GPU Cloud Economics Explained – The Hidden Truth
GPU 云经济学解析 - 隐藏的真相
CPU vs GPU Cloud Differences, TCO Model, PUE, Hyperscalers Disadvantage
CPU 与 GPU 云的差异,TCO 模型,PUE,超大规模数据中心的劣势
Over the last year there has been an explosion in the number of pureplay GPU clouds. We kid you not - more than a dozen different firms’ equity or debt proposals have crossed our desks for this exact purpose, there are likely many more out there that we haven’t even seen. The deal flow has finally slowed down, so let’s publicly examine the economics at play here more deeply.
在过去一年中,纯 GPU 云的数量激增。我们不是在开玩笑——超过十家不同公司的股权或债务提案已经出现在我们的桌面上,可能还有更多我们未曾见过的。交易流终于放缓,因此让我们更深入地公开审视这里的经济学。
The first quick point to address is the general motivation for the massive influx of new clouds. While there is certainly a unique set of infrastructure challenges, GPU clouds are significantly easier to operate than general purpose clouds from a software perspective. Third party pureplay GPU clouds do not need to worry about advanced database services, block storage, security guarantees for multi-tenancy, APIs for various 3rd party services providers, or in many cases even virtualization doesn’t matter.
首先需要解决的一个快速要点是大量新云涌入的总体动机。虽然确实存在一系列独特的基础设施挑战,但从软件的角度来看,GPU 云的操作显著比通用云更容易。第三方纯 GPU 云不需要担心高级数据库服务、块存储、多租户的安全保障、各种第三方服务提供商的 API,或者在许多情况下,甚至虚拟化也无关紧要。
A hilarious example of how little cloud developed software, outside of awesome models of course, matters for AI is at AWS. While AWS loves to talk up their SageMaker platform as a great tool for their customers to create, train, and deploy models in the cloud, it’s a clear example of “do as I say, not as I do.” Amazon uses Nvidia’s Nemo framework in place of Sagemaker for Titan, their very best model. Note that Titan is significantly worse than numerous open-source models! While Nemo and Sagemaker aren’t exactly analogous, it exemplifies how little the cloud’s “value add” software matters.
一个搞笑的例子,说明云开发的软件(当然,除了出色的模型)对人工智能的重要性有多小,就是在 AWS。虽然 AWS 喜欢宣传他们的 SageMaker 平台是客户在云中创建、训练和部署模型的好工具,但这明显是“说一套,做一套”的例子。亚马逊在 Titan(他们最好的模型)中使用的是 Nvidia 的 Nemo 框架,而不是 SageMaker。请注意,Titan 的表现明显不如许多开源模型!虽然 Nemo 和 SageMaker 并不完全相同,但它例证了云的“增值”软件有多么不重要。
Furthermore, while the standard cloud needs supreme flexibility and fungibility in compute, storage, RAM, and networking, the GPU cloud needs far fewer options due to the relative homogeneity of workloads. Servers are generally committed to for long time scales, and the H100 is the optimal GPU for basically all modern use cases, including LLM training and high volume LLM/diffusion inference. Infrastructure choices for end users are mostly about how GPUs you need. Of course, you need to ensure you have performant networking, but overshooting on networking spend is not a massive concern for most users because they are tiny cost relative to the GPUs.
此外,虽然标准云需要在计算、存储、RAM 和网络方面具备极高的灵活性和可替代性,但由于工作负载的相对同质性,GPU 云所需的选项要少得多。服务器通常是长期承诺的,而 H100 是几乎所有现代用例的最佳 GPU,包括LLM训练和高容量LLM/扩散推理。最终用户的基础设施选择主要是关于你需要多少 GPU。当然,你需要确保网络性能良好,但对于大多数用户来说,网络支出的过度投资并不是一个重大问题,因为与 GPU 相比,这些成本微不足道。
For all but the biggest users, locality of your existing data isn’t even that important during training and inference because egress costs are tiny. The data can be transformed and transferred, and high-performance storage is not terribly difficult for a cloud provider to purchase from Pure, Weka, Vast, etc, as again, like other items, storage constitutes a very small portion of the cost of AI infrastructure.
