. There is high positive evidence for the subscale’s structural validity and internal consistency, however, content validation of the scale is disputable. It is currently available in English.
该分量表是旨在评估大学生对生成式 AI 的看法的更大工具的一部分。在这里,我只回顾了处理自我感知的 AI 素养的子量表。作者借鉴了他们自己基于文献综述的 AI 素养概念化。这些项目围绕生成式 AI 的局限性和潜在偏见展开。CFA 证实了该分量表的单因素结构。该分量表的结构有效性和内部一致性有很高的积极证据,但是,该量表的内容验证是有争议的。它目前提供英文版本。
ChatGPT literacy scale38
ChatGPT 识字量表38
The scale for college students is focused specifically on assessing AI literacy using ChatGPT. The scale is grounded in a Delphi survey performed by the authors. There is good evidence for the scale’s content validity and high evidence for the scale’s structural validity, internal consistency, and construct validity. The scale is available in English language.
大学生量表特别侧重于使用 ChatGPT 评估 AI 素养。该量表以作者进行的 Delphi 调查为基础。量表的内容效度有充分的证据,量表的结构效度、内部一致性和结构效度有高证据。该量表以英语提供。
GSE-6AI—brief version of the general self-efficacy scale for use with artificial intelligence30
GSE-6AI — 用于人工智能30 的一般自我效能量表的简要版本
The scale comprises only six items, making it suitable for a rapid assessment of AI self-efficacy. There is high positive evidence for the scale’s structural validity, internal consistency, and measurement invariance by gender, however, content validation on the target population was not performed. It is currently available in Spanish and English.
该量表仅包含六个项目,使其适合快速评估 AI 自我效能感。该量表的结构效度、内部一致性和性别测量不变性有高度的积极证据,但是,未对目标人群进行内容验证。它目前提供西班牙语和英语版本。
Hwang et al.’s digital literacy scale in the artificial intelligence era for college students39
Hwang et al. 的人工智能时代大学生数字素养量表39
This scale targets higher education students and the authors also largely drew from Long & Magerko’s58 conceptualization of AI literacy. The authors employed a CFA which resulted in identification of the scale’s four-factor structure. There is high positive evidence for the scale’s structural validity and internal consistency, however, content validation on the target population was not performed. It is currently available in English.
这个量表针对的是高等教育学生,作者也主要借鉴了Long&Magerko的58个AI素养概念化。作者采用了 CFA,从而确定了量表的四因素结构。该量表的结构效度和内部一致性有很高的积极证据,但是,没有对目标人群进行内容验证。它目前提供英文版本。
Intelligent TPACK—technological, pedagogical, and content knowledge scale40
智能 TPACK — 技术、教学和内容知识量表40
Intelligent-TPACK aims to assess teachers’ self-perceived level of AI-related knowledge necessary for integration of AI in their pedagogical work. It draws from the TPACK framework62 adding an aspect of AI ethics. The scale assesses teachers’ knowledge of four AII-based tools: Chatbots, intelligent tutoring systems, dashboards, and automated assessment systems arguing that those are the most prevalent AI-based technologies in K-12 education. A CFA showed scale’s five-factor structure comprising the original TPACK dimensions with ethics. There is high positive evidence for the scale’s structural validity and internal consistency, however, content validation on the target population was not performed. It is currently available in English.
Intelligent-TPACK 旨在评估教师自我感知的人工智能相关知识水平,这是将人工智能整合到教学工作中所必需的。它借鉴了 TPACK 框架62,增加了 AI 伦理的一个方面。该量表评估了教师对四种基于 AII 的工具的了解:聊天机器人、智能辅导系统、仪表板和自动评估系统,认为这些是 K-12 教育中最普遍的基于 AI 的技术。CFA 显示了量表的五因素结构,包括原始 TPACK 维度和道德。该量表的结构效度和内部一致性有很高的积极证据,但是,没有对目标人群进行内容验证。它目前提供英文版本。
Kim & Lee’s artificial intelligence literacy scale for middle school students41
Kim & Lee的中学生人工智能素养量表41
This scale targets secondary education students. The authors drew from an ad hoc expert group’s conceptualization of AI literacy revolving around AI’s societal impact, understanding of AI, AI execution plans, problem solving, data literacy, and ethics. The authors employed a CFA which resulted in identification of the scale’s six-factor structure. There is some limited positive evidence for the scale’s content validity and high evidence for the scale’s structural validity, internal consistency, and construct validity. So far, the scale is only available in Korean.
该量表针对中学生。作者借鉴了一个特设专家组对 AI 素养的概念化,该概念围绕 AI 的社会影响、对 AI 的理解、AI 执行计划、问题解决、数据素养和道德规范展开。作者采用了 CFA,从而确定了量表的六因素结构。量表的内容效度有一些有限的正面证据,而量表的结构效度、内部一致性和结构效度则有高证据。到目前为止,该量表仅提供韩语版本。
MAILS—meta AI literacy scale42
MAILS—元 AI 素养量表42
MAILS is a general-population scale developed from Ng et al.’s18 conceptualization of AI literacy with four areas: know and understand AI, use and apply AI, evaluate and create AI, and AI Ethics. Additionally, it includes further psychological competencies related to the use of AI above the Ng et al.’s18 areas of AI Literacy—self-efficacy and self-perceived competency. It is the most extensive instruments out of the reviewed instruments. Resulting from a confirmatory factor analysis (CFA), the four AI literacy areas were not found to be all part of a single AI literacy concept—creating AI was found to be a separate factor. The authors made the scale modular in a sense that each of the resulting factors can be measured independently of each other—AI literacy (18 items), create AI (4 items), AI self-efficacy (6 items), and AI self-competency (6 items). There is high positive evidence for the scale’s structural validity, internal consistency, and construct validity, however, content validation on the target population was not performed. It is currently available in German and English, although English version has not yet been revalidated. There is evidence that the scale has good interpretability, although the scale shows some indication of floor effects for five items and ceiling effect for one item. The scale is feasible for a quick assessment of AI literacy, with most participants completing the scale within 20 min.
