区间 1:1996-2002 (1)
|
区间 2:2004-2010 (2)
|
区间 3:2012-2018 (3)
|
增长:1 至 3(4)
|
||||||||||
Panel A. Variance, in levels 面板 A. 方差,水平 |
|||||||||||||
Total variance 总方差 | 0.794 | 0.862 | 0.915 | 0.121 | |||||||||
Within-firm 企业内部 | 0.512 | 0.532 | 0.531 | 0.018 | |||||||||
Between-firm, within-industry 企业间,行业内 |
0.112 | 0.127 | 0.140 | 0.028 | |||||||||
Between-industry 行业间 | 0.170 | 0.203 | 0.245 | 0.075 | |||||||||
Panel B. Variance, as percent of total 面板 B。方差,占总数的百分比 |
|||||||||||||
Within-firm 企业内部 | 64.6 | 61.7 | 58.0 | 14.9 | |||||||||
Between-firm, within-industry 企业间,行业内 |
14.0 | 14.7 | 15.3 | 23.1 | |||||||||
Between-industry 行业间 | 21.4 | 23.6 | 26.8 | 61.9 | |||||||||
Panel C. Other measures 面板 C. 其他措施 |
|||||||||||||
Sample size (millions) 样本大小(百万) | 239.4 | 249.2 | 269.7 | ||||||||||
Number of firms (thousands) 企业数量(千家) |
470 | 460 | 466 | ||||||||||
Number of NAICS industries 北美行业分类系统(NAICS)行业数量 |
301 | 301 | 301 |
行业间方差增长的行业份额
|
Number of industries 行业数量 | 总就业份额 (%)
|
行业间方差增长的总贡献
|
行业间方差增长的总份额 (%)
|
||||||||||||
5 industries 五个行业 | 8.8 | 0.8 | ||||||||||||||
25 industries 25 个行业 | 30.5 | 0.031 | 40.7 | |||||||||||||
71 industries 71 个行业 | 21.8 | 0.017 | 57.4 | |||||||||||||
145 industries 145 个行业 | 19.3 | -0.000 | 22.3 | |||||||||||||
55 industries 55 个行业 | 19.7 | -0.015 | -0.1 | |||||||||||||
Overall 总体 | 301 industries 301 个行业 | 100.0 | 0.075 | -20.3 |
\hline & & Average
(1) & Change
(2) & Average
(3) & \begin{tabular}{l}
Change \\
(4)
\end{tabular} & \\
\hline 2111 & Oil and Gas Extraction & 0.3 & \(-0.0\) & 1.012 & 0.247 & 1.8 \\
\hline 2131 & Support Activities for Mining & 0.5 & 0.3 & 0.374 & 0.191 & 1.4 \\
\hline 3254 & Pharmaceutical Manufacturing & 0.5 & \(-0.1\) & 0.799 & 0.203 & 1.6 \\
\hline 3344 & Semiconductor Manufacturing & 0.8 & \(-0.5\) & 0.556 & 0.299 & 1.4 \\
\hline 4234 & Professional Equipment Wholesaler & 0.7 & \(-0.0\) & 0.557 & 0.190 & 1.9 \\
\hline 4441 & Building Material and Supplies & 0.9 & 0.1 & \(-0.293\) & \(-0.180\) & 1.5 \\
\hline 4451 & Grocery Stores & 2.4 & 0.0 & \(-0.378\) & \(-0.194\) & 4.7 \\
\hline 4481 & Clothing Stores & 0.7 & \(-0.0\) & \(-0.607\) & \(-0.244\) & 2.6 \\
\hline 4529 & Other General Merchndse. Stores & 1.4 & 1.5 & \(-0.539\) & \(-0.051\) & 6.8 \\
\hline 5112 & Software Publishers & 0.5 & 0.2 & 1.009 & 0.186 & 5.6 \\
\hline 5182 & Data Processing Services & 0.3 & \(-0.0\) & 0.545 & 0.301 & 1.3 \\
\hline 5191 & Other Information Services & 0.2 & 0.3 & 0.798 & 0.699 & 5.8 \\
\hline 5221 & Depository Credit Intermediate. & 2.1 & 0.0 & 0.189 & 0.234 & 2.5 \\
\hline 5231 & Securities Brokerage & 0.5 & \(-0.1\) & 0.866 & 0.204 & 1.1 \\
\hline 5239 & Other Financial Investment Activity & 0.3 & 0.1 & 0.834 & 0.388 & 3.3 \\
\hline 5241 & Insurance Carriers & 1.6 & \(-0.4\) & 0.488 & 0.167 & 2.3 \\
\hline 5413 & Archt. and Engineering Services & 1.2 & 0.1 & 0.469 & 0.161 & 2.6 \\
\hline 5415 & Computer Systems Design & 1.7 & 0.9 & 0.663 & 0.012 & 5.6 \\
\hline 5416 & Mgmt. and Scientific Services & 0.9 & 0.6 & 0.381 & 0.069 & 1.8 \\
\hline 5417 & Scientific Research Services & 0.8 & \(-0.1\) & 0.741 & 0.244 & 3.3 \\
\hline 5511 & Management of Companies & 2.0 & \(-0.1\) & 0.471 & 0.201 & 5.0 \\
\hline 5613 & Employment Services & 3.9 & 0.6 & \(-0.685\) & 0.017 & 2.5 \\
\hline 5617 & Services to Buildings and Dwell & 1.1 & 0.3 & \(-0.493\) & \(-0.002\) & 1.1 \\
\hline 6211 & Offices of Physicians & 1.7 & 0.5 & 0.254 & 0.099 & 1.6 \\
\hline 6216 & Home Health Care Services & 0.8 & 0.4 & \(-0.525\) & \(-0.016\) & 1.7 \\
\hline 6221 & General Medical and Hospitals & 4.5 & 0.5 & 0.205 & 0.162 & 4.2 \\
\hline 6233 & Continuing Care Retirement & 0.6 & 0.4 & \(-0.493\) & \(-0.001\) & 1.2 \\
\hline 6241 & Individual and Family Services & 0.8 & 0.6 & \(-0.490\) & \(-0.155\) & 3.5 \\
\hline 7139 & Othr. Amusement and Recreation & 0.6 & 0.1 & \(-0.594\) & \(-0.106\) & 1.7 \\
\hline 7225 & Restaurants and Othr. Eat Places & 4.9 & 2.0 & \(-0.739\) & \(-0.027\) & 16.9 \\
\hline
\end{tabular}
Notes: Authors' tabulations of LEHD microdata. Tabulations include workers with annual real earnings \(>\$ 3,770\) in EINs with 20 or more employees. Average log earnings for industry \(k\) are relative to the economy average. The 1996-2002 and 2012-2018 intervals are averaged. Changes are the growth (or decline) from 1996-2002 to 2012-2018. See equation (2) for definitions. Top 11 low-paying industries in bold.
transportation and material moving, building and grounds cleaning and maintenance, and food preparation and serving are increasingly employed in industries that provide services to other firms. Important industries in the top 11 low-paying industries that fit this description include Employment Services (5613) and Services to Buildings and Dwellings (5617). As we will see below using the OEWS, we find patterns consistent with the shift of such occupations away from the top 19 high-paying industries.
What about the other 271 four-digit NAICS industries? Figure 1 highlights they make relatively little contribution. Using Table 2, there are 145 industries that each
Table 4-Industry Contributions to Between-Industry Variance Growth, by Average Earnings
\begin{tabular}{|c|c|c|c|c|c|c|}
\hline \multirow[b]{2}{*}{Industry relative earnings} & \multirow[b]{2}{*}{Number of Industries} & \multirow[t]{2}{*}{Total employment share (1)} & \multirow[t]{2}{*}{Total contribution to between-industry growth
(2)} & \multirow[t]{2}{*}{Total share of between-industry growth} & \multicolumn{2}{|l|}{Shift-share} \\
\hline & & & & & \begin{tabular}{l}
Employment \\
(4)
\end{tabular} & Earnings
(5) \\
\hline Overall & 301 & 100.0\% & 0.075 & 100.0\% & \(14.0 \%\) & 86.0\% \\
\hline \multicolumn{7}{|l|}{Panel A. 30 industries with variance contribution \(>1 \%\)} \\
\hline High-paying & 19 & \(21.1 \%\) & 0.041 & \(54.1 \%\) & \(16.1 \%\) & 83.9\% \\
\hline Low-paying & 11 & \(18.1 \%\) & 0.033 & \(44.1 \%\) & \(68.3 \%\) & \(31.7 \%\) \\
\hline \multicolumn{7}{|l|}{Panel B. 271 industries with variance contribution \(\leq 1 \%\)} \\
\hline High-paying & 146 & \(34.9 \%\) & 0.001 & \(1.3 \%\) & & \\
\hline Low-paying & 125 & \(25.9 \%\) & 0.000 & 0.6\% & & \\
\hline
\end{tabular}
Notes: Authors' tabulations of LEHD microdata. Tabulations include workers with annual real earnings \(>\$ 3,770\) in EINs with 20 or more employees. Employment shares are calculated as the average of 1996-2002 and 2012-2018 employment shares. Industry \(k\) 's contribution to between-industry variance growth is specified in equation (2). The shift-share calculations for changing employment and earnings follow equation (3). Shift-share results are summed across industries and normalized by the total contribution so that the two components sum to 100 percent. The two rows for the 271 industries with variance contribution \(\leq 1\) percent have missing cells because the denominator for the shift-share decomposition is close to zero.
contribute approximately 0.0 percent (to be precise, greater than -0.05 percent and less than 0.05 percent) to between-industry variance growth. This says that almost one-half of all four-digit NAICS industries contribute essentially nothing to inequality growth. There are 71 industries that contribute between 0.05 percent and 1.0 percent, accounting for 22.3 percent of between-industry variance growth. These industries are basically offset by another 55 industries that have a negative contribution ( \(<-0.05\) percent), accounting for -20.3 percent of between-industry variance growth.
As seen in Table 4, the top 30 industries include 19 high-paying industries that account for 54.1 percent of between-industry variance growth, and 11 low-paying industries that account for 44.1 percent of between-industry variance growth. The other 271 industries that have small contributing and offsetting contributions to increasing inequality do not occur systematically among high-paying versus low-paying industries. 146 high-paying industries account for 1.3 percent of between-industry variance growth, and 125 low-paying industries account for only 0.6 percent of between-industry variance growth.
Changes in earnings and employment share determine an industry's contribution to growth in inequality. This is seen in the expression defining industry \(k\) 's contribution to between-industry variance growth: \(\Delta\left(\frac{N^{k, p}}{N^{p}}\right)\left(\bar{y}^{k, p}-\bar{y}^{p}\right)^{2}\). If an industry with relatively high earnings exhibits an earnings increase, then, ceteris paribus, inequality will increase. Analogously, inequality will increase if an industry with relatively low earnings exhibits an earnings decrease. In contrast, when average earnings in an industry moves closer to the overall average, inequality decreases.
Employment shares also determine industry-level contributions to inequality. An industry's earnings changes will have larger effects on inequality when its employment share is larger. Changes in an industry's employment share will have smaller effects on inequality when that industry's pay is more similar to the overall average.
Employment gains among very high- and very low-paying industries tend to increase inequality.
