Google DeepMind’s AI systems can now solve complex math problems
Google DeepMind 的 AI 系統現在可以解決複雜的數學問題
AlphaProof and AlphaGeometry are steps toward building systems that can reason, which could unlock exciting new capabilities.
AlphaProof 和 AlphaGeometry 向著構建能夠推理的系統邁出了一步,這可能會釋放令人興奮的新功能。

STEPHANIE ARNETT / 麻省理工科技評論 | 公共領域
AI models can easily generate essays and other types of text. However, they’re nowhere near as good at solving math problems, which tend to involve logical reasoning—something that’s beyond the capabilities of most current AI systems.
人工智慧模型可以輕鬆生成文章和其他類型的文本。然而,它們在解決數學問題方面的能力遠遠不夠,因為數學問題往往涉及邏輯推理,而這超出了當前大多數人工智慧系統的能力範圍。
But that may finally be changing. Google DeepMind says it has trained two specialized AI systems to solve complex math problems involving advanced reasoning. The systems—called AlphaProof and AlphaGeometry 2—worked together to successfully solve four out of six problems from this year’s International Mathematical Olympiad (IMO), a prestigious competition for high school students. They won the equivalent of a silver medal at the event.
但這種情況可能終於要改變了。Google DeepMind 表示,他們已經訓練了兩個專門的人工智慧系統來解決涉及高級推理的複雜數學問題。這兩個系統分別稱為 AlphaProof 和 AlphaGeometry 2,它們在今年為高中生舉辦的 prestigious 競賽國際數學奧林匹克競賽 (IMO) 中的六道題目中成功解出了四道。他們在比賽中贏得了相當於銀牌的成績。
It’s the first time any AI system has ever achieved such a high success rate on these kinds of problems. “This is great progress in the field of machine learning and AI,” says Pushmeet Kohli, vice president of research at Google DeepMind, who worked on the project. “No such system has been developed until now which could solve problems at this success rate with this level of generality.”
這是人工智慧系統首次在解決此類問題上取得如此高的成功率。「這是機器學習和人工智慧領域的一項重大進展,」Google DeepMind 研究副總裁 Pushmeet Kohli 表示,他參與了該專案。「到目前為止,還沒有開發出任何系統能夠以這種成功率和通用性來解決問題。」
There are a few reasons math problems that involve advanced reasoning are difficult for AI systems to solve. These types of problems often require forming and drawing on abstractions. They also involve complex hierarchical planning, as well as setting subgoals, backtracking, and trying new paths. All these are challenging for AI.
涉及高級推理的數學問題對人工智慧系統來說難以解決,原因有很多。這些類型的問題通常需要形成和利用抽象概念。它們還涉及複雜的層次化規劃,以及設定子目標、回溯和嘗試新路徑。這些對人工智慧來說都是挑戰。
“It is often easier to train a model for mathematics if you have a way to check its answers (e.g., in a formal language), but there is comparatively less formal mathematics data online compared to free-form natural language (informal language),” says Katie Collins, an researcher at the University of Cambridge who specializes in math and AI but was not involved in the project.
「如果你有辦法檢查模型的答案(例如,使用形式語言),那麼訓練一個數學模型通常會更容易,但是與自由形式的自然語言(非正式語言)相比,網上的形式數學資料相對較少,」劍橋大學專門研究數學和人工智慧的研究員 Katie Collins 表示,她沒有參與該專案。
Bridging this gap was Google DeepMind’s goal in creating AlphaProof, a reinforcement-learning-based system that trains itself to prove mathematical statements in the formal programming language Lean. The key is a version of DeepMind’s Gemini AI that’s fine-tuned to automatically translate math problems phrased in natural, informal language into formal statements, which are easier for the AI to process. This created a large library of formal math problems with varying degrees of difficulty.
Google DeepMind 在創造 AlphaProof 時,目標是彌合這一差距。AlphaProof 是一個基於強化學習的系統,它會訓練自己在正式程式語言 Lean 中證明數學陳述。關鍵在於 DeepMind 的 Gemini AI 版本,它經過微調,可以自動將以自然、非正式語言表述的數學問題轉換為 AI 更容易處理的正式陳述。這就創建了一個包含各種難度的正式數學問題的大型資料庫。
Automating the process of translating data into formal language is a big step forward for the math community, says Wenda Li, a lecturer in hybrid AI at the University of Edinburgh, who peer-reviewed the research but was not involved in the project.