MAILS 是根据 Ng 等人的18 个 AI 素养概念化发展而来的一般人群量表,分为四个领域:了解和理解 AI、使用和应用 AI、评估和创造 AI 以及 AI 伦理。此外,它还包括与 AI 使用相关的其他心理能力,位于 Ng 等人的 AI 素养的18 个领域之上——自我效能感和自我感知能力。它是所审查的工具中最广泛的工具。根据验证性因子分析 (CFA) 的结果,发现四个 AI 素养领域并非都是单个 AI 素养概念的一部分——创建 AI 被发现是一个单独的因素。作者将量表模块化,因为每个结果因素都可以相互独立地衡量——AI 素养(18 项)、创建 AI(4 项)、AI 自我效能感(6 项)和 AI 自我能力(6 项)。该量表的结构效度、内部一致性和结构效度有很高的积极证据,但是,未对目标人群进行内容验证。它目前提供德语和英语版本,但英语版本尚未重新验证。有证据表明该量表具有良好的可解释性,尽管该量表显示了 5 个项目的地板效应和 1 个项目的天花板效应。该量表对于快速评估 AI 素养是可行的,大多数参与者在 20 分钟内完成了量表。
MAIRS-MS—medical artificial intelligence readiness scale for medical students43
MAIRS-MS—医学生医学人工智能准备量表43
MAIRS-MS is aimed at medical students and the authors developed it from conceptualization of AI readiness of both professionals and medical students. Originally developed for Turkish medical students, the scale has since been revalidated in Persian language in Iran48, however, no direct cross-cultural validation has been performed. CFAs on two samples43,48 confirmed the scale’s four-factor structure. There is some limited positive evidence for the scale’s content validity and high evidence for the scale’s structural validity, internal consistency, and invariance by gender.
MAIRS-MS 针对医学生,作者从专业人士和医学生的 AI 就绪性的概念化中发展出来。该量表最初是为土耳其医学生开发的,此后在伊朗48 的波斯语中被重新验证,但是,没有进行直接的跨文化验证。两个样本的 CFA43,48 证实了该量表的四因素结构。该量表的内容效度有一些有限的正面证据,而该量表的结构效度、内部一致性和性别不变性的证据则很高。
Pinski & Belian’s instrument44
Pinski & Belian 的乐器44
This scale targets general population. The authors draw from their own conceptualization of AI literacy grounded in their literature review. The authors employed a structural equation model to come to the scale’s five-factor structure. Due to a limited sample size, there is only limited positive evidence for the scale’s content and structural validity, and medium evidence for internal consistency. It is currently available in English.
该量表以一般人群为目标。作者借鉴了他们自己基于文献综述的 AI 素养概念化。作者采用结构方程模型得出量表的五因子结构。由于样本量有限,该量表的内容和结构效度的积极证据有限,内部一致性的证据有限。它目前提供英文版本。
SAIL4ALL—the scale of artificial intelligence literacy for all34
SAIL4ALL - 所有人的人工智能素养量表34
SAIL4ALL is a general-population scale comprising four distinct subscales, which can be used independently. However, the individual subscales cannot be aggregated to get an overall AI literacy score. The scale can also be used in both true/false and Likert-scale format. The authors drew from Long & Magerko’s58 conceptualization of AI literacy. Content validation on the target population was not performed. There is mixed evidence for the scale’s structural validity and internal consistency. On the one hand, a two-factor “What is AI?” subscale, a single-factor “How does AI work?”, and a single-factor “How should AI be used?” show good structural validity and internal consistency in both true/false and Likert scale format. On the other hand, “What can AI do?” subscale shows poor structural validity and internal consistency. There is an indication that the scale suffers from the ceiling effect.
SAIL4ALL 是一个由四个不同的分量表组成的一般人群量表,可以独立使用。但是,不能汇总各个分量表以获得总体 AI 素养分数。该量表也可以以 true/false 和 Likert 量表格式使用。作者借鉴了Long&Magerko的58个AI素养概念化。未对目标群体执行内容验证。该量表的结构效度和内部一致性的证据好坏参半。一方面,双因素 “What is AI?” 子量表、单因素 “How does AI work?” 和单因素 “How should be used?” 在真/假和李克特量表格式中都显示出良好的结构效度和内部一致性。另一方面,“AI can do do?” 子量表显示出较差的结构效度和内部一致性。有迹象表明,该秤受到天花板效应的影响。
SNAIL—scale for the assessment of non-experts’ AI literacy45
SNAIL - 非专家 AI 素养评估量表45
SNAIL is a general-population scale developed from the authors’63 extensive Delphi expert study’s conceptualization of AI literacy. The authors used an exploratory factor analysis to assess the scale’s factor structure resulting in a three-factor TUCAPA model of AI literacy—technical understanding, critical appraisal, and practical application. The scale has since been revalidated in Turkish language50 and in German language and for the use of learning gains using retrospective-post-assessment49, however, no direct cross-cultural validation has been performed. There is high positive evidence for the scale’s structural validity and internal consistency, and due to a small longitudinal sample size, only limited evidence for the scale’s reliability and responsiveness. Content validation on the target population was not performed in any of the four studies45,49,50,51, nor in the Delphi study63. There is an indication that the scale suffers from the floor effect, with almost half of the items having >15% responses with the lowest possible score. The scale is feasible for a quick assessment of AI literacy, with most participants completing the scale within 10 min.
SNAIL 是根据作者 63 广泛的 Delphi 专家研究对 AI 素养的概念化发展而来的一般人群量表。作者使用探索性因子分析来评估量表的因子结构,从而产生人工智能素养的三因素 TUCAPA 模型——技术理解、批判性评估和实际应用。此后,该量表在土耳其语50 和德语中进行了重新验证,并使用回顾性后评估49 来使用学习收益,但是,没有进行直接的跨文化验证。该量表的结构有效性和内部一致性有很高的积极证据,并且由于纵向样本量小,该量表的可靠性和响应性的证据有限。四项研究45、49、50、51 和 Delphi 研究63 均未对目标人群进行内容验证。有迹象表明,该量表受到地板效应的影响,几乎一半的项目的回答>15%,得分最低。该量表可用于快速评估 AI 素养,大多数参与者在 10 分钟内完成量表。
Discussion 讨论
This review identified 22 studies (re)validating 16 scales designed to assess AI literacy. Unfortunately, none of the scales showed positive evidence for all COSMIN measurement properties and most studies suffered from poor methodological rigour. Furthermore, the scales’ interpretability and feasibility also remain largely unknown due to most studies not reporting the necessary indicators, and, with an exception of Laupichler et al.45,49, not providing open data. By not providing public open data, the authors not only prevent calculations of some of the relevant quality indicators but may also contribute to the replicability crisis in science. Most studies did not report percentages of missing data and strategies they employed to address missing data, which puts their credibility into question.