对于除最大用户之外的所有用户,现有数据的本地性在训练和推理过程中并不是那么重要,因为出口成本微乎其微。数据可以被转换和传输,而高性能存储对于云服务提供商来说,从 Pure、Weka、Vast 等公司购买并不是特别困难,因为与其他项目一样,存储在 AI 基础设施的成本中占据的比例非常小。
CPU vs GPU Colocation Total Cost of Ownership (TCO)
CPU 与 GPU 共址总拥有成本(TCO)
Even ignoring the lack of a moat regarding GPU clouds (outside of a cozy relationship with Nvidia), the true driver of this boom in new providers is the total cost of ownership (TCO) equation for CPU servers versus GPU servers in a colocation (colo) environment. CPU servers’ TCO have a more varied number of important factors to balance, while GPUs, due to Nvidia’s extremely high margins, are dominated purely by capital costs.
即使忽略与 Nvidia 的亲密关系所带来的 GPU 云缺乏护城河的问题,这一新供应商繁荣的真正驱动因素是 CPU 服务器与 GPU 服务器在托管环境中的总拥有成本(TCO)方程。CPU 服务器的 TCO 需要平衡更多种类的重要因素,而由于 Nvidia 的极高利润率,GPU 则纯粹受资本成本的主导。
In other words, since capital is the only real barrier to entry, not physical infrastructure, it’s no surprise there are so many new entrants.
换句话说,由于资本是唯一真正的进入壁垒,而不是物理基础设施,因此有这么多新进入者并不奇怪。
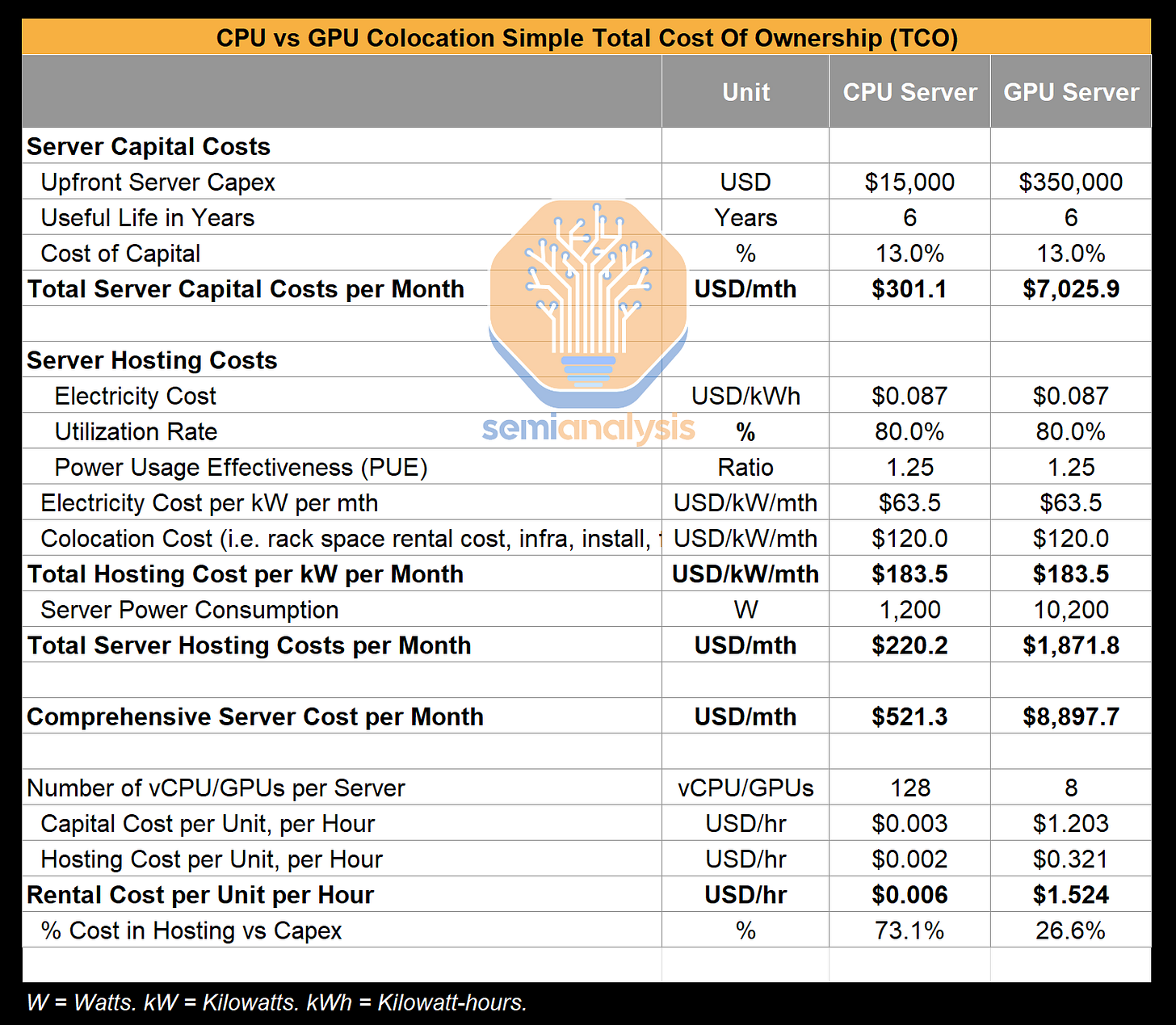
OEM 定价将网络成本分配到服务器级别,而非超大规模
In the case of CPU servers, the various hosting costs ($220 a month) are of similar magnitude to the capital costs ($301 a month). Compare this to a GPU server, where the various hosting costs ($1,871 a month) are completely dwarfed by the capital costs ($7,025 a month). This is the core reason why 3rd party clouds can exist.