愛丁堡大學混合人工智慧講師李文達(音譯)表示,自動將數據轉換為正式語言的過程是數學界的一大進步。他對這項研究進行了同行評審,但沒有參與該項目。
“We can have much greater confidence in the correctness of published results if they are able to formulate this proving system, and it can also become more collaborative,” he adds.
他補充說:「如果他們能夠建立這個證明系統,我們對已發表結果的正確性就會更有信心,而且它也會變得更具協作性。」
The Gemini model works alongside AlphaZero—the reinforcement-learning model that Google DeepMind trained to master games such as Go and chess—to prove or disprove millions of mathematical problems. The more problems it has successfully solved, the better AlphaProof has become at tackling problems of increasing complexity.
Gemini 模型與 AlphaZero(Google DeepMind 訓練用於掌握圍棋和西洋棋等遊戲的強化學習模型)協同工作,以證明或反駁數百萬個數學問題。它成功解決的問題越多,AlphaProof 在處理日益複雜的問題方面就越出色。
Although AlphaProof was trained to tackle problems across a wide range of mathematical topics, AlphaGeometry 2—an improved version of a system that Google DeepMind announced in January—was optimized to tackle problems relating to movements of objects and equations involving angles, ratios, and distances. Because it was trained on significantly more synthetic data than its predecessor, it was able to take on much more challenging geometry questions.
雖然 AlphaProof 被訓練用於處理各種數學主題的問題,但 AlphaGeometry 2(Google DeepMind 在 1 月份發布的系統的改進版本)經過優化,可以處理與物體運動以及涉及角度、比率和距離的方程式有關的問題。由於它接受了比其前身更多的合成數據訓練,因此它能夠處理更具挑戰性的幾何問題。
To test the systems’ capabilities, Google DeepMind researchers tasked them with solving the six problems given to humans competing in this year’s IMO and proving that the answers were correct. AlphaProof solved two algebra problems and one number theory problem, one of which was the competition’s hardest. AlphaGeometry 2 successfully solved a geometry question, but two questions on combinatorics (an area of math focused on counting and arranging objects) were left unsolved.
為了測試這些系統的能力,Google DeepMind 的研究人員要求它們解決今年國際數學奧林匹克競賽中給人類選手的六個問題,並證明答案是正確的。AlphaProof 解決了兩個代數問題和一個數論問題,其中一個是比賽中最難的。AlphaGeometry 2 成功地解決了一個幾何問題,但兩個關於組合數學(一個專注於計算和排列物體的數學領域)的問題仍未解決。
“Generally, AlphaProof performs much better on algebra and number theory than combinatorics,” says Alex Davies, a research engineer on the AlphaProof team. “We are still working to understand why this is, which will hopefully lead us to improve the system.”
AlphaProof 團隊的研究工程師亞歷克斯·戴維斯說:「一般來說,AlphaProof 在代數和數論方面的表現比組合數學好得多。我們仍在努力理解其中的原因,希望能藉此改進系統。」
Two renowned mathematicians, Tim Gowers and Joseph Myers, checked the systems’ submissions. They awarded each of their four correct answers full marks (seven out of seven), giving the systems a total of 28 points out of a maximum of 42. A human participant earning this score would be awarded a silver medal and just miss out on gold, the threshold for which starts at 29 points.
兩位知名數學家蒂姆·高爾斯和約瑟夫·邁爾斯審閱了這些系統提交的答案。他們給予四個正確答案中的每一個滿分(七分),使這些系統在總分 42 分中獲得了 28 分。獲得這個分數的人類參與者將獲得銀牌,僅差一分就能獲得金牌,金牌的門檻是 29 分。
This is the first time any AI system has been able to achieve a medal-level performance on IMO questions. “As a mathematician, I find it very impressive, and a significant jump from what was previously possible,” Gowers said during a press conference.