In Table 4, we report the relative importance of earnings changes versus employment changes using a shift share decomposition. Industry \(k\) 's contribution to between-industry variance growth is \(\Delta\left(\frac{N^{k, p}}{N^{p}}\right)\left(\bar{y}^{k, p}-\bar{y}^{p}\right)^{2}\). We can use a standard shift-share decomposition to express this change in terms of the components attributable to changes in employment versus earnings:
\[
\underbrace{\Delta\left(\frac{N^{k, p}}{N^{p}}\right)\left(\bar{y}^{k, p}-\bar{y}^{p}\right)^{2}}_{\begin{array}{c}
\text { industry } k^{\prime} \text { s contribution to } \\
\text { between-industry variance growth }
\end{array}}=\underbrace{\overline{\left(\bar{y}^{k, p}-\bar{y}^{p}\right)^{2}} \Delta\left(\frac{N^{k, p}}{N^{p}}\right)}_{\text {shift-share: employment }}+\underbrace{\frac{\overline{N^{k, p}}}{N^{p}} \Delta\left(\bar{y}^{k, p}-\bar{y}^{p}\right)^{2}}_{\text {shift-share: earnings }}
\]
where \(\overline{\left(\bar{y}^{k, p}-\bar{y}^{p}\right)^{2}}\) and \(\overline{\frac{N^{k, p}}{N^{p}}}\) are averages of intervals 1 and 3 . We do this for our top 30 industries, distinguished by high-paying and low-paying industries (we do not present the shift share estimates for the other 271 industries since the denominator of the shift share is very close to zero). Among the 19 high-paying industries, 83.9 percent of between-industry variance growth is accounted for by changing relative earnings, and the remaining 16.1 percent is accounted for by changing employment shares. Among the 11 low-paying industries, the relative importance of earnings versus employment is reversed: 68.3 percent of between-industry variance growth is accounted for by changing employment shares, and the remaining 31.7 percent is accounted for by changing relative earnings. These results highlight different explanations for why between-industry variance growth is increasing at the opposite tails of the earnings distribution. Inequality growth at the top of the earnings distribution is a story of increasing earnings, whereas inequality growth at the bottom of the earnings distribution is a story of increasing employment.
These two different explanations for increasing inequality among low- versus high-paying industries is evident in the earnings and employment changes of the thirty industries listed in Table 3. All of the 19 high-paying industries exhibit earnings increases during our time period. The most rapid growth is found in Other Information Services (5191), which had a 69.9 log point (101.2 percent) increase in relative earnings. \({ }^{17}\) Of the remaining high-paying industries, nine had earnings increases in excess of \(20 \log\) points ( 22.1 percent), six had increases between 10 (10.5 percent) and \(20 \log\) points, and three had increases less than \(10 \log\) points.
Most of the 11 low-paying industries exhibit earnings decreases, yet they are smaller in absolute value than the earnings increases among the high-paying industries. The only low-paying industry with a decline greater in magnitude than 20 \(\log\) points ( 22.1 percent) is Clothing Stores (4481), which had a 24.4 log point ( 27.6 percent) decrease in relative earnings. Of the remaining low-paying industries, four had earnings declines between 10 ( 10.5 percent) and 20 log points, and five had
\footnotetext{
\({ }^{17}\) We convert \(\log\) differentials to proportionate changes using the expression \(e^{x}-1\). For small differences, \(\log\) points are approximately equal to the percentage change.
}
earnings declines between 0 and 10 log points. One industry, Employment Services (5613), exhibited a relatively small increase in earnings.
On the other hand, changes in employment are more important for the 11 low-paying industries than for the 19 high-paying industries. Two low-paying industries in Table 3 stand out: Restaurants and Other Eating Places (7225) had a 2.0 percentage point increase in employment share, and Other General Merchandise Stores (4529) had a 1.5 percentage point increase in employment share. Eight of the other low-paying industries have smaller employment share increases (less than one percentage point), and one industry (Clothing Stores, 4481) had a declining employment share. Among the 19 high-paying industries, none had employment share increases exceeding 1 percentage point, ten had small employment share increases (less than 1 percentage point), and about one-half (9) of the high-paying industries had declining employment shares.
In the analysis that follows, we also use the CPS-LEHD integrated data, the OEWS and LBD data to provide further insights into the role of rising between-industry dispersion. It is worth highlighting that all of these alternative sources provide a similar quantification of the contribution of the top 30 industries listed above to rising between-industry dispersion. While more detail is provided below, the share of the between-industry increase in dispersion from 1996-2002 to 2012-2018 accounted for by the top 30 industries is 98.1 percent in the LEHD data, 105.5 percent in the CPS-LEHD data, 96.2 percent in the OEWS data, and 94 percent in the LBD data.
\section*{IV. Firm and Worker Composition in the Top 30 Industries}
\section*{A. Mega Firms}
Changes in the employment shares and size-earnings premia for mega (10,000+) firms play a critical role in accounting for rising between-industry earnings inequality. Table 5 shows descriptive statistics of employment and earnings in mega firms and non-mega firms in our four industry groups. One immediate result in Table 5 is that employment has shifted over time to the top 30 industries. The employment share of the top 30 industries increased by 8.2 percentage points, with most of this increase ( 6.0 percentage points) among the 11 low-paying industries. The employment share of the other 271 industries analogously declined by 8.2 percentage points, with most of this decline ( 6.8 percentage points) among the 146 high-paying industries.
The substantial increase in the employment share of the top 30 industries is driven by mega firms. This is evident in both Table 5 and Figure 2. Figure 2 shows the change in employment share by detailed size class for each of our four industry groups. \({ }^{18}\) The employment share of the 11 low-paying industries increased in every size class, with mega firms exhibiting the largest increase ( 2.5 percentage points). The 19 high-paying industries had a smaller increase in employment, but most of this increase ( 1.4 of a total of 2.2 percentage points) is accounted for by mega firms. Given the high average relative pay of mega firms in the high-paying
\footnotetext{
\({ }^{18}\) The corresponding employment share levels in the first interval (1996-2002) and in the third interval (2012-2018) are given in online Appendix Figure F1.
}
Table 5-Changes in Employment and Earnings, by Industry Earnings, Mega Firms versus Others
\begin{tabular}{|c|c|c|c|c|c|c|}
\hline \multirow[t]{2}{*}{Industry relative earnings} & \multirow[t]{2}{*}{Number of industries} & \multirow[t]{2}{*}{Firm employment} & \multicolumn{2}{|l|}{Employment share} & \multicolumn{2}{|l|}{Relative earnings} \\
\hline & & & Average
(1) & Change
(2) & \begin{tabular}{l}
Average \\
(3)
\end{tabular} & Change
(4) \\
\hline \multicolumn{7}{|l|}{Panel A. 30 industries with variance contribution \(>1 \%\)} \\
\hline \multirow[t]{3}{*}{High-paying} & 19 industries & Any & \(21.1 \%\) & \(2.2 \%\) & 0.440 & 0.177 \\
\hline & & \(10,000+\) & \(3.8 \%\) & 1.4\% & 0.579 & 0.145 \\
\hline & & <10,000 & 17.3\% & 0.8\% & 0.410 & 0.174 \\
\hline \multirow[t]{3}{*}{Low-paying} & 11 industries & Any & 18.1\% & 6.0\% & -0.586 & -0.069 \\
\hline & & 10,000+ & 4.3\% & 2.5\% & -0.492 & \(-0.125\) \\
\hline & & \(<10,000\) & 13.8\% & \(3.5 \%\) & \(-0.613\) & \(-0.061\) \\
\hline \multicolumn{7}{|l|}{Panel B. 271 industries with variance contribution \(\leq 1 \%\)} \\
\hline \multirow[t]{3}{*}{High-paying} & 146 industries & Any & \(34.9 \%\) & \(-6.8 \%\) & 0.281 & 0.046 \\
\hline & & \(10,000+\) & \(3.9 \%\) & \(-1.2 \%\) & 0.646 & 0.042 \\
\hline & & \(<10,000\) & \(31.0 \%\) & \(-5.7 \%\) & 0.236 & 0.052 \\
\hline \multirow[t]{3}{*}{Low-paying} & 125 industries & Any & 25.9\% & \(-1.3 \%\) & -0.325 & -0.002 \\
\hline & & 10,000+ & 3.3\% & -0.5\% & -0.404 & \(-0.061\) \\
\hline & & <10,000 & 22.6\% & -0.9\% & \(-0.314\) & 0.006 \\
\hline
\end{tabular}
Notes: Authors' tabulations of LEHD microdata. Tabulations include workers with annual real earnings \(>\$ 3,770\) in EINs with 20 or more employees. Averages and changes use the employment shares and earnings from the 1996-2002 and 2012-2018 intervals. Average log earnings are relative to the economy average.
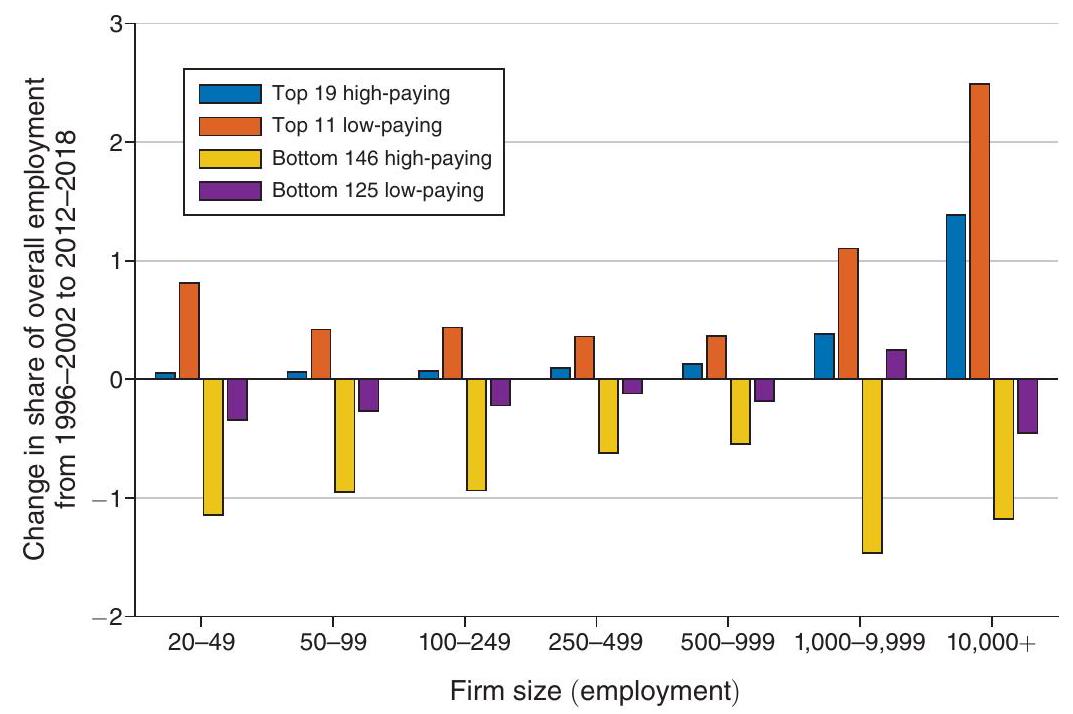
Figure 2. Change in Employment Share by Size Class, by Industry Group
Notes: Authors' tabulations of linked LEHD microdata. Tabulations include workers with annual real earnings \(>\$ 3,770\) in EINs with 20 or more employees. Changes in the employment shares are expressed in terms of percentage points. The denominator is total employment across all size classes and industry groups.
industries ( \(57.9 \log\) points, or 77.9 percent) and the low pay of mega firms ( -49.2 log points, or -63.6 percent) in the low-paying industries, these shifts
in employment to mega firms contributed to rising between-industry earnings inequality. \({ }^{19}\)
Mega firms also play a key role in the changing earnings of the top 30 industries. For the 11 low-paying industries, the relative pay of mega firms decreased by \(12.5 \log\) points ( 13.3 percent) compared to a decline of \(6.1 \log\) points ( 6.3 percent) for the non-mega firms. Both mega firms and non-mega firms in the 19 high-paying industries exhibit large earnings increases: \(14.5 \log\) points ( 15.6 percent) for mega firms and 17.4 log points ( 19.0 percent) for non-mega firms. Earnings at mega firms increased relative to the smallest firms in the top-paying industries but not by as much as the increase in relative earnings at large but not mega firms. \({ }^{20}\) In contrast, relative earnings increases at mega firms in the 146 remaining high-paying industries are modest ( 4.2 log points, or 4.3 percent) compared to \(14.5 \log\) points in the top 19 high-paying industries. Similarly, relative earnings declines at the mega firms in the remaining 125 low-paying industries are modest ( -6.1 log points, or -6.3 percent) compared to the \(-12.5 \log\) points in the top 11 low-paying industries.