在 CPU 服务器的情况下,各种托管成本(每月 220 美元)与资本成本(每月 301 美元)大致相当。相比之下,GPU 服务器的各种托管成本(每月 1,871 美元)完全被资本成本(每月 7,025 美元)所淹没。这是第三方云存在的核心原因。
The hyperscale cloud providers such as Google, Amazon, Microsoft can optimize their hosting costs significantly by being better designers and operators of datacenters. Take for example the metric of Power Usage Effectiveness (PUE). It is a metric that compares the total amount of energy used by a datacenter compared to the energy delivered to computing equipment. Efforts to reduce this metric generally center around cooling and power delivery. Google, Amazon, and Microsoft are amazing, so their PUE’s are approaching as close to 1 as possible.
超大规模云服务提供商如谷歌、亚马逊和微软可以通过更好地设计和运营数据中心显著优化其托管成本。以电力使用效率(PUE)为例。它是一个比较数据中心使用的总能量与提供给计算设备的能量的指标。减少该指标的努力通常集中在冷却和电力供应上。谷歌、亚马逊和微软非常出色,因此它们的 PUE 接近 1。
Most colocation (colo) facilities are generally significantly worse at ~1.4+, meaning ~40% more power is lost to cooling and power transmission. Even the newest facilities for the GPU Cloud will only be around 1.25, which is significantly higher than the big clouds who also can build the datacenters cheaper due to various scale advantages. This difference is incredibly important for CPU servers, because the increased hosting costs of colo makes a large percentage of TCO. In the case of GPU servers, while hosting costs are high, it really doesn’t matter on the grand scheme of things because hosting costs are minor and server capital costs are the dominating factor in the TCO equation.