這是 AI 系統首次在 IMO 題目中取得獎牌級別的表現。「作為一名數學家,我認為這非常令人印象深刻,並且與之前可能取得的成就相比是一個顯著的飛躍,」高爾斯在新聞發布會上表示。
Myers agreed that the systems’ math answers represent a substantial advance over what AI could previously achieve. “It will be interesting to see how things scale and whether they can be made faster, and whether it can extend to other sorts of mathematics,” he said.
邁爾斯同意,這些系統的數學答案代表著與 AI 之前所能取得的成就相比的重大進步。「看看事情如何發展,它們是否可以變得更快,以及它是否可以擴展到其他類型的數學,將會很有趣,」他說。
Creating AI systems that can solve more challenging mathematics problems could pave the way for exciting human-AI collaborations, helping mathematicians to both solve and invent new kinds of problems, says Collins. This in turn could help us learn more about how we humans tackle math.
柯林斯說,創造能夠解決更具挑戰性數學問題的 AI 系統,可以為令人興奮的人工智慧協作鋪平道路,幫助數學家解決和發明新型問題。反過來,這可以幫助我們更多地了解人類是如何處理數學的。
“There is still much we don't know about how humans solve complex mathematics problems,” she says.
「關於人類如何解決複雜的數學問題,我們還有很多不知道的地方,」她說。
Deep Dive 深度解析