\section*{B. Education and Occupation}
To shed light on the changing education composition of the top and bottom industries, we turn to the CPS-LEHD integrated data. Figure 3 shows the change in employment in the top 30 industries from 1996-2002 to 2012-2018. Both low- and high-paying industries had increases in the educational attainment of the workers that they employ. These changes were much more dramatic in the top 19 high-paying industries. The share of workers with bachelor's degrees at these high-paying industries increased by 7.0 percent, workers with advanced degree increased by 8.4 percent, and workers with a high school diploma declined by 8.7 percent. 21
For occupation, we turn to the OEWS published data. \({ }^{22}\) In Figure 4, we consider employment changes across all 22 occupation groups in the top 30 industries. The occupation groups are ranked from left to right by the changes in employment shares in the top 30 industries. There are substantial differences in the changing mix of occupations across the top 19 and bottom 11. The top 19 industries have large increases in Business and Financial Operations (13) and Computer and Mathematical Science (15) with accompanying large declines in Office and
\footnotetext{
\({ }^{19}\) Online Appendix Table H2 shows that the patterns of changes in employment shares by mega firms is robust to using the national and enterprise concepts available in the Business Dynamic Statistics (BDS). The BDS is derived from the LBD.
\({ }^{20}\) Online Appendix Figure F3 shows the cross-sectional size-earnings premia for the 1996-2002 and the 2012-2018 intervals. Among the top 19 high-paying industries, the size-earnings profile shifts upward, with increases in all size classes. Online Appendix F also provides more details of the changing patterns by firm size.
\({ }^{21}\) Online Appendix Figure D1 shows the distribution of employment by education group in the top 19 high-paying and 11 low-paying industries for the 1996-2002 and 2012-2018 periods. In the initial period, the top 19 high-paying industries had significantly higher shares of workers with a bachelor's or advanced degree while the top 11 low-paying industries had higher shares of workers with only a high school diploma or less. These differences became much more pronounced by 2012-2018 with sharp increases in the share of workers with bachelor's and advanced degrees in the top 19 high-paying industries and accompanying declines in the share of workers with a high school diploma or less.
\({ }^{22}\) Online Appendix Figure G1 shows that the CPS-LEHD yields similar patterns on changes in occupations by industry.
}
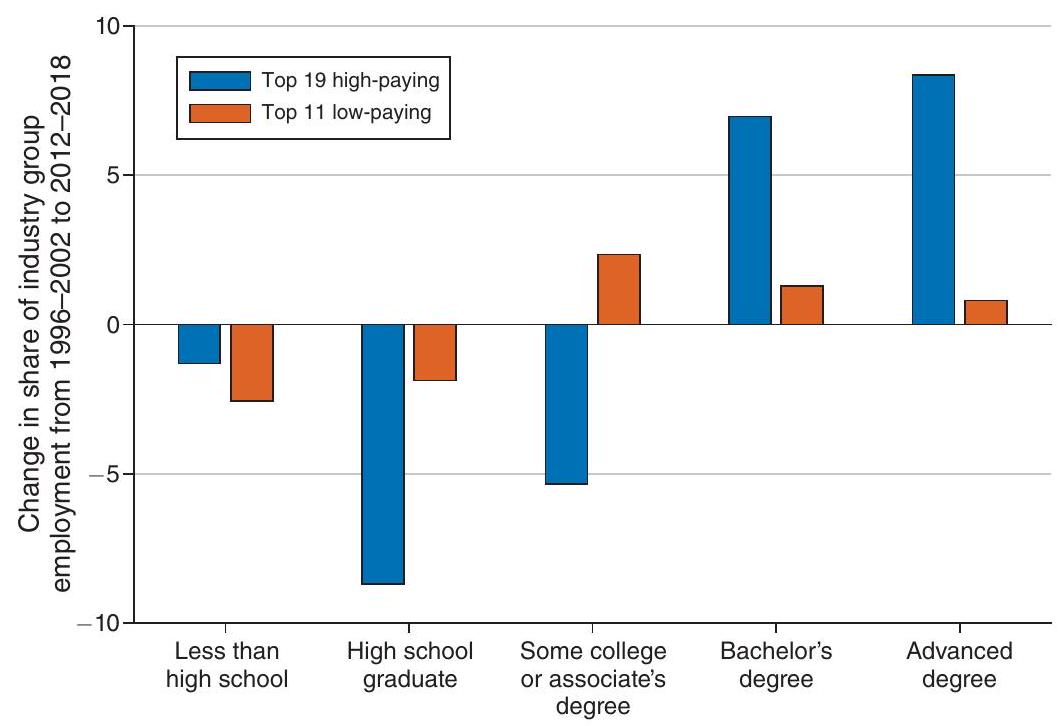
Figure 3. Change in Employment Share by Educational Attainment, by Industry Group
Notes: Authors' tabulations of linked CPS-LEHD microdata. Tabulations include workers with annual real earnings \(>\$ 3,770\). Changes in the employment shares are expressed in terms of percentage points. The denominator is total employment in the respective industry group.
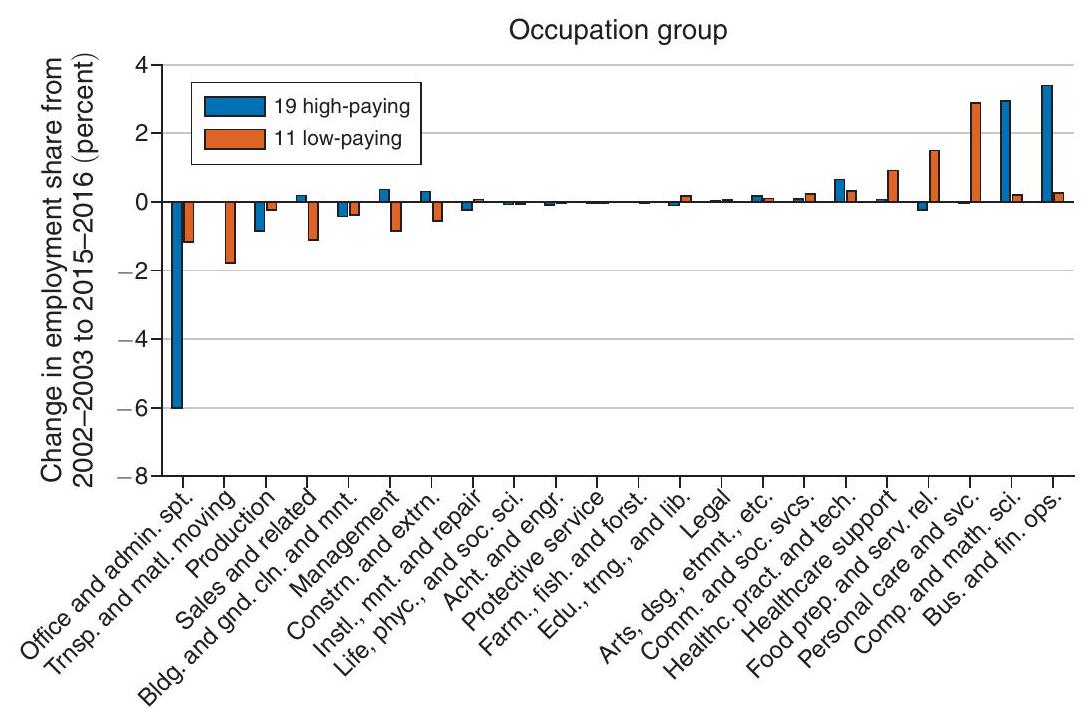
Figure 4. Change in Employment by Occupation and Industry Group
Note: Authors' calculations of published OEWS aggregates.
Administrative Support (43), a low wage occupation group. These patterns are consistent with the dramatic innovations in information and communication technology largely developed by top-paying industries. Related, much of the role of Office and Administrative Support (43) tasks in these high-paying industries are
Table 6-Changes in Employment and EarningS, by Industry EarningS, by Occupation Groups
\begin{tabular}{|c|c|c|c|c|c|}
\hline \multirow[b]{2}{*}{Industry group earnings} & \multirow[b]{2}{*}{Occupation group earnings} & \multicolumn{2}{|l|}{Employment share(\%)} & \multicolumn{2}{|l|}{Relative earnings} \\
\hline & & Average
(1) & Change
(2) & \begin{tabular}{l}
Average \\
(3)
\end{tabular} & Change
(4) \\
\hline \multicolumn{6}{|l|}{Panel A. 30 industries with variance contribution \(>1 \%\)} \\
\hline \multirow[t]{3}{*}{19 high-paying} & All & 16.3 & 1.3 & 0.405 & 0.107 \\
\hline & 12 high-paying & 9.7 & 2.0 & 0.707 & 0.067 \\
\hline & 10 low-paying & 6.6 & \(-0.7\) & \(-0.039\) & 0.029 \\
\hline \multirow[t]{3}{*}{11 low-paying} & All & 19.8 & 3.1 & \(-0.437\) & \(-0.010\) \\
\hline & 12 high-paying & 2.1 & 0.3 & 0.269 & 0.031 \\
\hline & 10 low-paying & 17.7 & 2.8 & \(-0.521\) & \(-0.013\) \\
\hline \multicolumn{6}{|l|}{Panel B. 251 industries with variance contribution \(\leq 1 \%\)} \\
\hline \multirow[t]{3}{*}{141 high-paying} & All & 30.0 & \(-2.8\) & 0.213 & 0.001 \\
\hline & 12 high-paying & 13.0 & \(-0.1\) & 0.496 & 0.005 \\
\hline & 10 low-paying & 17.1 & \(-2.7\) & \(-0.002\) & \(-0.036\) \\
\hline \multirow[t]{3}{*}{110 low-paying} & All & 33.9 & \(-1.6\) & \(-0.130\) & \(-0.011\) \\
\hline & 12 high-paying & 12.8 & \(-0.2\) & 0.238 & \(-0.017\) \\
\hline & 10 low-paying & 21.1 & \(-1.5\) & \(-0.353\) & \(-0.020\) \\
\hline
\end{tabular}
Notes: Authors' calculations of OEWS published aggregates. Changes in employment and earnings compare year intervals 2002-2003 with 2015-2016. Industry pay designations and contributions to variance growth follow the LEHD administrative records data.
now increasingly accomplished with adoption of information and communication technologies. Some of the reduction in Office and Administrative Support (43) may also reflect outsourcing of these tasks to service firms. Consistent with the latter, there have also been decreases in production and building and ground maintenance and cleaning. The employment share of the bottom 11 industries increases, with particularly strong growth in Food Preparation and Serving Related (35) and Personal Care and Service (39). These low-paying industries also exhibit a nontrivial decline in Management (11) occupations.
To get a sense of the contribution of occupations to industry-level wage inequality, we divide occupations into 2 broad categories, 12 that are high-paying relative to the overall average, and ten that are analogously low-paying (see Table 6). Occupational shifts appear to occur, at least broadly, at the industry level. Neanly all of the growth in the employment share of high-paying occupations occurs in the 19 high-paying industries, and all of the growth in low-paying occupations occurs in our 11 low-paying industries. Our 19 high-paying industries increase their employment share by 1.3 percent in the OEWS data. This reflects strong growth among our 12 high-paying occupations, whose share of employment grows by 2.0 percent. The employment share of our 10 low-paying occupations in these 19 high-paying industries contracts by 0.7 percent. The 11 low-paying industries increase their employment share by 3.1 percent. This mostly reflects an increase among our 10 low-paying occupations, whose share of overall employment increases by 2.8 percentage points.