大多数共置(colo)设施的效率通常较差,约为 1.4 以上,这意味着约 40%的电力用于冷却和电力传输的损耗。即使是最新的 GPU 云设施,其效率也仅约为 1.25,这显著高于大型云服务商,因为他们由于各种规模优势能够以更低的成本建设数据中心。这个差异对 CPU 服务器来说非常重要,因为共置的增加托管成本占总拥有成本(TCO)的很大一部分。在 GPU 服务器的情况下,尽管托管成本较高,但从整体来看并不重要,因为托管成本相对较小,而服务器的资本成本在 TCO 计算中占主导地位。
A relatively poor datacenter operator can buy an Nvidia HGX H100 server with 13% interest rate debt and still come away with an all-in cost per hour of $1.525. There are many optimizations the better operators can do from here, but the capital costs are the main knob. In turn, even the most favorable GPU cloud deals are around $2 an hour per H100, and we have even seen desperate folks get fleeced for more than $3 an hour. The returns for cloud providers are tremendous….
一个相对较差的数据中心运营商可以以 13%的利率债务购买一台 Nvidia HGX H100 服务器,最终每小时的总成本为 1.525 美元。更优秀的运营商可以在此基础上进行许多优化,但资本成本是主要因素。因此,即使是最有利的 GPU 云交易,每小时每台 H100 的费用也在 2 美元左右,我们甚至看到一些绝望的人被收取超过 3 美元的费用。云服务提供商的回报是巨大的……
Of course, this is the simplified framework. Many variables can change and they radically change the costing equation. We have even seen CoreWeave try to pitch 8 year lifecycles to people, but that math is utter nonsense.
当然,这是一个简化的框架。许多变量可能会改变,并且它们会彻底改变成本计算公式。我们甚至看到 CoreWeave 试图向人们推销 8 年的生命周期,但这个数学计算完全是胡说八道。
In fact many of the assumptions in the table above are not representative of the reality of colo today. Instead we share more realistic figures below.
事实上,上表中的许多假设并不代表今天的实际情况。相反,我们在下面分享更现实的数字。
Let’s dive in and explain the simplified model more.
让我们深入探讨并进一步解释简化模型。
In reality many of these assumptions being pushed are bogus. Yes, CPU servers useful lives are ~6 years. This is due to the relative stagnation that has occurred in the CPU space. GPUs on the other hand have a very different rate of innovation.
实际上,许多被推动的假设都是虚假的。是的,CPU 服务器的有效使用寿命约为 6 年。这是由于 CPU 领域相对停滞不前。而 GPU 则有着非常不同的创新速度。
As such their useful life isn’t 6 years, but more like 4 years. The cloud rental cost decline for H100 and its relative steepness is all that matters.
因此,它们的使用寿命不是 6 年,而更像是 4 年。H100 的云租赁成本下降及其相对陡峭程度才是最重要的。
The Colocation Cost is the rental cost charged by the colocation company for physically hosting the IT equipment in the data center – importantly, it usually does not include power costs. It is usually quoted in terms of USD per kilowatt (kW) per month – this is because the cost of construction for a data center usually scales with the intended power delivery to the data hall given all the transformers, air conditioning/evaporation towers, and other equipment needed. This cost has been soaring all year. $120 does not reflect the reality of todays turbulent colo market.
共置成本是共置公司收取的在数据中心物理托管 IT 设备的租赁费用——重要的是,它通常不包括电力成本。它通常以每千瓦(kW)每月的美元(USD)报价——这是因为数据中心的建设成本通常与计划提供给数据大厅的电力交付规模相关,考虑到所有变压器、空调/蒸发塔和其他所需设备。这个成本在今年一直在飙升。120 美元并不能反映今天动荡的共置市场的现实。
Furthermore, with greenfield buildings being prepped to go all in on water-cooling and supporting >100kW racks for the upcoming B100 watercooled variant, the costs of the datacenter physical infrastructure are increasing.
此外,随着新建建筑准备全面采用水冷技术并支持超过 100kW 的机架以迎接即将推出的 B100 水冷变体,数据中心的物理基础设施成本正在上升。
The cost of capital for a business is a function of the risk-free rate (US debt rate) as well as a risk premium on top to account for the volatility and riskiness of the business. The riskier and more uncertain a business is, the higher the returns an investors should earn on their capital. For example, a defensive company like Proctor and Gamble with a low-risk premium would have a low cost of capital, while a more cyclical semiconductor company would have a higher cost of capital.