本综述确定了 22 项研究(重新)验证了 16 个旨在评估 AI 素养的量表。不幸的是,没有一个量表显示所有 COSMIN 测量特性的积极证据,并且大多数研究的方法学严谨性较差。此外,由于大多数研究没有报告必要的指标,并且除了 Laupichler 等人45,49 没有提供开放数据,因此量表的可解释性和可行性在很大程度上也仍然未知。由于不提供公开的开放数据,作者不仅阻止了某些相关质量指标的计算,而且还可能导致科学中的可复制性危机。大多数研究没有报告缺失数据的百分比以及他们为解决缺失数据而采用的策略,这使他们的可信度受到质疑。
Considering the overall limited evidence for the quality of the scales, I will formulate recommendations drawing mainly from the COSMIN priorities considering content validity the most important measurement property, the scales’ potential for efficient revalidation, and the target populations.
考虑到量表质量的总体证据有限,我将主要根据 COSMIN 的优先事项制定建议,考虑内容有效性最重要的测量属性、量表有效重新验证的潜力以及目标人群。
When aiming for an assessment of general population, AILS36 is the scale with the most robust quality evidence. It showed at least some evidence for content validity and reliability, while showing good evidence for structural validity and internal consistency. Also, it has been revalidated in another two studies46,47. Pinski & Belian’s instrument44 also showed at least some evidence for content validity, but it has been validated on a limited sample, requiring revalidation on a bigger sample in the future. The following general population scales did not include target population in the content validation phase. SNAIL45 was constructed on an elaborate Delphi study63, it has been revalidated in another three studies49,50,51 including one with comparative self-assessment gains49, it is one of the few scales with evidence of reliability and responsiveness, and it demonstrated good structural validity and internal consistency, which makes it a promising instrument. In the future, it is important to check the scale’s content validity on general population and investigate the floor effect. MAILS42 is also a promising instrument, with good evidence for structural validity, internal consistency, and construct validity. It is the only scale with evidence for minimal floor and ceiling effects. In the future, it is important to check the scale’s content validity on general population. AISES31 also showed good evidence for structural validity and internal consistency, but as with the previous two instruments, it is important to check the scale’s content validity on general population. Lastly, most SAIL4ALL34 subscales showed good evidence for structural validity and internal consistency, however, the psychometric properties of “What can AI do?” subscale are questionable. SAIL4ALL is currently the only available performance-based scale targeting general population.
当以评估一般人群为目标时,AILS36 是具有最有力质量证据的量表。它至少显示了一些内容有效性和可靠性的证据,同时显示了结构有效性和内部一致性的良好证据。此外,它还在另外两项研究中得到了重新验证46,47。Pinski & Belian的44号仪器也至少显示出一些内容有效性的证据,但它已经在有限的样本上得到了验证,需要在未来更大的样本上重新验证。以下一般群体量表在内容验证阶段不包括目标群体。SNAIL45 是在精心设计的 Delphi 研究63 的基础上构建的,它已在另外三项研究49,50,51 中得到重新验证,其中包括一项具有比较自我评估收益49,它是为数不多的具有可靠性和响应性证据的量表之一,它表现出良好的结构有效性和内部一致性,这使其成为一种有前途的工具。未来,检查量表对普通人群的内容效度并研究地板效应非常重要。MAILS42 也是一种很有前途的工具,在结构效度、内部一致性和结构效度方面有很好的证据。它是唯一有证据表明地板和天花板影响最小的秤。将来,检查量表在一般人群中的内容效度非常重要。 AISES31 也显示了结构效度和内部一致性的良好证据,但与前两种工具一样,检查量表在一般人群中的内容效度很重要。最后,大多数34 SAIL4ALL量表显示出结构效度和内部一致性的良好证据,然而,“AI 能做什么”子量表的心理测量特性值得怀疑。SAIL4ALL 是目前唯一针对普通人群的基于表现的量表。
When aiming for an assessment of higher education students, AI literacy test32 and ChatGPT literacy scale38 are the scales with the most robust quality evidence. Both showed at least some evidence for content validity while showing good evidence for structural validity, internal consistency, and construct validity. AI literacy test32 is the only performance-based scale available now targeting higher education students. MAIRS-MC43 also showed at least some evidence for content validity while showing good evidence for structural validity and internal consistency. GSE-6AI30, Hwang et al.’s instrument39, and Chan & Zhou’s EVT based instrument (knwl. of gen. AI subscale)37 are also promising instruments with good evidence for structural validity and internal consistency, however, their content validity needs to be checked on the higher-education students. GSE-6AI30, MAIRS-MC43, and SNAIL45 have been validated specifically for medical students, which makes them the instruments of choice if medical students are to be assessed.
在对高等教育学生进行评估时,AI 素养测试32 和 ChatGPT 素养量表38 是具有最有力质量证据的量表。两者都至少显示了一些内容有效性的证据,同时也显示了结构有效性、内部一致性和结构有效性的良好证据。AI 素养测试32 是目前唯一针对高等教育学生的基于表现的量表。MAIRS-MC43 还显示了至少一些内容有效性的证据,同时显示了结构有效性和内部一致性的良好证据。GSE-6AI30、Hwang 等人的仪器39 以及 Chan & 周 基于 EVT 的仪器(gen. AI 分量表的 knwl.)37 也是有前途的工具,具有结构有效性和内部一致性的良好证据,但是,它们的内容有效性需要在高等教育学生身上进行检查。GSE-6AI30、MAIRS-MC43 和 SNAIL45 已专门针对医学生进行了验证,这使它们成为医学生接受评估的首选工具。
When aiming for an assessment of secondary education students, AI-CI33, AILQ35 and Kim & Lee’s instrument41 all provided evidence for content validity, structural validity, and internal consistency, although AI-CI33 and AILQ35 had higher level of evidence for content validity and provided evidence for responsiveness. The decision between the two instruments might, to some degree, be guided by the languages they are available in, with AI-CI33 and AILQ35 currently available only in English, and Kim & Lee’s instrument41 only in Korean.
在针对中等教育学生进行评估时,AI-CI33、AILQ35和Kim & Lee的41工具都提供了内容有效性、结构有效性和内部一致性的证据,尽管AI-CI33和AILQ35对内容有效性的证据级别更高,并为响应性提供了证据。在某种程度上,这两种乐器之间的决定可能取决于它们可用的语言,AI-CI33和AILQ35目前只有英文版本,而Kim & Lee的乐器41只有韩语版本。
When aiming for an assessment of teachers’ perceived readiness to implement AI into their pedagogical practice, Intelligent TPACK40 in the only instrument available now. It showed good evidence for structural validity and internal consistency, however, its content validity needs to be checked on the teachers.