Outside the thirty industries that contribute to inequality, low-paying occupations have a declining employment share. The employment share of our other 141 high-paying industries declines by 2.8 percentage points, and nearly all of this (2.7 percentage points) occurs among low-paying occupations. The employment
share of the 110 low-paying industries declines by 1.6 percentage points, which almost matches ( 1.5 percentage points) its decline in the share of low-paying occupations.
Table 6 also illustrates the role of industry-occupation pay differentials in rising inequality. Our 19 high-paying industries have a strong ( 40.5 log point) earnings differential. This reflects an even larger ( 70.7 log point) differential among our 12 high-paying occupations in these industries. These industries also had the highest earnings growth, both overall ( 10.7 log points), and especially among high-paying occupations ( 6.7 log points). Workers in our 10 low-paying occupations in these 19 high-paying industries had earnings gain as well ( 2.9 log points).
Earnings changed by relatively less among our top 11 low-paying industries. The overall change in earnings was a decline of only \(1.0 \log\) points. This reflects a gain in earnings of 3.1 log points among the relatively rate high-paying occupations. Low-paying occupations in these industries had an earnings decline of 1.3 log points. There were smaller changes among the other 251 industries (an increase of 0.1 log points among high-paying industries, and a decline of 1.1 log points among low-paying industries), with consistent declines in the earnings of low-paying occupations (of 3.6 log points, and 2.0 log points, respectively). \({ }^{23}\)
\section*{V. Inequality in Terms of Sorting and Pay Premia}
In this section, we present decompositions of earnings in the LEHD data using the AKM approach, in the CPS-LEHD data using a human capital approach, and in the OEWS data using a related but distinct approach given the limitation of only having occupation by industry data.
\section*{A. Sorting and Industry Premia Using AKM}
To understand the role of workers and firms in the generation of earnings inequality, we begin by using the linear model of AKM. We estimate our model separately for each of three seven-year intervals: 1996-2002, 2004-2010, and 2012-2018. Following Song et al. (2019), we assume that earnings \(y_{t}^{i, j, k, p}\) are the sum of the effect \(\theta^{i, p}\) of worker \(i\) in interval \(p\), a firm effect \(\psi^{j, k, p}\) when employed by employer \(j\) in industry \(k\) during interval \(p\), and a vector of time-varying observable characteristics \(X_{t}^{i, p}\) for worker \(i\) at time \(t\), which have distinct marginal effects \(\beta^{p}\) by interval \(p\). We express this as
\[
y_{t}^{i, j, k, p}=X_{t}^{i, p} \beta^{p}+\theta^{i, p}+\psi^{j, k, p}+\varepsilon_{t}^{i, j, k, p} .
\]
Our observable characteristics include a set of year dummies that capture calendar year effects on earnings. Following Card, Cardoso, and Kline (2016), we center age around 40 , include a quadratic and cubic transformation of worker age, and omit the
\footnotetext{
\({ }^{23}\) One potential issue is whether greater occupational detail may change the inferences in this section. However, there is a high degree of concentration of occupations across detailed industries. Online Appendix Figure G3 shows that for the two-digit occupations we use in the current analysis, occupations are highly concentrated in detailed industries. Online Appendix Figure G4 shows that using six-digit occupations, the median occupation has a top 20 -industry concentration ratio of 100 percent.
}
linear term. To solve this model, we implement the iterative method proposed by Guimarães and Portugal (2010).
The AKM approach to decomposing earnings has received substantial scrutiny in terms of the interpretation of the estimated person and firm effects. Recent research has highlighted the potential for limited mobility bias arising from the small number of transitions per firm. Bonhomme et al. (2022) find that limited mobility bias yields an upward bias in the variance of firm effects and a downward bias in the covariance between firm and worker effects. However, they find little bias on the contribution of the change in the role of premia and sorting for the change in inequality (and it is the latter that is the focus of our work). \({ }^{24}\) Our use of AKM makes our results directly comparable to Song et al. (2019) which enables us to highlight that the between-firm effects they identified are mostly occurring at the industry level. However, we also include (see Section VB) alternative decompositions based on the CPS-LEHD and OEWS data.
We use the AKM approach to decompose the between-firm components into those that occur within- and between-industries. \({ }^{25}\) To explore cross-industry differences, we calculate industry-level averages. For a given interval \(p\) (and hereafter omitting this superscript), we define the average worker effect in industry \(k\) as \(\bar{\theta}^{k}\), the average effect of observable characteristics as \(\bar{X}^{k} \beta\), and the average firm effect as \(\bar{\psi}^{k}\). Given this notation, it is possible to distinguish between how firm-level pay premia relate to within- versus between-industry earnings dispersion. This is given by
(5) \(\operatorname{var}\left[y_{t}^{i, j, k}\right]\)
\[
\begin{aligned}
& =\underbrace{\operatorname{var}\left[\theta^{i}-\bar{\theta}^{j, k}\right]+\operatorname{var}\left[X_{t}^{i} \beta-\bar{X}^{j, k} \beta\right]+2 \operatorname{cov}\left[\theta^{i}-\bar{\theta}^{j, k}, X_{t}^{i} \beta-\bar{X}^{j, k} \beta\right]}_{\text {within-firm person effect and observables }} \\
& +\underbrace{\operatorname{var}\left[\bar{\psi}^{k}\right]}_{\begin{array}{c}
\text { between-industry } \\
\text { pay premia }
\end{array}}+\underbrace{\operatorname{var}\left[\psi^{j, k}-\bar{\psi}^{k}\right]}_{\begin{array}{c}
\text { within-industry, } \\
\text { between-firm } \\
\text { pay premia }
\end{array}}+\underbrace{2 \operatorname{cov}\left[\bar{\theta}^{k}, \bar{\psi}^{k}\right]+2 \operatorname{cov}\left[\bar{\psi}^{k}, \bar{X}^{k} \beta\right]}_{\text {between-industry covariance }} \\
& +\underbrace{2 \operatorname{cov}\left[\left(\bar{\theta}^{j, k}-\bar{\theta}^{k}\right),\left(\psi^{j, k}-\bar{\psi}^{k}\right)\right]+2 \operatorname{cov}\left[\left(\psi^{j, k}-\bar{\psi}^{k}\right),\left(\bar{X}^{j, k} \beta-\bar{X}^{k} \beta\right)\right]}_{\text {within-industry, between-firm covariance }} \\
& +\underbrace{\operatorname{var}\left[\bar{\theta}^{j, k}-\bar{\theta}^{k}\right]+\operatorname{var}\left[\bar{X}^{j, k} \beta-\bar{X}^{k} \beta\right]+2 \operatorname{cov}\left[\left(\bar{\theta}^{j, k}-\bar{\theta}^{k}\right),\left(\bar{X}^{j, k} \beta-\bar{X}^{k} \beta\right)\right.}_{\text {within-industry, between-firm segregation }}] \\
& +\underbrace{\operatorname{var}\left[\bar{\theta}^{k}\right]+\operatorname{var}\left[\bar{X}^{k} \beta\right]+2 \operatorname{cov}\left[\bar{\theta}^{k}, \bar{X}^{k} \beta\right]}_{\text {between-industry segregation }}+\underbrace{\operatorname{var}\left[\varepsilon_{t}^{i, j, k}\right]}_{\text {residual (within-firm) }} .
\end{aligned}
\]
\footnotetext{
\({ }^{24}\) There has also been concern raised about exogenous mobility. Bonhomme et al. (2022) have highlighted that this is less of an issue than limited mobility bias. The reason is that what is required is that mobility is unrelated to the residual from the AKM model after controlling for person and firm effects.
\({ }^{25}\) Our between-industry decomposition builds on Song et al. (2019). In online Appendix E, we show the original Song et al. (2019) decomposition and compare our results to theirs.
}
Within-firm dispersion is given by the collection of terms in the first line of equation (5). Worker-level effects are given by
\[
\operatorname{var}\left[\theta^{i}-\bar{\theta}^{j, k}\right]+\operatorname{var}\left[X_{t}^{i} \beta-\bar{X}^{j, k} \beta\right]+2 \operatorname{cov}\left[\theta^{i}-\bar{\theta}^{j, k}, X_{t}^{i} \beta-\bar{X}^{j, k} \beta\right]
\]
Residual dispersion \(\operatorname{var}\left[\varepsilon_{t}^{i, j, k}\right]\) occurs within firms given the inclusion of firm effects.
The firm-level premia contributions can be decomposed into withinand between-industry components. Specifically, \(\quad \operatorname{var}\left[\psi^{j, k}\right]=\operatorname{var}\left[\bar{\psi}^{k}\right]+\) \(\operatorname{var}\left[\psi^{j, k}-\bar{\psi}^{k}\right]\), where \(\operatorname{var}\left[\bar{\psi}^{k}\right]\) reflects the between-industry dispersion in average firm effects, that is, industry-level pay premia. The remaining term \(\operatorname{var}\left[\psi^{j, k}-\bar{\psi}^{k}\right]\) captures the within-industry dispersion of firm-level pay premia.
In addition to pay premia, we can distinguish the within- versus between-industry components of a covariance contribution and segregation. The between-industry covariance contribution is defined as \(2 \operatorname{cov}\left[\bar{\theta}^{k}, \bar{\psi}^{k}\right]+2 \operatorname{cov}\left[\bar{\psi}^{k}, \bar{X}^{k} \beta\right]\), which reflects the extent to which highly paid workers are employed in industries with a high pay premium (and vice-versa). This is distinct from the within-industry covariance contribution \(2 \operatorname{cov}\left[\left(\bar{\theta}^{j, k}-\bar{\theta}^{k}\right),\left(\theta^{j, k}-\bar{\theta}^{k}\right)\right]+2 \operatorname{cov}\left[\left(\theta^{j, k}-\bar{\theta}^{k}\right),\left(\bar{X}^{j, k} \beta-\bar{X}^{k} \beta\right)\right]\). This is the component of the covariance contribution where relatively highly paid workers tend to work at high-paying firms, apart from industry-level differences. For example, workers and firms in the Restaurants and Other Eating Places (7225) industry may tend to have low worker effects, while those among Software Publishers (5112) may have high effects. The between-industry component reflects these industry level differences. The within-industry component reflects the extent to which relatively low- versus high-paid workers work for relatively low- versus high-paying firms in those industries.
Segregation also can be decomposed into its within- versus between-industry components. Between-industry segregation is given by industry-level average worker effects. Formally, this is expressed as \(\operatorname{var}\left[\bar{\theta}^{k}\right]+\operatorname{var}\left[\bar{X}^{k} \beta\right]+2 \operatorname{cov}\left[\bar{\theta}^{k}, \bar{X}^{k} \beta\right]\). This is the extent to which low- versus highly paid workers tend to work with each other. To continue with the previous example, Restaurants and Other Eating Places (7225) may employ workers with a low person effect, on average, while employers among Software Publishers (5112) may employ workers with a high average person effect. The extent to which this is related to the firm-level pay differences reflects the covariance component. The extent to which it reflects similar workers grouped together is segregation. Segregation that occurs within industries is expressed as \(\operatorname{var}\left[\bar{\theta}^{j, k}-\bar{\theta}^{k}\right]+\operatorname{var}\left[\bar{X}^{j, k} \beta-\bar{X}^{k} \beta\right]+2 \operatorname{cov}\left[\left(\bar{\theta}^{j, k}-\bar{\theta}^{k}\right),\left(\bar{X}^{j, k} \beta-\bar{X}^{k} \beta\right)\right]\).