企业的资本成本是无风险利率(美国债务利率)以及额外的风险溢价的函数,以考虑企业的波动性和风险性。企业越风险越大、不确定性越高,投资者在其资本上应获得的回报就越高。例如,像宝洁这样的防御性公司由于低风险溢价,其资本成本较低,而更具周期性的半导体公司则会有更高的资本成本。
New GPU hosting clouds have some similar risks to the large cloud providers, but run the additional risks of being greenfield companies within a nascent industry with a limited track record and have very high exposure to potential cyclicality of the GPU compute market as well as the additional risk of committing capital when the cost of GPUs has already inflated significantly.
新的 GPU 托管云与大型云服务提供商存在一些相似的风险,但还面临作为新兴行业中绿地公司的额外风险,缺乏足够的业绩记录,并且对 GPU 计算市场潜在的周期性波动有很高的暴露风险,以及在 GPU 成本已经显著上涨时承诺资本的额外风险。
Stacking it all together, the true breakeven cost of a GPU cloud installing incremental H100’s today is $2.20 an hour under this simplified TCO model, with 80% of this cost from capital cost. Many GPU cloud deals are being done well below $2.20 an hour.
将所有因素综合考虑,按照这个简化的总拥有成本模型,今天在 GPU 云中安装增量 H100 的真实盈亏平衡成本为每小时 2.20 美元,其中 80%的成本来自资本成本。许多 GPU 云交易的价格远低于每小时 2.20 美元。
The picture is not pretty when a new cloud signs cloud deals for only 1 to 3 years, at elevated infrastructure hosting pricing, with massive counterparty risk associated with their random startup customer which may or may not be around long term.
当新的云服务商仅签订 1 到 3 年的云交易时,情况并不乐观,基础设施托管价格高昂,且与其随机的初创客户相关的巨大对手风险可能会影响其长期存在。
Even if the customer is a solid bluechip firm, if it is not locked in for the long term, the picture can still be quite ugly. For example, let’s say the cloud signs a 3-year deal with Salesforce. 3 years from now, B100 will have ramped fully, and X/R100 will start to ship. These newer, faster chips will mean the market price of H100’s should be much lower.
即使客户是一家稳健的蓝筹公司,如果没有长期锁定,情况仍然可能相当糟糕。例如,假设云计算与 Salesforce 签订了一份为期 3 年的合同。3 年后,B100 将完全 ramp up,而 X/R100 将开始发货。这些更新、更快的芯片将意味着 H100 的市场价格应该会低得多。
You’d be downright insane to willingly pay $2 an hour for H100s in 2026 on a new contract. The open market for a GPU cloud for H100’s in 2026 will be priced significantly lower than the current market rates.
在 2026 年,自愿以每小时 2 美元的价格为 H100 支付新合同的费用简直是疯狂的。2026 年 H100 的 GPU 云开放市场价格将显著低于当前市场价格。
It’s not all negative, the reality is that many installs are happening that are tremendously profitable. For example, take CoreWeave. They snapped up a lot of low cost colo space and have sold 5 year deals. Those will turn a handsome profit. Some of these deals have very little counterparty risk like in the case of the CoreWeave/Microsoft/OpenAI deal. Furthermore, CoreWeave has forced a lot of the early startup buyers like Inflection to pay big portions of the capital up front, significantly reducing their cost of capital, and thereby improving the economics. Not all their deals are this well choreographed though.