当旨在评估教师是否准备好将 AI 实施到他们的教学实践中时,Intelligent TPACK40 是目前唯一可用的工具。它显示了结构有效性和内部一致性的良好证据,但是,其内容有效性需要在教师身上进行检查。
There are several general recommendations for future research. Cross-cultural validity, measurement error, and floor and ceiling effects of the existing scales should be checked. If the authors of the scales made the raw data open, it would solve many problems as, for example, multiple group factor analyses require raw data for comparison. With a single performance-based scale available32 targeting higher education students, it might be beneficial to design performance-based scales aimed at different populations as well. It would also be beneficial to cross-validate the results of the performance-based and self-report scales. Finally, it will be necessary to review the state of AI literacy scales in the future and update the current quality assessment.
对于未来的研究,有几项一般性建议。应检查现有量表的跨文化效度、测量误差以及下限和上限效应。如果量表的作者公开了原始数据,它将解决许多问题,例如,多组因子分析需要原始数据进行比较。由于针对高等教育学生的单一基于表现的量表32 可用,因此设计针对不同人群的基于表现的量表也可能是有益的。交叉验证基于绩效的量表和自我报告量表的结果也是有益的。最后,有必要在未来审查人工智能素养量表的状况并更新当前的质量评估。
This review has some limitations. It was performed by a single author, which might have caused some bias in the scales’ quality assessment, despite the COSMIN quality criteria being straightforwardly and quantitatively stated in the COSMIN manuals. Then, some AI literacy scales might have been missed if published in grey literature, since the search was limited to Scopus and arXiv. However, the chances of missing some relevant scales were reduced by the reversed search in Scopus and Google Scholar.
本综述有一些局限性。它由一位作者进行,尽管 COSMIN 手册中对 COSMIN 质量标准进行了直接和定量的说明,但这可能会导致量表的质量评估出现一些偏差。然后,如果以灰色文献形式发表,可能会错过一些 AI 素养量表,因为搜索仅限于 Scopus 和 arXiv。然而,在 Scopus 和 Google Scholar 中反向搜索降低了错过某些相关量表的机会。
Methods 方法
To address the objectives of this study, I employed a systematic review followed by a quality assessment of AI literacy scales. I performed the review in accordance with the updated PRISMA 2020 guidelines64. The study was preregistered at OSF at https://osf.io/tcjaz.
为了实现这项研究的目标,我采用了系统评价,然后对 AI 素养量表进行了质量评估。我根据更新的 PRISMA 2020 指南64 进行了综述。该研究于 https://osf.io/tcjaz 日在 OSF 进行了预注册。
Literature search 文献检索
I conducted the literature search on June 18, 2024, ensuring coverage of all literature available up to mid-2024. Initially, I conducted the search on January 1, 2024, as planned in the preregistration. However, due to the dynamically evolving field, I decided to redo the search during the first round of peer review to include the most up-to-date sources. I searched for literature in two databases—Scopus and arXiv. Scopus served as a primary database for peer-reviewed articles with arXiv supplementing Scopus with its coverage of pre-prints. I created search strings (Table 5) after an iterative process of finding and adding relevant terms and removing terms yielding irrelevant results65. I set no limits on publication date, publication type, or publication stage. In Scopus, I searched in titles, abstracts, and keywords; in arXiv, I searched in all fields. In Scopus, I limited the search to English papers. Additionally, in conjunction with the database searches, I looked for sources in reference lists of the included studies, as well as by a reversed search by works citing the included studies in Scopus and Google Scholar on June 20, 2024.
我于 2024 年 6 月 18 日进行了文献检索,确保覆盖了截至 2024 年年中的所有可用文献。最初,我按照预注册的计划在 2024 年 1 月 1 日进行了搜索。然而,由于该领域不断发展,我决定在第一轮同行评审期间重新进行搜索,以包括最新的来源。我在两个数据库中检索了文献 — Scopus 和 arXiv。Scopus 是同行评审文章的主要数据库,arXiv 补充了 Scopus 的预印本覆盖范围。在查找和添加相关术语并删除产生不相关结果的术语的迭代过程之后,我创建了搜索字符串(表 5)65。我对发布日期、发布类型或发布阶段没有限制。在 Scopus 中,我搜索了标题、摘要和关键词;在 arXiv 中,我搜索了所有字段。在 Scopus 中,我将检索范围限制在英文论文中。此外,结合数据库搜索,我在纳入研究的参考文献列表中寻找来源,并于 2024 年 6 月 20 日在 Scopus 和 Google Scholar 中通过引用纳入研究的作品进行反向搜索。
Inclusion criteria 纳入标准
Studies met the inclusion criteria if they: (1) developed new or revalidated existing AI literacy scale, (2) provided the full item list, (3) described how the items were formulated, (4) described the study participants, and (5) described validation techniques used in the scale development.
如果研究符合纳入标准,则:(1) 开发了新的或重新验证的现有 AI 素养量表,(2) 提供了完整的项目列表,(3) 描述了项目的制定方式,(4) 描述了研究参与者,以及 (5) 描述了量表开发中使用的验证技术。
Data extraction 数据提取
I extracted the following data from the studies: name(s) of the author(s), date of the publication, scale type (self-report or performance-based), number and type of the items, language(s) that the scale is available in, target population, participant characteristics, factor extraction method, factor structure, and data related to the quality assessment procedure as described in the Quality assessment section. I emailed authors for information missing in the articles—often the age distributions of the participants—and, when available, I also used published datasets to compute the missing information. Most information on completion time, missing data, and floor and ceiling effects were calculated from the published datasets.
我从研究中提取了以下数据:作者姓名、出版日期、量表类型(自我报告或基于绩效)、项目的数量和类型、量表可用的语言、目标人群、参与者特征、因子提取方法、因子结构以及与质量评估程序相关的数据,如质量评估部分所述。我向作者发送电子邮件,询问文章中缺失的信息(通常是参与者的年龄分布),并且,如果可用,我还使用已发布的数据集来计算缺失的信息。有关完成时间、缺失数据以及下限和上限效应的大部分信息都是根据已发布的数据集计算的。
Quality assessment 质量评估
First, I evaluated methodological quality of the individual studies by using the COnsensus‑based Standards for the selection of health Measurement INstruments (COSMIN)52,53,54 for the self-report scales, and additionally the COSMIN Risk of Bias tool to assess the quality of studies on reliability or measurement error of outcome measurement instruments55 for the performance-based scales. While the COSMIN tool was originally devised for the medical field, it has since been used in both psychological66,67 and educational research68. The psychometric qualities of self-reports are generally consistent across these fields, making the COSMIN tool satisfactory for use in diverse research areas.