While the covariance and segregation components are distinct in the above decomposition and potentially conceptually, in practice we find that the covariance and segregation components of the between industry increase in earnings inequality are closely related. For the complete set of four-digit NAICS industries, the correlation of the changes in the two components is 0.93 and for the top 30 industries it is 0.90 . This tight relationship reflects our finding that high-wage workers work increasingly in high wage industries and work together while low-wage workers work increasingly in low wage industries and work together. Given this tight relationship, we combine these covariance and segregation components into a combined sorting contribution and in turn for expositional convenience primarily focus our
Table 7-Industry-Enhanced Variance Decomposition
\begin{tabular}{lcccc}
\hline \hline & Interval 1: & Interval 2: & Interval 3: & Growth: \\
& \(1996-2002\) & \(2004-2010\) & \(2012-2018\) & 1 to 3 \\
& \((1)\) & \((2)\) & \((3)\) & \((4)\) \\
\hline Panel A. Variance, in levels & & & & \\
Total variance & 0.794 & 0.862 & 0.915 & 0.121 \\
Within-firm & 0.512 & 0.532 & 0.531 & 0.018 \\
Person effect and observables & 0.382 & 0.401 & 0.405 & 0.023 \\
\(\quad\) Residual & 0.130 & 0.131 & 0.125 & -0.005 \\
Between-firm, within-industry & 0.112 & 0.127 & 0.140 & 0.028 \\
\(\quad\) Firm sorting & 0.087 & 0.098 & 0.111 & 0.025 \\
\(\quad\) Firm pay premium & 0.025 & 0.029 & 0.028 & 0.004 \\
Between-industry & 0.170 & 0.203 & 0.245 & 0.075 \\
\(\quad\) Industry sorting & 0.137 & 0.162 & 0.201 & 0.065 \\
\(\quad\) Industry pay premium & 0.033 & 0.042 & 0.044 & 0.011 \\
Panel B. Variance, as percent of total & & & & \\
Within-firm & 64.6 & 61.7 & 58.0 & 14.9 \\
\(\quad\) Person effect and observables & 48.2 & 46.5 & 44.3 & 18.8 \\
\(\quad\) Residual & 16.4 & 15.2 & 13.7 & -3.9 \\
Between-firm, within-industry & 14.0 & 14.7 & 15.3 & 23.1 \\
\(\quad\) Firm sorting & 10.9 & 11.3 & 12.2 & 20.3 \\
\(\quad\) Firm pay premium & 3.1 & 3.4 & 3.1 & 2.9 \\
Between-industry & 21.4 & 23.6 & 26.8 & 61.9 \\
Industry sorting & 17.2 & 18.8 & 22.0 & 53.2 \\
Industry pay premium & 4.2 & 4.8 & 4.8 & 8.7 \\
\hline
\end{tabular}
Notes: Authors' tabulations of LEHD microdata. Tabulations include workers with annual real earnings \(>\$ 3,770\) in EINs with 20 or more employees. See equation (5) and discussion in text for definitions.
discussion on this combined contribution in the remainder of the paper. \({ }^{26}\) In addition, while often expositionally convenient to combine these terms, it is useful to emphasize the especially large contribution of the covariance component of what we denote as sorting to rising inequality. This covariance contribution highlights that rising dispersion in between industry premia contributes directly and indirectly via this rising covariance. We draw this point out in various places in the main text.
Table 7 exploits our industry-enhanced AKM decomposition to understand rising earnings inequality. \({ }^{27}\) The first three columns of Table 7 show results for our three intervals while the last column computes the terms underlying the change in inequality from our first to last intervals (1996-2002 to 2012-2018). 28
Between-industry dispersion accounts for 61.9 percent of the growth in inequality over the time period covered by our dataset. 53.2 percent of the total rise in
\footnotetext{
\({ }^{26}\) In the online Appendix (Appendix Table A4), we provide the separate contribution of these components. In using sorting for the combined contribution of the covariance and segregation components, we deviate from the labels used by Song et al. (2019) who denoted the covariance component sorting. We have a further discussion of the alternative decompositions and terminology in the literature in the online Appendix.
\({ }^{27}\) Online Appendix Table A1 presents estimates that use the Song et. al (2019) approach without reference to industry, and online Appendix Table A2 aggregates these estimates into firm-level segregation, pay premium, and covariance contributions. Online Appendix Table A3 presents estimates of equation (5) before aggregating them as done in Table 7 .
\({ }^{28}\) While their focus is on inequality in the cross section rather than its change over time, Card, Rothstein, and Yi (2024) demonstrate that there is substantial variation in industry premia not explained by worker sorting, which is consistent with our findings in Table 7.
}
inequality can be attributed to rising between-industry sorting. Rising dispersion in industry-level pay premia accounts directly for a smaller but still substantial 8.7 percent of the total rise in inequality in addition to its indirect contribution via sorting.
What firm-level inequality is left after we account for industry-level differences? Table 7 shows that, in the cross-section, less than one-sixth ( 14.0 percent to 15.3 percent) of earnings dispersion occurs between firms in the same industry. Looking at growth, we find that 23.1 percent of variance growth is between firms, within industries. Of this, sorting accounts for 20.2 percent. Rising within-industry, between-firm pay premia play a smaller role in rising inequality accounting for 2.9 percent of the increase in inequality. \({ }^{29}\)
Table 7 also describes within-firm inequality. In the cross section, most of the variation in earnings is within-firm rather than between-firm-but notably the share is declining from 64.6 percent in the first interval to 58.0 percent in the last. Although its share of overall earnings dispersion falls over time, rising within-firm inequality accounts for a modest amount ( 14.9 percent) of the growth in inequality. This mostly reflects an increase in the dispersion of worker effects (18.8 percent), as the residual has a relatively small role offsetting inequality growth ( -3.9 percent). \({ }^{30}\)
We next turn to two alternative methods of analyzing the critical role of industry in rising inequality in recent decades. These methods allow us to assess the robustness of our AKM approach and also provide detail on the mechanisms by which worker characteristics may lead to earnings dispersion.
\section*{B. Sorting and Industry Premia: Alternative Approaches}
We use the CPS-LEHD and OEWS to decompose rising inequality into related but distinct sorting and industry premia contributions. Details are in the online Appendix but we summarize the results here. \({ }^{31}\) Starting with the CPS-LEHD data, we estimate the contributions of age by education, occupation, and industry using the specification from Hoffman, Lee, and Lemieux (2020). This allows us to decompose the variance of earnings into the contribution of within-industry dispersion arising from observable (age by education and occupation) differences in earnings for workers in the same industry, between-industry pay premia, and between-industry sorting. The latter reflects the contribution of the covariance between average industry worker observable effects and industry premia along with the segregation of observable worker effects between industries.
Using the OEWS, we do not have person-level information but we can estimate a related decomposition using the industry by occupation by time interval data. First, we estimate a model in which each occupation has an additive effect on earnings, as does each industry. We then decompose the variance of earnings into within-industry occupation effects (and a within-industry residual), between-industry premia, and
\footnotetext{
\({ }^{29}\) Details about industry contributions to the between-firm, within-industry contributions are provided in online Appendix C.
\({ }^{30}\) These estimates are quite close to analogous results reported by Song et al. (2019) for a similar time period. We elaborate on this in online Appendix E.
\({ }^{31}\) The CPS-LEHD decomposition is described in online Appendix D and that of the OEWS is in online Appendix G.
}
Table 8-Variance Decomposition: AKM versus Human Capital versus Occupation
\begin{tabular}{lcccc}
\hline \hline Data source: \\
Specification: & \begin{tabular}{c}
LEHD \\
AKM \\
\((1)\)
\end{tabular} & \begin{tabular}{c}
CPS-LEHD \\
AKM \\
\((2)\)
\end{tabular} & \begin{tabular}{c}
CPS-LEHD \\
Human capital \\
\((3)\)
\end{tabular} & \begin{tabular}{c}
OEWS \\
Occupation \\
\((4)\)
\end{tabular} \\
\hline Variance, as percent of total \((\%)\) & & & & \\
Between-industry growth & 61.9 & 66.2 & 66.2 & 87.8 \\
\(\quad\) Sorting & 53.2 & 56.5 & 44.8 & 85.0 \\
\(\quad\) Pay premia & 8.7 & 9.6 & 21.4 & 2.8 \\
\hline
\end{tabular}
Notes: The first column is taken from Table 7. The second and third columns are from the linked CPS-LEHD dataset, including workers with annual real earnings \(>\$ 3,770\), see discussion in text and online Appendix equation (D2) for definitions. The fourth column is from the OEWS dataset, see discussion in text and equation (G2) for definitions. In the first 3 columns total variance growth is measured at the person level. In the OEWS, total variance growth is measured at the industry-occupation level.
between-industry sorting (reflecting the covariance between industry premia and average industry occupation effects and the segregation of occupation effects across industries). \({ }^{32}\)
Table 8 compares the decomposition of the contribution of industry using the full LEHD with AKM as in Table 7 with the linked CPS-LEHD analysis using the human capital equation approach. In taking advantage of the data infrastructure from both approaches, we can also integrate the AKM estimates from the full LEHD analysis into the linked CPS-LEHD. Also, included in the table is the decomposition using the OEWS data.
In comparing the columns 1 and 2 in Table 8 we find that the overall contribution of industry is very similar in the full LEHD and the linked CPS-LEHD. The same is true of the respective contributions of industry premia and sorting using AKM estimates. This finding is reassuring as the CPS-LEHD linked data replicates the core patterns from the LEHD. It also highlights that the firm size restriction, imposed in column 1 but not in column 2, has a limited impact.
In comparing columns 2 and 3 in Table 8, we find that, whether using AKM or human capital equation based estimates, the contributions of industry premia and sorting are broadly similar. By construction, the overall contribution of industry is the same in columns 2 and 3 . Industry premia appear to be especially important when we use the human capital approach. In contrast, when we use AKM, between-industry sorting becomes more important. The AKM approach attributes more of inter-industry earnings differentials to time-invariant worker characteristics, some of which are not captured by our human capital framework. \({ }^{33}\)
The last column in Table 8 presents the results using the OEWS. The overall magnitudes of the change in inequality are not comparable to those from the first three columns given that this reflects the changing between-industry variance from industry by occupation data rather than from person-level data. However, it is still striking that the overwhelming fraction (87.8 percent) of the increase in dispersion
\footnotetext{
\({ }^{32}\) See online Appendix G for details and formulas.
\({ }^{33}\) The covariance components from AKM versus the human capital approach are almost identical. It is the segregation component that is smaller with the human capital approach reflecting the smaller role of observable human capital variables compared to worker effects in AKM.
}
Table 9—Sources of Between-Industry Variance Growth: Top 30 Industries
\begin{tabular}{|c|c|c|c|}
\hline \multirow[b]{3}{*}{Industry group} & \multirow[b]{3}{*}{Total contribution to between-industry variance growth} & \multicolumn{2}{|l|}{Share of contribution explained by between-industry (\%)} \\
\hline & & Sorting & Premium \\
\hline & & (2) & (3) \\
\hline \multicolumn{4}{|l|}{Panel A. LEHD AKM decomposition} \\
\hline Top 19 high-paying & 0.041 & 86.2 & 13.8 \\
\hline Top 11 low-paying & 0.033 & 84.2 & 15.8 \\
\hline \multicolumn{4}{|l|}{Panel B. CPS-LEHD human capital decomposition} \\
\hline Top 19 high-paying & 0.039 & 63.5 & 36.5 \\
\hline Top 11 low-paying & 0.030 & 66.1 & 33.9 \\
\hline \multicolumn{4}{|l|}{Panel C. OEWS occupation-industry decomposition} \\
\hline Top 19 high-paying & 0.016 & 77.3 & 22.7 \\
\hline Top 11 low-paying & 0.008 & 81.8 & 18.2 \\
\hline
\end{tabular}
Notes: "LEHD AKM decomposition:" Authors' tabulations of LEHD microdata. Tabulations include workers with annual real earnings \(>\$ 3,770\) in EINs with 20 or more employees. See discussion in text and equation (5) for definitions. "CPS-LEHD human capital decomposition:" Authors' tabulations of linked CPS-LEHD dataset including workers with annual real earnings \(>\$ 3,770\), see discussion in text and equation (D2) for definitions. "OEWS occupation-industry decomposition:" Authors' tabulation of OEWS published aggregates, 281 four-digit industries and 22 occupations. See discussion in text and equation (G2) for definitions.
in earnings in the published OEWS data derives from increasing between-industry dispersion. Furthermore, the between-industry contribution is dominated by sorting (high wage workers based on occupation increasingly sorting into high wage industries and working together and low wage workers increasingly sorting into low wage industries and working together).