Artificial intelligence 人工智慧

What is AI? 什麼是人工智慧?
Everyone thinks they know but no one can agree. And that’s a problem.
每個人都認為自己知道,但沒有人能達成共識。而這就是問題所在。

What are AI agents?
什麼是人工智慧代理?
The next big thing is AI tools that can do more complex tasks. Here’s how they will work.
下一個科技熱潮是能夠執行更複雜任務的 AI 工具。以下是它們的運作方式。

How to use AI to plan your next vacation
如何使用 AI 來規劃您的下次旅行
AI tools can be useful for everything from booking flights to translating menus.
從預訂航班到翻譯菜單,AI 工具在各方面都能派上用場。
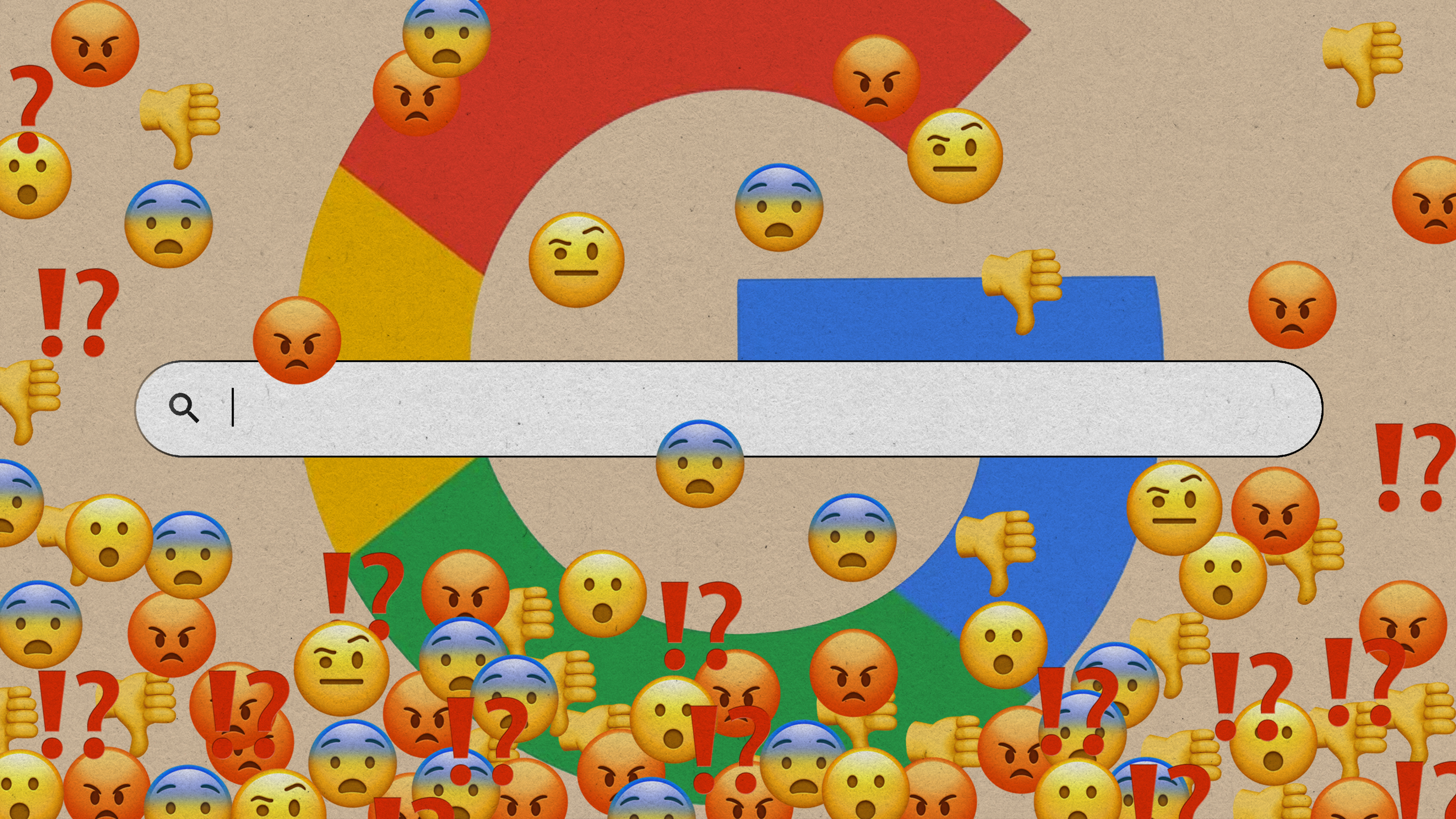
Why Google’s AI Overviews gets things wrong
Google AI 總覽為何會出錯
Google’s new AI search feature is a mess. So why is it telling us to eat rocks and gluey pizza, and can it be fixed?
Google 的新 AI 搜尋功能一團糟。為什麼它會讓我們吃石頭和黏糊糊的披薩,而且可以修復嗎?
Stay connected 保持聯繫
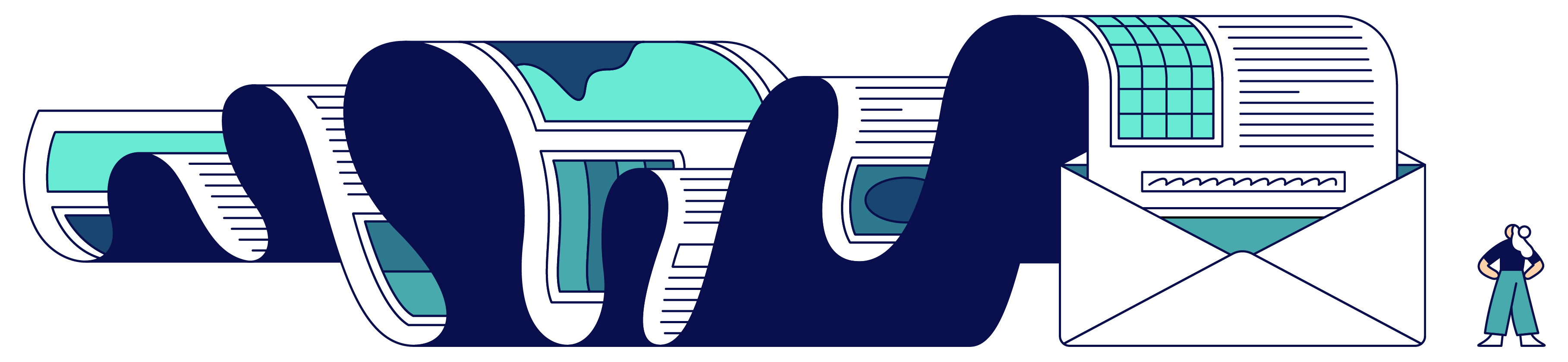
插圖:Rose Wong
Get the latest updates from
MIT Technology Review
取得來自《麻省理工科技評論》的最新資訊
Discover special offers, top stories,
upcoming events, and more.
發掘特別優惠、熱門報導、即將舉行的活動等資訊。