首先,我通过使用基于 COnsensus 的健康测量工具选择标准 (COSMIN)52,53,54 作为自我报告量表,以及 COSMIN 偏倚风险工具来评估结果测量工具的可靠性或测量误差的研究质量55对于基于性能的量表。虽然 COSMIN 工具最初是为医学领域设计的,但此后它已被用于心理学66,67 和教育研究68。自我报告的心理测量质量在这些领域中通常是一致的,这使得 COSMIN 工具在不同的研究领域中得到令人满意的使用。
Drawing from the COSMIN tool, I assessed the scales based on the measurement properties of content validity, structural validity, internal consistency, cross-cultural validity, reliability, measurement error, construct validity, and responsiveness. I did not evaluate the scales based on the criterion validity as suggested in the COSMIN tool because as of January 2024, there was no gold standard tool for measuring AI literacy. I assessed each measurement property by a box containing several questions scored on the scale of very good, adequate, doubtful, and inadequate, according to the defined COSMIN criteria56. A system of worst score counts applied for each box. Additionally, I assessed the scales based on the criteria of interpretability and feasibility—while not being measurement properties, COSMIN recognizes them as important characteristics of the scales.
从 COSMIN 工具中汲取灵感,我根据内容效度、结构效度、内部一致性、跨文化效度、可靠性、测量误差、结构效度和响应性的测量属性评估了量表。我没有根据 COSMIN 工具中建议的标准有效性来评估量表,因为截至 2024 年 1 月,还没有衡量 AI 素养的黄金标准工具。我通过一个包含几个问题的方框评估了每个测量属性,根据定义的 COSMIN 标准56,按非常好、充分、可疑和不足的等级评分。适用于每个框的最差分数计数系统。此外,我根据可解释性和可行性标准评估了量表——虽然不是测量属性,但 COSMIN 将它们视为量表的重要特征。
Then, I applied the criteria for good measurement properties by using COSMIN quality criteria for the individual studies. The criteria assess the measurement properties on a scale of sufficient, insufficient, and indeterminate. Studies assessed as sufficient on some measurement property had to report a given metrics and the metrics had to be above a quality threshold set by COSMIN. On the other hand, studies assessed as insufficient on some measurement property reported a given metrics, but the metrics was under the quality threshold set by COSMIN, while studies assessed as indeterminate on some measurement property did not report a given metrics.
然后,我通过使用 COSMIN 质量标准对单个研究应用了良好测量特性的标准。该标准在充分、不足和不确定的范围内评估测量属性。在某些测量特性上被评估为足够的研究必须报告给定的指标,并且这些指标必须高于 COSMIN 设定的质量阈值。另一方面,在某些测量属性上评估为不足的研究报告了给定的指标,但这些指标低于 COSMIN 设定的质量阈值,而在某些测量属性上评估为不确定的研究没有报告给定的指标。
Finally, I synthetized the evidence per measurement property per scale. I rated the overall results against the criteria for good measurement properties and used the Grading of Recommendations Assessment, Development, and Evaluation (GRADE) approach for systematic reviews of clinical trials57 to come to a final scale-level quality rating. In case of the scales which have been revalidated, I pooled the estimates from the individual studies with a random-effect meta-analysis in R69 package metafor70 and gave rating based on the pooled estimates. The individual methodological quality ratings as well as the quality criteria ratings with the COSMIN thresholds are available as Supplementary Data 1. Table 6 shows the interpretation of the overall levels of evidence for the quality of the measurement properties.
最后,我综合了每个量表的每个测量属性的证据。我根据良好测量特性的标准对总体结果进行了评级,并使用建议分级评估、开发和评估 (GRADE) 方法对临床试验进行系统评价57,以得出最终的量表级质量评级。在已重新验证的量表的情况下,我将单个研究的估计值与 R69 包 metafor70 中的随机效应荟萃分析合并,并根据合并估计值给出评分。单个方法质量评级以及具有 COSMIN 阈值的质量标准评级可作为补充数据 1 获得。表 6 显示了对测量特性质量的总体证据水平的解释。
表 6 测量属性质量的总体证据水平
Content validity 内容有效性
Content validity refers to the degree to which the instrument measures the construct(s) it purports to measure71. COSMIN considers content validity the most important measurement property of an instrument as it should be ensured that the instrument is relevant, comprehensive, and comprehensible with respect to the construct of interest and study population54. COSMIN requires that both experts and target population are involved in content validation for content validity to be considered adequate.
内容效度是指工具测量其声称测量的结构的程度71。COSMIN 认为内容有效性是工具最重要的测量属性,因为应确保该工具与感兴趣的结构和研究人群的结构相关、全面且易于理解54。COSMIN 要求专家和目标人群都参与内容验证,才能将内容有效性视为充分。
Structural validity 结构有效性
Structural validity refers to the degree to which the instrument scores are an adequate reflection of the dimensionality of the construct to be measured. COSMIN requires that factor analyses or IRT/Rasch analyses are used to assess structural validity71.
结构效度是指乐器分数充分反映待测结构维度的程度。COSMIN 要求使用因子分析或 IRT/Rasch 分析来评估结构效度71。
Internal consistency 内部一致性
Internal consistency refers to the degree to which the items are interrelated. COSMIN requires Cronbach’s alpha(s) to be calculated for each unidimensional scale or subscale71.
内部一致性是指项目相互关联的程度。COSMIN 要求计算每个单维量表或子量表71 的 Cronbach's alpha(s)。
Measurement invariance 测量不变性
Measurement invariance refers to the degree to which the factor structure remains same for various subgroups—i.e., gender, age, or level of education—and whether the items exhibit Differential Item Functioning (DIF). COSMIN requires multiple group factor analysis or DIF analysis to be used to assess measurement invariance71.
测量不变性是指各个子组(即性别、年龄或教育水平)的因子结构保持不变的程度,以及项目是否表现出差异项目功能 (DIF)。COSMIN 需要使用多组因子分析或 DIF 分析来评估测量不变性71。
Cross-cultural validity 跨文化有效性
Cross-cultural validity refers to the degree to which the performance of the items on a translated or culturally adapted scale are an adequate reflection of the performance of the items of the original version of the scale. COSMIN requires multiple group factor analysis or DIF analysis to be used to assess cross-cultural validity71.