\section*{C. Sorting and Industry Premia in the Top 30 Industries}
We now focus our attention on the top 30 industries that contribute to rising between-industry inequality taking advantage of the decompositions from the LEHD, CPS-LEHD, and OEWS data. Table 9 presents the between-industry sorting and firm pay premia contributions from these sources for the top 30 industries. Both of these components contribute substantially to rising between-industry dispersion. However, it is apparent that sorting dominates. However, industry premia contribute directly and also via the covariance component of sorting. \({ }^{34}\) In interpreting Table 9, it is useful to recall the dominance of the top 30 industries in accounting for rising between industry dispersion from the alternative data sources and decompositions. The share of the between-industry increase in dispersion from 1996-2002 to 2012-2018 accounted for by the top 30 industries is 98.1 percent in the LEHD data, 105 percent in the CPS-LEHD data, and 96.2 percent in the OEWS data.
\footnotetext{
\({ }^{34}\) The covariance component is large in all approaches and datasets as shown in the online Appendix, e.g., online Appendix Tables A4, D1, and G3.
}
\section*{VI. Industry Premia and Worker Effects in the Top 30 Industries}
In Table 3, we show that over our sample period all of the top 19 high-paying industries exhibit earnings per worker increases relative to the mean and ten of the top 11 low-paying industries exhibit earnings per worker decreases relative to the mean. \({ }^{35}\) In this section, we show that this translates into rising industry premia and worker effects for the top 19 high-paying industries and falling industry premia and worker effects for the top 11 low-paying industries.
At the industry-level, the average change in earnings per worker is equal to the sum of the change in the average worker effect plus the sum of the change in the industry premia. For the AKM decomposition, we have

We use analogous expressions for the CPS-LEHD human capital decomposition and OEWS decomposition. \({ }^{36}\) We start with the LEHD AKM based decomposition of the change in average industry earnings, shown in Figure 5. For each of the 30 industries, we report the change in average worker effects and industry premia, where industries are ranked by the changes in the industry's average pay.
In 23 of the top 30 industries, the change in the average worker and industry premia have the same sign. The correlation in the top 30 industries between the changing industry premia and changing average worker effects is 0.53 . Notable contributors to this correlation are high-paying industries like Other Information Services with a very large increase its average worker effect of \(42.3 \log\) points ( 52.7 percent) and its industry premia of \(27.8 \log\) points ( 32.1 percent) and low-paying industries like Grocery Stores with a large decline in its average worker effect of 11.5 log points ( 12.2 percent) and its industry premia of \(7.9 \log\) points ( 8.2 percent).
In 8 of our 19 high-paying industries (Figure 5, panel B), the average worker effect contributes more than \(10.0 \log\) points ( 10.5 percent) to that industry's change in average earnings, while the average firm effect contributes less than 5.0 \(\log\) points ( 5.1 percent). These include the two manufacturing industries, as well as Professional and Commercial Equipment and Supplies Merchant Wholesalers (4234), and Management of Companies and Enterprises (5511).
We show that the same patterns hold on average for the top 19 high-paying industries and top 11 low-paying industries using the CPS-LEHD human capital and OEWS occupation decompositions in Figure 6. First, panel A of the figure shows the average changes from the AKM based decomposition consistent with Figure 6. Panel B shows the results using the CPS-LEHD data with the earnings decomposition
\footnotetext{
\({ }^{35}\) The one exception in the top 11 industries is the very low-paying Employment Services (5613) industry that on average has earnings that are more than 50 percent lower than average and has a modest increase of about 2 percent.
\({ }^{36}\) The exact formulas for these CPS-LEHD and OEWS decompositions are online Appendices D and G, respectively. The CPS-LEHD decomposition is given as online Appendix equation (D3) and that of the OEWS decomposition is given as online Appendix equation (G3).
}
Panel A. Top 11 low-paying industries
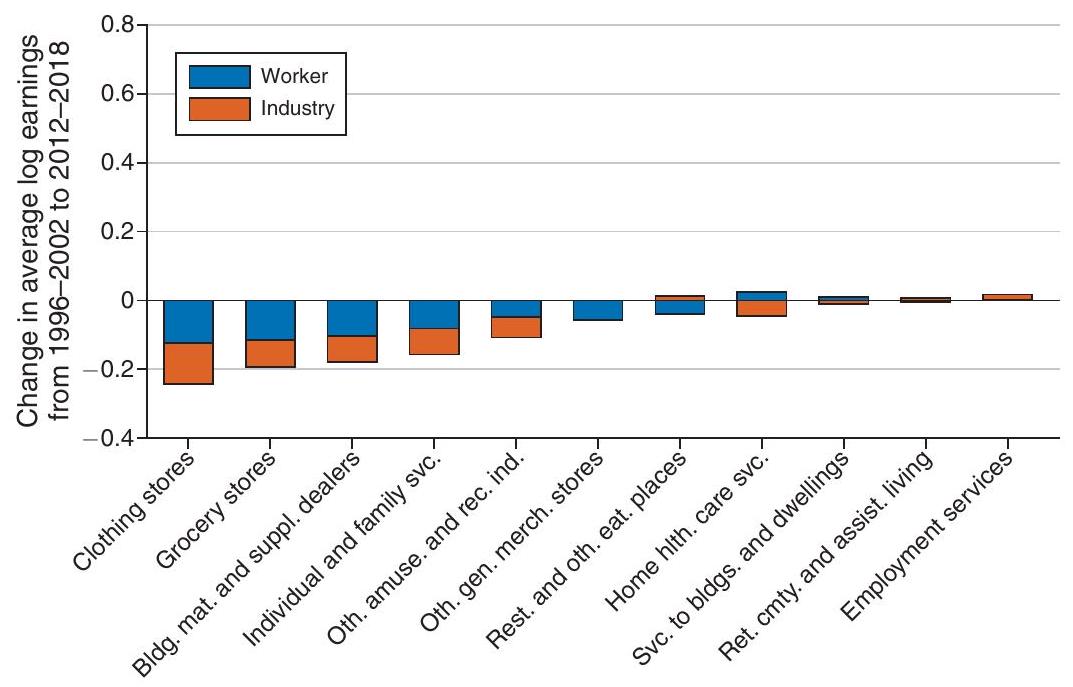
Panel B. Top 19 high-paying industries
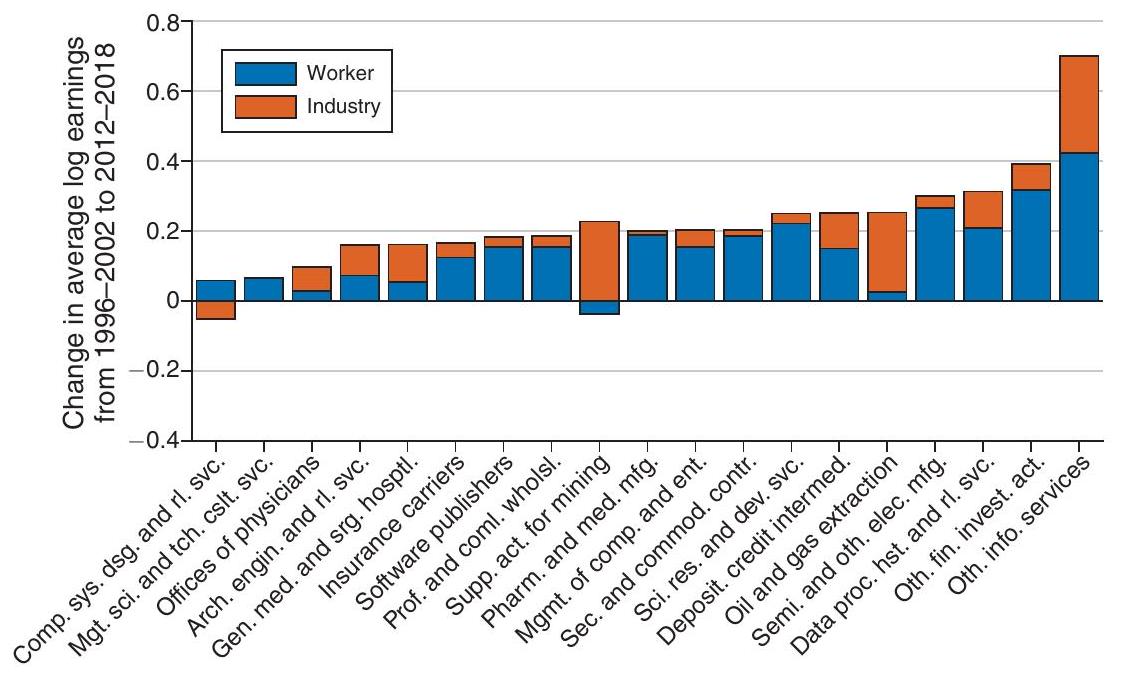
Figure 5. Change in Industry-Level Average Worker and Firm Effects
Notes: Authors' tabulations of LEHD microdata. Tabulations include workers with annual real earnings \(>\$ 3,770\) in EINs with 20 or more employees. See equation (6) for definitions. Average earnings are normalized by the overall average in each time interval. "Worker" combines the contribution of the worker effect with that of observable characteristics.
based upon observable human capital variables. The top 19 high-paying industries have positive changes on average in industry premia and human capital premia. The top 11 low-paying industries have negative changes on average in each of these components. Panel C depicts the patterns for the OEWS decomposition. Recall the magnitudes are not directly comparable to the LEHD AKM or CPS-LEHD human capital decompositions since the starting point is industry by occupation data. However, for the top 19 high-paying industries we find that on average increases
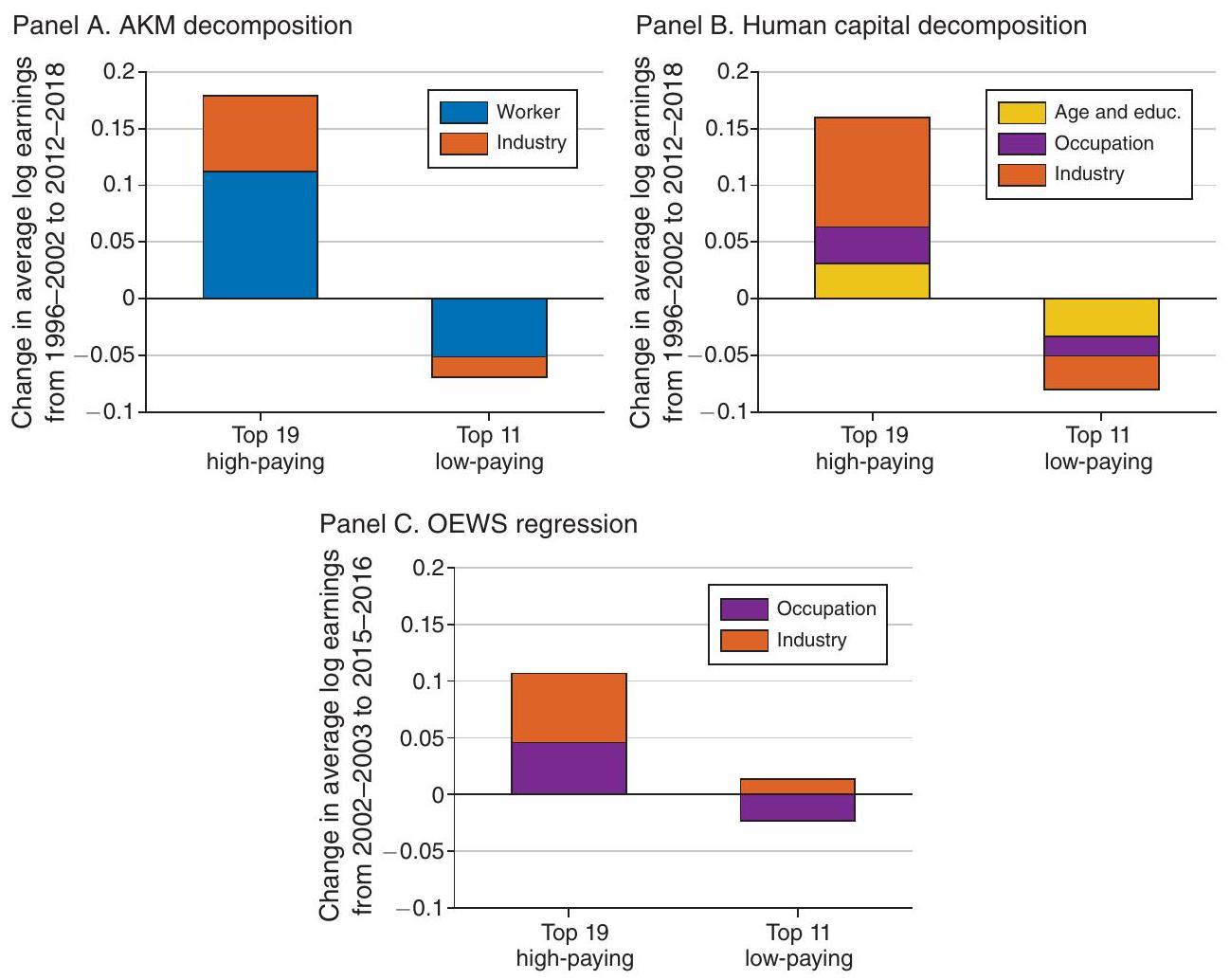
Figure 6. Worker and Employer Components of the Change in Industry Average Earnings
Notes: Panel A: Authors' tabulations of LEHD microdata, including workers with annual real earnings \(>\$ 3,770\) in EINs with 20 or more employees. "Worker" combines the contribution of the worker effect with that of observable characteristics. See equation (6) for definitions. Panel B: Authors' tabulations of linked CPS-LEHD microdata, including workers with annual real LEHD earnings \(>\$ 3,770\) who match to the CPS. See online Appendix equation (D3) for definitions. Panel C: Authors' tabulations of published OEWS aggregates. See online Appendix equation (G3) for definitions.