并非所有情况都是负面的,现实是许多安装正在进行中,且非常有利可图。例如,CoreWeave。他们抢购了大量低成本的机房空间,并签订了五年的合同。这些合同将带来可观的利润。其中一些交易几乎没有对手方风险,比如 CoreWeave/Microsoft/OpenAI 的交易。此外,CoreWeave 迫使许多早期创业买家,如 Inflection,提前支付大部分资本,显著降低了他们的资本成本,从而改善了经济效益。不过,并不是所有的交易都如此精心安排。
While the GPU clouds burn hot and bright, remember firms like Google, Microsoft, and Amazon are pure cash generating machines. This means their cost of capital is theoretically incredibly low. The cash printer gives them a natural advantage in the long term if the new GPU clouds can’t find a way to also have sustainable low cost source of capital, like for example a robust paid off existing GPU rental fleet.
尽管 GPU 云计算热火朝天,但请记住,像谷歌、微软和亚马逊这样的公司是纯粹的现金生成机器。这意味着它们的资本成本在理论上非常低。如果新的 GPU 云计算无法找到可持续的低成本资本来源,比如一个强大的、已偿还的现有 GPU 租赁车队,那么现金打印机将为它们在长期内提供自然优势。
On the flip side, the 3 giants also have a return hurdle that is very high, and so this may lead to them still having a higher hurdle than the cost of capital + return hurdle of the pureplay GPU clouds.
另一方面,这三大巨头的回报门槛也非常高,因此这可能导致它们的门槛仍高于资本成本 + 纯粹 GPU 云的回报门槛。
Google, Microsoft, and Amazon need to develop their own chips for exactly this reason. They need to reduce the capital cost side of the equation in a way these new competitors cannot, because IAaS is not a moat. If they convince users to deploy on their chips, then all of a sudden, their costs are way lower and they have a competitive edge.
谷歌、微软和亚马逊正是出于这个原因需要开发自己的芯片。他们需要以这些新竞争对手无法做到的方式降低资本成本,因为 IAaS 并不是护城河。如果他们说服用户在他们的芯片上部署,那么突然间,他们的成本就会大幅降低,从而获得竞争优势。
The GPU hosting business models bears some similarities to airlines. Aircraft are purchased from one of two companies, with the capital cost of aircraft being one of the largest cost line items, with capital being one of the only barriers to entry. Airlines also have customers that are not locked in for any period of time and have to do their best to maximize utilization and sweat expensive assets as hard as possible. Absent a cozy monopoly or oligopoly, most Airlines barely earn their cost of capital.
GPU 托管业务模型与航空公司有一些相似之处。飞机是从两家公司之一购买的,飞机的资本成本是最大的成本项目之一,资本是进入市场的唯一障碍之一。航空公司也有客户,他们没有被锁定在任何时间段内,必须尽力最大化利用率,尽可能充分利用昂贵的资产。在没有舒适的垄断或寡头垄断的情况下,大多数航空公司几乎无法赚取其资本成本。
We also have a significantly more detailed model that contains much more detail and answers many other questions. See below.
我们还有一个更详细的模型,包含更多细节并回答许多其他问题。请见下文。
The SemiAnalysis AI Cloud Total Cost of Ownership Model examines the ownership economics of AI Clouds that purchase accelerators and sell either bare metal or cloud GPU compute. It also sheds light on the likely future cost curves for AI Compute based on the capabilities of upcoming AI Accelerators as well as the impact of various optimization techniques and parallelism schemes being implemented in the market.
SemiAnalysis AI 云总拥有成本模型考察了购买加速器并出售裸金属或云 GPU 计算的 AI 云的拥有经济学。它还阐明了基于即将推出的 AI 加速器的能力以及市场上实施的各种优化技术和并行方案的影响,AI 计算的未来成本曲线。
It can be used to evaluate the business case for establishing and running an AI Cloud for various stakeholders from AI Cloud management teams to equity and debt investors, examining the economics of business operations as well as AI Accelerator residual value. It can also serve as a useful benchmarking and planning tool for customers that are currently purchasing or are considering procuring AI Compute, particularly on a long-term basis.