跨文化效度是指项目在翻译或文化改编的量表上的表现在多大程度上充分反映了原始版本的量表中项目的表现。COSMIN 要求使用多群体因素分析或 DIF 分析来评估跨文化效度71。
Reliability 可靠性
Reliability refers to the proportion of total variance in the measurement which is because of true differences among participants. COSMIN requires reliability to be assessed by intra-class correlation coefficients or weighted Kappa and it requires multiple observations in time71.
可靠性是指测量中总方差的比例,这是因为参与者之间的真实差异。COSMIN 要求通过类内相关系数或加权 Kappa 来评估可靠性,并且需要在时间71 中进行多次观测。
Measurement error 测量误差
Measurement error refers to the systematic and random error of participants’ scores which are not attributed to true changes in the construct to be measured. COSMIN requires smallest detectable change or limits of agreement to be measured to assess the measurement error. As with reliability, it requires multiple observations in time71.
测量误差是指参与者分数的系统性和随机误差,这些误差不归因于待测结构的真实变化。COSMIN 需要测量最小的可检测变化或一致性限值来评估测量误差。与可靠性一样,它需要在时间71 中进行多次观测。
Construct validity 构造有效性
Construct validity refers to the degree to which the scores are consistent with hypotheses based on the assumption that the scale validly measures the intended construct. COSMIN requires a comparison to either another scale aiming to measure a similar construct or hypothesis testing among subgroups71.
结构效度是指分数与基于量表有效测量预期结构的假设的假设一致的程度。COSMIN 需要与另一个旨在测量类似结构的量表或亚组之间的假设检验进行比较71。
Responsiveness 反应
Responsiveness refers to the scale’s ability to detect change over time in the construct to be measured. COSMIN allows several ways to test scale’s responsiveness including hypothesis testing before and after intervention, comparison between subgroups, comparison with other outcome measurement instruments, or comparison to a gold standard71.
响应性是指秤检测待测结构随时间变化的能力。COSMIN 允许多种方法来测试量表的反应性,包括干预前后的假设检验、亚组之间的比较、与其他结果测量工具的比较或与金标准71 的比较。
Interpretability 可解释性
Interpretability refers to the degree to which one can assign qualitative meaning to the scores or changes in scores71. I included an assessment of overall scores’ distributions, missing data, and floor and ceiling effects. Overall scores’ distributions show if the scale results in normally distributed data. Missing data should be minimized to ensure they did not affect the validation procedure. Finally, floor and ceiling effects show whether the extreme items are missing in the lower or upper end of the scale, indicating limited content validity. Consequently, participants with the lowest or highest possible score cannot be distinguished from each other, thus reliability is reduced. I considered floor and ceiling effects to be present if more than 15% of respondents achieved the lowest or highest possible score, respectively72.
可解释性是指一个人可以为分数或分数的变化赋予定性意义的程度71.我包括了对总分分布、缺失数据以及下限和上限效应的评估。总分的分布显示尺度是否产生正态分布的数据。应尽量减少缺失数据,以确保它们不会影响验证过程。最后,下限和上限效果显示极端项目是否在量表的下端或上限缺失,这表明内容有效性有限。因此,无法区分得分最低或最高的参与者,从而降低了可靠性。如果超过 15% 的受访者获得最低或最高可能分数,即72,则我认为存在下限和上限效应。
Feasibility 可行性
Feasibility refers to the ease of application of the scale in its intended context of use, given constraints such as time or money73. I checked the languages in which the scales are available and the scales’ completion times.
可行性是指在时间或金钱等限制条件下,秤在其预期使用环境中的易用性73。我检查了量表可用的语言以及量表的完成时间。
Data availability 数据可用性
All data generated or analysed during this study are included in this published article.
本研究期间生成或分析的所有数据都包含在这篇发表的文章中。
References 引用
Benzakour, A. et al. Artificial intelligence in spine surgery. Int. Orthop. 47, 457–465 (2023).
Benzakour, A. 等人。脊柱手术中的人工智能。国际骨科47, 457–465 (2023 年)。Hamet, P. & Tremblay, J. Artificial intelligence in medicine. Metabolism 69, S36–S40 (2017).
Hamet, P. & Tremblay, J. 医学中的人工智能。代谢69,S36–S40 (2017)。Haug, C. J. & Drazen, J. M. Artificial intelligence and machine learning in clinical medicine. N. Engl. J. Med. 388, 1201–1208 (2023).
Haug, C. J. & Drazen, J. M. 临床医学中的人工智能和机器学习。N. Engl. J. Med.388, 1201–1208 (2023 年)。Kumar, Y. et al. Artificial intelligence in disease diagnosis: a systematic literature review, synthesizing framework and future research agenda. J. Ambient Intell. Humaniz. Comput. 14, 8459–8486 (2023).
Kumar, Y. 等人。人工智能在疾病诊断中的应用:系统文献综述、综合框架和未来研究议程。J. 环境智能。人性化。计算。14, 8459–8486 (2023 年)。Chiu, T. K. F. et al. Systematic literature review on opportunities, challenges, and future research recommendations of artificial intelligence in education. Comput. Educ. 4, 100118 (2022).
Sourati, J. & Evans, J. A. Accelerating science with human-aware artificial intelligence. Nat. Hum. Behav. 7, 1–15 (2023).
Xu, Y. et al. Artificial intelligence: a powerful paradigm for scientific research. The Innovation https://doi.org/10.1016/j.xinn.2021.100179 (2021).
Wang, H. et al. Scientific discovery in the age of artificial intelligence. Nature 620, 47–60 (2023).
Verma, A., Lamsal, K. & Verma, P. An investigation of skill requirements in artificial intelligence and machine learning job advertisements. Ind. High. Educ. 36, 63–73 (2022).
Wilson, H. J., Dougherty, P. R. & Morini-Bianzino, N. The jobs that artificial intelligence will create. MITSloan Manag. Rev. 58, 13–16 (2017).
Alekseeva, L. et al. The demand for AI skills in the labor market. Labour Econ. 71, 102002 (2021).
Acemoglu, D. et al. Artificial intelligence and jobs: evidence from online vacancies. J. Labor Econ. 40, S293–S340 (2022).
Helmus, T. C. Artificial Intelligence, Deepfakes, and Disinformation: A Primer. https://doi.org/10.7249/PEA1043-1 (2022).