in industry premia and occupation effects. For the top 11 low-paying industries, we find on average decreases in occupation effects with modest offsetting industry premia effects. Notably the modest positive industry premia change effects on average in the top 11 low-paying industries are much smaller than the positive industry premia changes on average in the top 19 high-paying industries.
\section*{VII. Taking Stock}
Our findings provide a distinct perspective on the role of polarization in increasing inequality (e.g., Autor, Katz, and Kearney 2006, 2008; Goos and Manning 2007; and Acemoglu and Autor 2011). This literature highlights the increasing bifurcation of the occupational skill and pay structure. Our findings show that this manifests itself via low- and high-paying industries becoming more sorted on worker skill, becoming more skill-segregated across industries, and becoming relatively higher and relatively lower paid due to rising industry premia in high-paying sectors and falling industry premia in low-paying sectors.
Panel A. Employment change by initial premium
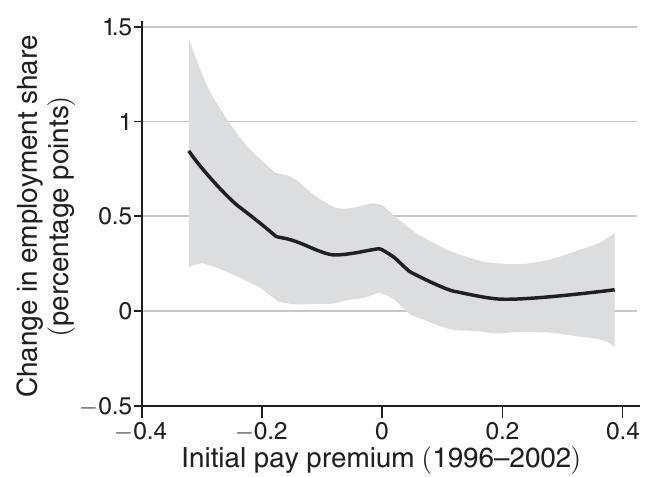
Panel C. Employment change by premium change
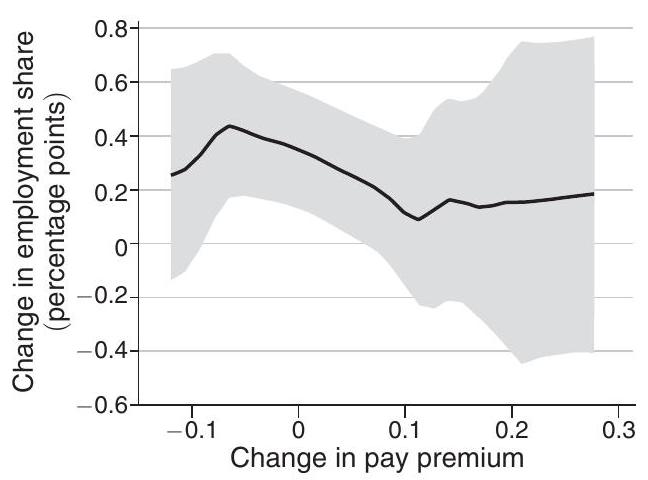
Panel B. Premium change by initial premium
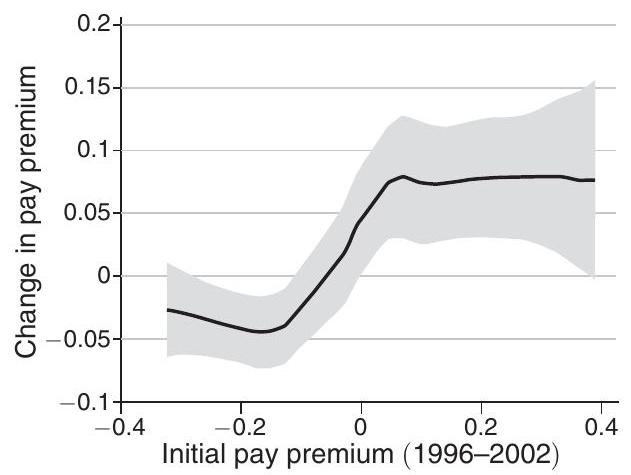
Panel D. Worker effect change by initial premium
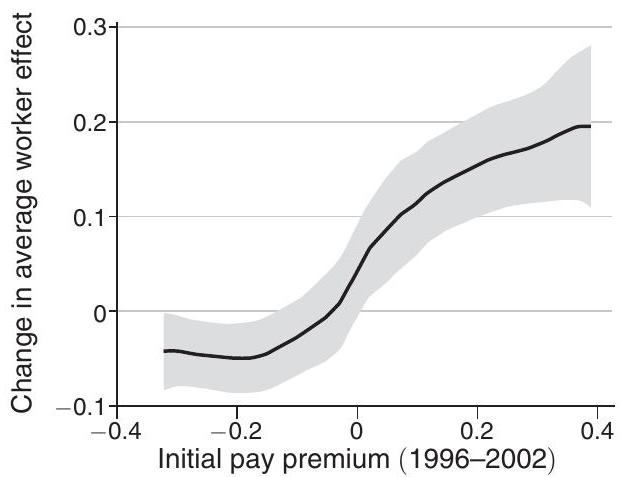
Figure 7. Changes in Employment and Earnings (Top 30 Industries)
Notes: Authors' tabulations of LEHD microdata. Tabulations include workers with annual real earnings \(>\$ 3,770\) in EINs with 20 or more employees. Graphs plot local polynomial estimates of the variable on the vertical axis on the horizontal axis. Shaded regions indicate 95 percent confidence intervals. Changes compare 2012-2018 with 1996-2002.
This industry polarization is concentrated in relatively small set of industries in the tails of the industry earnings distribution. Figure 7 provides a visual summary of pattern of polarization in the top 30 industries that dominate rising earnings inequality. Both the low industry premia industries and high industry premia industries in the top 30 exhibit (upper-left panel) increases in employment shares with especially large employment share increases in the low premia industries. High initial premia industries exhibit increases in industry premia while the low premia industries exhibit declines in industry premia (upper-right panel)—with high premia industries having especially large increases. Industries with declines in the industry premia (the low premia industries) and industries with increases in the industry premia (the high premia industries) have had increases in employment shares (lower-left panel). Increases in worker effects are large in the high initial premia industries and declines in worker effects are present in the low premia industries.
In short, the upper-tail component is due to rising industry premia without much change in employment; the lower tail is driven by falling industry premia and rising
employment. These findings suggest that different forces are at play. At the top, the patterns are consistent with a positive demand shift, yielding higher wages and slightly higher quantities. At the bottom, the patterns are consistent with a positive supply shift, yielding lower wages and higher quantities.
The results are thus related but distinct from the existing polarization literature, which argues that the growth of employment in low-wage sectors is in part driven by the decline of middle-skill blue-collar production and white-collar office-administrative occupations. Relatedly, Acemoglu and Restrepo (2022) argue that skill groups that have been displaced by automation of routine tasks have seen falling earnings as these groups are reallocated to activities where they have weaker comparative advantage. These findings and perspective have a natural mapping to our findings on the growth of low-paid service industries, which have substantially expanded even as their already-low pay premia have fallen further.
In emphasizing the role of industry, it is important to emphasize the prominent role for industry premia in our findings. Conceptually, industry premia (and more generally firm premia) are consistent with the presence of frictions or market imperfections (e.g., monopsony power) in labor markets. Industry and firm premia may also arise from other factors than market imperfections such as environments where the marginal product of labor depends on the unique product attributes of the firm or industry, see Tervio (2008). While we acknowledge there are issues for mapping such mechanisms to the estimated firm and industry effects, the robustness of our findings across different source data and methodologies suggests that it is important to understand the sources of the direct and indirect effects of the industry premia in accounting for rising earnings inequality. That is, whether we use AKM to control for worker effects, or a human capital approach to control for age, education, and occupation effects, or rich occupation data to control for occupation effects, we find a role for rising between-industry premia and especially a role for a rising covariance between those premia and alternative estimates of worker premia (AKM worker effects, education and occupation premia).
Viewed from this perspective, our findings rule out explanations of rising inequality that don't have a prominent role for industry premia through both direct and sorting effects. While we have noted our results are complementary to Acemoglu and Restrepo (2022), the structural model in their paper has competitive labor markets without frictions. Hence, their model implies that the prominent role of industry they find is associated with rising segregation of workers between industries without any role for industry premia and sorting associated with such premia. In other words, their model focuses on the changing composition of workers across industries without any interaction of that changing composition with industry premia. Our empirical results suggest that enhancing the structural model to permit such interactions is critical. One way to do this would be to consider the role of frictions and imperfect competition in labor markets for capturing this important aspect of variation in the data. This argument is not restricted to this specific mechanism (which is a form of skill biased technical change). Our results imply that any mechanism (whether driven by frictions, distortions, or some other mechanism) must account for the combined contribution of increased between-industry sorting and rising between-industry premia.
\section*{VIII. Conclusion}
Rising earnings inequality is dominated by rising between-industry inequality. High earnings workers are more likely to work in high earnings industries and with each other, and low earnings workers are more likely to work with in low earnings industries and with each other. This polarization of the industry earnings and employment structure is concentrated in a relatively small number of industries. About 10 percent of the 301 detailed four-digit NAICS industries account for almost all of rising between-industry dispersion, while accounting for less than 40 percent of employment. These thirty industries are drawn from the top and bottom of the earnings distribution in terms of industry-level averages. The top 19 high-paying industries exhibit increases in industry pay induced by both increases in industry premia and average worker effects. The top 11 low-paying industries exhibit decreases in industry pay induced by both decreases in industry premia and average worker effects. These inferences are robust to identifying industry premia and worker effects using the AKM approach, a standard human capital approach using worker observables including age, education, and occupation, and using high quality industry by occupation data.