Khanjani, Z., Watson, G. & Janeja, V. P. Audio deepfakes: a survey. Front. Big Data 5, 1001063 (2023).
Bray, S. D., Johnson, S. D. & Kleinberg, B. J. Testing human ability to detect ‘deepfake’ images of human faces. Cybersecurity 9, tyad011 (2023).
Köbis, N. C., Doležalová, B. & Soraperra, I. Fooled twice: people cannot detect deepfakes but think they can. Iscience. https://doi.org/10.1016/j.isci.2021.103364 (2021).
Yang, W. Artificial intelligence education for young children: why, what, and how in curriculum design and implementation. Comput. Educ. 3, 100061 (2022).
Ng, D. T. K. et al. Conceptualizing AI literacy: an exploratory review. Comput. Educ. 2, 100041 (2021).
Laupichler, M. C., Aster, A., Schirch, J. & Raupach, T. Artificial intelligence literacy in higher and adult education: a scoping literature review. Comput. Educ. 3, 100101 (2022).
Ng, D. T. K. et al. A review of AI teaching and learning from 2000 to 2020. Educ. Inf. Technol. 28, 8445–8501 (2023).
Su, J., Ng, D. T. K. & Chu, S. K. W. Artificial intelligence (AI) literacy in early childhood education: the challenges and opportunities. Comput. Educ. 4, 100124 (2023).
Casal-Otero, L. et al. AI literacy in K-12: a systematic literature review. Int. J. STEM Educ. 10, 29 (2023).
Ng, D. T. K. et al. Artificial intelligence (AI) literacy education in secondary schools: a review. Interact. Learn. Environ. 31, 1–21 (2023).
Steinbauer, G., Kandlhofer, M., Chklovski, T., Heintz, F. & Koenig, S. A differentiated discussion about AI education K-12. Künstl. Intell. 35, 131–137 (2021).
Hwang, Y., Lee, J. H. & Shin, D. What is prompt literacy? An exploratory study of language learners’ development of new literacy skill using generative AI. https://doi.org/10.48550/arXiv.2311.05373 (2023).
Mertala, P. & Fagerlund, J. Finnish 5th and 6th graders’ misconceptions about artificial intelligence. Int. J. Child. https://doi.org/10.1016/j.ijcci.2023.100630 (2024)
Yau, K. W. et al. Developing an AI literacy test for junior secondary students: The first stage. In 2022 IEEE International Conference on Teaching, Assessment and Learning for Engineering (TALE). https://doi.org/10.1109/TALE54877.2022.00018 (IEEE, 2022).
Li, X. et al. Understanding medical students’ perceptions of and behavioral intentions toward learning artificial intelligence: a survey study. Int. J. Environ. Res. Public Health 19, 8733 (2022).
Su, J. Development and validation of an artificial intelligence literacy assessment for kindergarten children. Educ. Inf. Technol. https://doi.org/10.1007/s10639-024-12611-4 (2024).
Morales-García, W. C., Sairitupa-Sanchez, L. Z., Morales-García, S. B. & Morales-García, M. Adaptation and psychometric properties of a brief version of the general self-efficacy scale for use with artificial intelligence (GSE-6AI) among university students. Front. Educ. 9, 1293437 (2024).
Wang, Y. Y. & Chuang, Y. W. Artificial intelligence self-efficacy: scale development and validation. Educ. Inf. Technol. 28, 1–24 (2023).
Hornberger, M., Bewersdorff, A. & Nerdel, C. What do university students know about Artificial Intelligence? Development and validation of an AI literacy test. Comput. Educ. 5, 100165 (2023).
Zhang, H., Perry, A. & Lee, I. Developing and validating the artificial intelligence literacy concept inventory: an instrument to assess artificial intelligence literacy among middle school students. Int. J. Artif. Intell. Educ. https://doi.org/10.1007/s40593-024-00398-x (2024).
Soto-Sanfiel, M. T., Angulo-Brunet, A. & Lutz, C. The scale of artificial intelligence literacy for all (SAIL4ALL): a tool for assessing knowledge on artificial intelligence in all adult populations and settings. Preprint at arXiv https://osf.io/bvyku/ (2024).
Ng, D. T. K. et al. Design and validation of the AI literacy questionnaire: the affective, behavioural, cognitive and ethical approach. Br. J. Educ. Technol. 54, 1–23 (2023).
Wang, B., Rau, P. L. P. & Yuan, T. Measuring user competence in using artificial intelligence: validity and reliability of artificial intelligence literacy scale. Behav. Inf. Technol. 42, 1324–1337 (2022).
Chan, C. K. Y. & Zhou, W. An expectancy value theory (EVT) based instrument for measuring student perceptions of generative AI. Smart Learn. Environ. 10, 1–22 (2023).
Lee, S. & Park, G. Development and validation of ChatGPT literacy scale. Curr. Psychol. https://doi.org/10.1007/s12144-024-05723-0 (2024).
Hwang, H. S., Zhu, L. C. & Cui, Q. Development and validation of a digital literacy scale in the artificial intelligence era for college students. KSII Trans. Internet Inf. Syst. https://doi.org/10.3837/tiis.2023.08.016 (2023).
Celik, I. Towards Intelligent-TPACK: an empirical study on teachers’ professional knowledge to ethically integrate artificial intelligence (AI)-based tools into education. Comput. Hum. Behav. 138, 107468 (2023).
Kim, S. W. & Lee, Y. The artificial intelligence literacy scale for middle school. Stud. J. Korea Soc. Comput. Inf. 27, 225–238 (2022).
Carolus, A. et al. MAILS—meta AI literacy scale: development and testing of an AI literacy questionnaire based on well-founded competency models and psychological change- and meta-competencies. Comput. Hum. Behav. 1, 100014 (2023).
Karaca, O., Çalışkan, S. A. & Demir, K. Medical artificial intelligence readiness scale for medical students (MAIRS-MS)–development, validity and reliability study. BMC Med. Educ. 21, 1–9 (2021).
Pinski, M. & Benlian, A. AI Literacy-towards measuring human competency in artificial intelligence. In Hawaii International Conference on System Sciences. https://hdl.handle.net/10125/102649 2023.
Laupichler, M. C. et al. Development of the “Scale for the assessment of non-experts’ AI literacy”—ān exploratory factor analysis. Comput. Hum. Behav. Rep. 12, 100338 (2023a).
Çelebi, C. et al. Artificial intelligence literacy: an adaptation study. Instr. Technol. Lifelong Learn 4, 291–306 (2023).
Polatgil, M. & Güler, A. Bilim. Nicel Araştırmalar Derg. 3, 99–114, https://sobinarder.com/index.php/sbd/article/view/65. (2023).
Moodi Ghalibaf, A. et al. Psychometric properties of the persian version of the Medical Artificial Intelligence Readiness Scale for Medical Students (MAIRS-MS). BMC Med. Educ. 23, 577 (2023).
Laupichler, M. C., Aster, A., Perschewski, J. O. & Schleiss, J. Evaluating AI courses: a valid and reliable instrument for assessing artificial-intelligence learning through comparative self-assessment. Educ. Sci. 13, 978 (2023b).
Yilmaz, F. G. K. & Yilmaz, R. Yapay Zekâ Okuryazarlığı Ölçeğinin Türkçeye Uyarlanması. J. Inf. Commun. Technol. 5, 172–190 (2023).
Laupichler, M. C., Aster, A., Meyerheim, M., Raupach, T. & Mergen, M. Medical students’ AI literacy and attitudes towards AI: a cross-sectional two-center study using pre-validated assessment instruments. BMC Med. Educ. 24, 401 (2024).
Mokkink, L. B. et al. COSMIN risk of bias checklist for systematic reviews of patient-reported outcome measures. Qual. Life Res. 27, 1171–1179 (2018).
Prinsen, C. A. et al. COSMIN guideline for systematic reviews of patient-reported outcome measures. Qual. Life Res. 27, 1147–1157 (2018).
Terwee, C. B. et al. COSMIN methodology for evaluating the content validity of patient-reported outcome measures: a Delphi study. Qual. Life Res. 27, 1159–1170 (2018).
Mokkink, L. B. et al. COSMIN Risk of Bias tool to assess the quality of studies on reliability or measurement error of outcome measurement instruments: a Delphi study. BMC Med. Res. Methodol. 20, 1–13 (2020).
Terwee, C. B. et al. Rating the methodological quality in systematic reviews of studies on measurement properties: a scoring system for the COSMIN checklist. Qual. Life Res. 21, 651–657 (2012).
Schünemann, H., Brożek, J., Guyatt, G. & Oxman, A. (Eds.) GRADE Handbook. https://gdt.gradepro.org/app/handbook/handbook.html (2013).
Long, D. & Magerko, B. What is AI literacy? Competencies and design considerations. In Proceedings of the 2020 CHI conference on human factors in computing systems, 1–16. https://doi.org/10.1145/3313831.3376727 (2020).
Ali, S., Payne, B. H., Williams, R., Park, H. W. & Breazeal, C. Constructionism, ethics, and creativity: Developing primary and middle school artificial intelligence education. International Workshop on Education in Artificial Intelligence K-12 (EDUAI’19) 1–4 (2019).
Cassidy, S. & Eachus, P. Developing the computer user self-efficacy (CUSE) scale: Investigating the relationship between computer self-efficacy, gender and experience with computers. J. Educ. Comput. Res. 26, 133–153 (2002).
Compeau, D. R. & Higgins, C. A. Computer self-efficacy: development of a measure and initial test. MIS Q. 19, 189–211 (1995).
Mishra, P. & Koehler, M. J. Technological pedagogical content knowledge: a framework for teacher knowledge. Teach. Coll. Rec. 108, 1017–1054 (2006).
Laupichler, M. C., Aster, A. & Raupach, T. Delphi study for the development and preliminary validation of an item set for the assessment of non-experts’ AI literacy. Comput. Educ. 4, 100126 (2023).
Page, M. J. et al. The PRISMA 2020 statement: an updated guideline for reporting systematic reviews. Int. J. Surg. 88, 105906 (2021).
Aromataris, E. & Riitano, D. Constructing a search strategy and searching for evidence. Am. J. Nurs. 114, 49–56 (2014).
Shoman, Y. et al. Psychometric properties of burnout measures: a systematic review. Epidemiol. Psychiatr. Sci. 30, e8 (2021).
Wittkowski, A., Vatter, S., Muhinyi, A., Garrett, C. & Henderson, M. Measuring bonding or attachment in the parent-infant relationship: a systematic review of parent-report assessment measures, their psychometric properties and clinical utility. Clin. Psychol. Rev. 82, 101906 (2020).
Rahmatpour, P., Nia, H. S. & Peyrovi, H. Evaluation of psychometric properties of scales measuring student academic satisfaction: a systematic review. J. Educ. Health Promot. 8, 256 (2019).
R Core Team. R: A language and environment for statistical computing. (R Foundation for Statistical Computing, Vienna, Austria, 2020). https://www.R-project.org/.
Viechtbauer, W. Conducting meta-analyses in R with the metafor Package. J. Stat. Softw. 36, 1–48 (2010).
Mokkink, L. B. et al. The COSMIN study reached international consensus on taxonomy, terminology, and definitions of measurement properties for health-related patient-reported outcomes. J. Clin. Epidemiol 63, 737–745 (2010).
McHorney, C. A. & Tarlov, A. R. Individual-patient monitoring in clinical practice: are available health status surveys adequate? Qual. Life Res. 4, 293–307 (1995).
Prinsen, C. A. et al. How to select outcome measurement instruments for outcomes included in a “Core Outcome Set”–a practical guideline. Trials 17, 1–10 (2016).
Acknowledgements
This work was supported by the NPO ‘Systemic Risk Institute’ number LX22NPO5101, funded by European Union—Next Generation EU (Ministry of Education, Youth and Sports, NPO: EXCELES).
Author information
Authors and Affiliations
Contributions
T.L. conceived the review, performed the systematic search and scales’ assessments, and wrote and revised this study.
Corresponding author
Ethics declarations
Competing interests
The author declares no competing interests.
Additional information
Publisher’s note Springer Nature remains neutral with regard to jurisdictional claims in published maps and institutional affiliations.
Supplementary information
Rights and permissions
Open Access This article is licensed under a Creative Commons Attribution 4.0 International License, which permits use, sharing, adaptation, distribution and reproduction in any medium or format, as long as you give appropriate credit to the original author(s) and the source, provide a link to the Creative Commons licence, and indicate if changes were made. The images or other third party material in this article are included in the article’s Creative Commons licence, unless indicated otherwise in a credit line to the material. If material is not included in the article’s Creative Commons licence and your intended use is not permitted by statutory regulation or exceeds the permitted use, you will need to obtain permission directly from the copyright holder. To view a copy of this licence, visit http://creativecommons.org/licenses/by/4.0/.