The top 10 percent of industries that account for virtually all of rising between-industry inequality are not randomly spread across the distribution of industries but concentrated in specific industry clusters in the tails of the earnings distribution. At the high end, dominant industries are drawn from high-tech and STEM intensive industries, finance, mining, and selected industries in health. At the low end, dominant industries are drawn from selected industries in retail and health. The top 30 industries are in industry clusters that have exhibited structural transformations that have been the subject of independent study. Notably absent are the vast majority of industries in manufacturing. Part of the polarization story is the decline in production workers in manufacturing and the rise of employment and decline in earnings in key low pay service sectors.
The dominance of industry effects is closely linked to the rising importance of mega \((10,000+\) ) firms in the US economy. The increasing share of employment accounted for by mega firms is concentrated in the thirty four-digit industries that account for virtually all of rising between-industry dispersion. This rising share of employment at mega firms is accompanied by a declining size-earnings premium in the 11 low-paying industries. For mega firms in the 19 high-paying industries in the top 30, earnings premia rise sharply relative to other industries (albeit not as rapidly as other large but not mega firms in these industries).
We find there is a close connection between changes in the occupation distribution and our top 30 industries. Most of the increase in employment in top-paying occupations is accounted for by our 19 top-paying industries, while all of the increase in employment in low-paying occupations is in our 11 low-paying industries. For the top-paying industries, there is a substantial increase in earnings differentials for the top-paying occupations. Likewise there is a substantial decline in earnings differentials for the low-paying occupations in our low-paying industries.
In the top 19 high-paying industries there is a sharp increase in the share of workers with a bachelor's or advanced degree with accompanying declines in workers without such degrees. In the top 11 low-paying industries there is no such shift in
the distribution. On average, this distribution is dominated by workers with a high school diploma or less.
Our findings imply that understanding rising earnings inequality during the last several decades requires understanding the evolution of how firms organize themselves in a relatively small set of industries. Moreover, since it is the between-industry contribution that dominates, the common effects of reorganization across firms in the same industry matter. Many mechanisms such as changing technology, market structure, and globalization likely underlie rising earnings inequality. The focus of future research on the impact of such changes on rising earnings inequality should be on the uneven and concentrated impact of such mechanisms across industries. In addition, since between-industry sorting and industry premia play important roles, the mechanisms must interact with the factors that yield such effects including possibly frictions and imperfect competition in labor markets.
\section*{REFERENCES}
-Abowd, John, Francis Kramarz, and David Margolis. 1999. "High Wage Workers and High Wage Firms." Econometrica 67 (2): 251-333.
-Abowd, John M., Kevin L. McKinney, and Nellie L. Zhao. 2018. "Earnings Inequality and Mobility Trends in the United States: Nationally Representative Estimates from Longitudinally Linked Employer-Employee Data." Journal of Labor Economics 36 (S1): S183-300.
Abowd, John, Bryce Stephens, Lars Vilhuber, Fredrik Andersson, Kevin McKinney, Marc Roemer, and Simon Woodcock. 2009. "The LEHD Infrastructure Files and the Creation of the Quarterly Workforce Indicators." In Producer Dynamics: New Evidence from Micro Data, edited by Timothy Dunne, J. Bradford Jensen, and Mark J. Roberts, 149-230. Chicago: University of Chicago Press.
Acemoglu, Daron, and David H. Autor. 2011. "Skills, Tasks and Technologies: Implications for Employment and Earnings." In Handbook of Labor Economics, Vol. 4, edited by Orley Ashenfelter and David Card, 1043-1171. Amsterdam: Elsevier-North Holland.
-Acemoglu, Daron, and Pascual Restrepo. 2022. "Tasks, Automation, and the Rise in US Wage Inequality." Econometrica \(90(5): 1973-2016\).
-Autor David, David Dorn, Lawrence F. Katz, Christina C. Patterson, and John Van Reenen. 2020. "The Fall of the Labor Share and the Rise of Superstar Firms." Quarterly Journal of Economics \(135(2): 645-709\).
-Autor, David H., Lawrence F. Katz, and Melissa S. Kearney. 2006. "The Polarization of the U.S. Labor Market." American Economic Review 96 (2): 189-94.
-Autor, David H., Lawrence F. Katz, and Melissa S. Kearney. 2008. "Trends in U.S. Wage Inequality: Revising the Revisionists." Review of Economics and Statistics 90 (2): 300-23.
- Barth, Erling, Alex Bryson, James C. Davis, and Richard Freeman. 2016. "It's Where You Work: Increases in the Dispersion of Earnings across Establishments and Individuals in the United States." Journal of Labor Economics 34 (S2): S67-97.
-Bloom, Nicholas, Fatih Guvenen, Benjamin S. Smith, Jae Song, and Till von Wachter. 2018. "The Disappearing Large-Firm Premium." AEA Papers and Proceedings 108: 317-22.
Bureau of Economic Analysis. 2021. "Personal Consumption Expenditures Price Index." Bureau of Economic Analysis. https://www.bea.gov/data/personal-consumption-expenditures-price-index (accessed November 22, 2021).
Bureau of Labor Statistics. 2022. "Occupation Employment and Wage Survey Data." Bureau of Labor Statistics. https://www.bls.gov/oes/tables.htm (accessed July 26, 2022).
Bureau of Labor Statistics. 2022. "Quarterly Census of Employment and Wages." https://https://www. bls.gov/cew/ (accessed July 26, 2022).
Bonhomme, Stéphane, Kerstin Holzheu, Thibaut Lamadon, Elena Manresa, Magne Mogstad and Bradley Setzler. 2022. "How Much Should We Trust Estimates of Firm Effects and Worker Sorting?" Journal of Labor Economics 41 (2): 291-322.
Briskar, Juraj, José Rodríguez Mora, Edoardo di Porto, and Cristina Tealdi. 2022. "Decomposition of Italian Inequality." Unpublished.
- Card, David, Ana Cardoso, and Patrick Kline. 2016. "Bargaining, Sorting, and the Gender Wage Gap: Quantifying the Effect of Firms on the Relative Pay of Women." Quarterly Journal of Economics 131 (2): 633-86.
-Card, David, Jorg Heining, and Patrick Kline. 2013. "Workplace Heterogeneity and the Rise of West German Wage Inequality." Quarterly Journal of Economics 128 (3): 967-1015.
-Card, David, Jesse Rothstein, and Moises Yi. 2024. "Industry Wage Differentials: A Firm- Based Approach." Journal of Labor Economics 42 (S1): S11-S59.
Davis, Steve J., and John Haltiwanger. 1991. "Wage Dispersion Between and Within U.S. Manufacturing Plants, 1963-1986." Brookings Papers: Microeconomics 21: 115-20.
Dey, Matthew, Susan Houseman, and Anne Polivka. 2010. "What Do We Know About Contracting Out in the United States? Evidence from Household and Establishment Surveys." In Labor in the New Economy, edited by Katharine Abraham, James Spletzer, and Michael Harper, 267-304. Chicago: University of Chicago Press.
Dorn, David, Johannes Schmeider, James Spletzer, and Lee Tucker. 2023. "Domestic Outsourcing in the United States." Unpublished.
-Dunne, Timothy, Lucia Foster, John Haltiwanger, and Ken Troske. 2004. "Wage and Productivity Dispersion in United States Manufacturing: The Role of Computer Investment." Journal of Labor Economics 22 (2): 397-429.
Fernald, John. 2014. "Productivity and Potential Output before, during, and after the Great Recession." NBER Macroeconomics Annual 29 (1): 1-51.
-Foster, Lucia, John Haltiwanger, and C. J. Krizan. 2006. "Market Selection, Reallocation, and Restructuring in the U.S. Retail Trade Sector in the 1990s." Review of Economics and Statistics 88 (4): 748-58.
-Goos, Maarten, and Alan Manning. 2007. "Lousy and Lovely Jobs: The Rising Polarization of Work in Britain." Review of Economics and Statistics 89 (1): 118-33.
—Guimarães, Paulo, and Pedro Portugal. 2010. "A Simple Feasible Procedure to Fit Models with High-Dimensional Fixed Effects." Stata Journal 10 (4): 628-49.
-Haltiwanger, John, Henry Hyatt, and James Spletzer. 2023. "Increasing Earnings Inequality: Reconciling Evidence from Survey and Administrative Data." Journal of Labor Economics 41 (S1): S61-93.
Haltiwanger, John, Henry Hyatt, and James Spletzer. 2024. Data and Code for: "Rising Top, Falling Bottom: Industries and Rising Wage Inequality." Nashville, TN: American Economic Association; distributed by Inter-university Consortium for Political and Social Research, Ann Arbor, MI. https://doi.org/10.3886/E198751V1.
Haltiwanger, John, and James Spletzer. 2020. "Between-firm changes in earnings inequality: the dominant role of industry effects." NBER Working Paper 26786.
Haltiwanger, John, and James Spletzer. 2022. "Rising Between-Firm Inequality and Declining Labor Market Fluidity: Evidence of a Changing Job Ladder." In Measuring Distribution and Mobility of Income and Wealth, edited by Raj Chetty, John Friedman, Janet Gornick, Barry Johnson, and Arthur Kennickell, 45-67. Chicago: University of Chicago Press.
Hecker, Daniel. 2005. "High-Technology Employment: A NAICS-Based Update." Monthly Labor Review 128 (7): 57-72.
-Hoffman, Florian, David S. Lee, and Thomas Lemieux. 2020. "Growing Income Inequality in the United States and Other Advanced Economies." Journal of Economic Perspectives 34 (4): 52-78.
Hoffman, Florian, David S. Lee, and Thomas Lemieux. 2020. Replication data and code for: "Growing Income Inequality in the United States and Other Advanced Economies" Nashville, TN: American Economic Association; distributed by Inter-university Consortium for Political and Social Research, Ann Arbor, MI. https://doi.org/10.3886/E122201V1.
-Krueger, Alan, and Lawrence Summers. 1988. "Efficiency Wages and the Inter-Industry Wage Structure." Econometrica 56 (2): 259-93.
Oliner, Stephen, Daniel Sichel and Kevin Stiroh. 2007. "Explaining a Productive Decade." Brookings Papers on Economic Activity 37 (2).
Ruggles, Steven, Sarah Flood, Matthew Sobek, Daniel Backman, Annie Chen, Grace Cooper, Stephanie Richards, Renae Rodgers, and Megan Schouweiler. "IPUMS USA: Version 15.0 [dataset]." IPUMS. Minneapolis, MN: IPUMS, 2024. https://doi.org/10.18128/D010.V15.0.
-Song, Jae, David J. Price, Fatih Guvenen, Nicholas Bloom, and Till von Wachter. 2019. "Firming Up Inequality." Quarterly Journal of Economics 134 (1): 1-50.
- Tervio, Marko. 2008. "The Difference That CEOs Make: An Assignment Model Approach." American Economic Review 98 (3): 642-68.
US Census Bureau. 2021. "Current Population Survey (CPS) - Annual Social and Economic Supplements (ASEC) microdata." US Census Bureau. https://www.census.gov/data/datasets/time-series/ demo/cps/cps-asec.html (accessed November 15, 2021).
US Census Bureau. 2023. "Longitudinal Business Database (LBD) microdata." US Census Bureau. https://www.census.gov/programs-surveys/ces/data/restricted-use-data/longitudinal-businessdatabase.html.
US Census Bureau. 2023. "Longitudinal-Employer Household Dynamics (LEHD) micro- data." US Census Bureau. https://lehd.ces.census.gov/data/lehd-snapshot-doc/latest/.
Walker, Ed. 1997. "The Census Bureau's Business Register: Basic Features and Quality Issues." Paper presented at the Joint Statistical Meetings of the American Statistical Association, Anaheim, CA.