它可以用来评估为各种利益相关者建立和运营人工智能云的商业案例,从人工智能云管理团队到股权和债务投资者,审查商业运营的经济性以及人工智能加速器的残值。它还可以作为一个有用的基准和规划工具,供当前正在购买或考虑长期采购人工智能计算的客户使用。
The AI Cloud Total Cost of Ownership model incorporates the below topics and analyses:
AI 云总拥有成本模型包含以下主题和分析:
Historical and future rental price analysis and estimates for a variety of GPUs incorporating the following:
历史和未来租金价格分析及多种 GPU 的估算,包括以下内容:Detailed install base by GPU projections through 2028, estimated GPU total unit shipments by major vendor through 2034.
到 2028 年的详细 GPU 安装基础预测,预计主要供应商到 2034 年的 GPU 总出货量。Inference throughput, Training throughput, GPU TDP, All-in TDP per GPU, cost of ownership ($/hr), Inference Cost per M tokens, Training Cost per FLOP by accelerator including Nvidia, AMD, Intel, and custom accelerators.
推理吞吐量,训练吞吐量,GPU TDP,每个 GPU 的总 TDP,拥有成本($/小时),每百万个令牌的推理成本,按加速器计算的每 FLOP 训练成本,包括 Nvidia、AMD、Intel 和定制加速器。Market-wide inference and training throughput, most advanced inference and training cost ($/M tokens), market average training cost ($/hr per PFLOP).
市场整体推理和训练吞吐量,最先进的推理和训练成本($/M tokens),市场平均训练成本($/小时每 PFLOP)。Analysis of impact of various optimizations and parallelism schemes (Pipeline Parallel, Tensor Parallel, Expert Parallel, Data Parallel) on GPU inference and training throughput.
各种优化和并行方案(管道并行、张量并行、专家并行、数据并行)对 GPU 推理和训练吞吐量影响的分析。Future GPU rental price scenario analysis based on supply-demand analysis and estimates and incorporating evolution of cost curve over time given future GPU capabilities.
基于供需分析和估算的未来 GPU 租赁价格情景分析,并结合未来 GPU 能力下成本曲线随时间演变的情况。
GPU Total Cost of Ownership analysis, calculating comprehensive cost of operating GPU servers ($/hr) based on upfront server capex, system power consumption, colocation and electricity costs, costs of capital.
GPU 总拥有成本分析,基于前期服务器资本支出、系统功耗、联合托管和电力成本、资本成本计算 GPU 服务器的综合运营成本($/小时)。Returns and Residual value analysis including the following:
收益和残值分析,包括以下内容:Net present value and residual value analysis for a GPU cluster based on future earnings and cash generation power.
基于未来收益和现金生成能力的 GPU 集群净现值和残值分析。Cumulative project and equity cash flow.
累计项目和股权现金流。Equity and project IRR, return on assets, return on invested capital, return on equity, EBIT, EBITDA.
股权和项目内部收益率,资产回报率,投资资本回报率,股本回报率,息税前利润,息税折旧摊销前利润。
AI Cloud Full Financial Model incorporating the following elements:
AI 云全财务模型,包含以下元素:Three statement financial model – Income Statement, Balance Sheet, Cash flow, including all key balance sheet items – server depreciation, unearned/prepaid revenue, borrowings and more.
三表财务模型 - 收入表、资产负债表、现金流量表,包括所有关键资产负债表项目 - 服务器折旧、未赚取/预收收入、借款等。Support for key financial assumptions: various capital structures and mix of debt/equity, mix of cash and PIK interest, accounting depreciation period, colocation, electricity, annual maintenance contracts, sales and marketing costs, customer fixed price and fixed price duration, customer prepay assumptions, physical GPU operating lifetime/endurance, repairs and maintenance, tax expense and more.
对关键财务假设的支持:各种资本结构和债务/股权组合、现金和 PIK 利息的组合、会计折旧期、共址、电力、年度维护合同、销售和营销成本、客户固定价格和固定价格期限、客户预付款假设、物理 GPU 的操作寿命/耐用性、维修和维护、税费等。Overview of current market GPU rental prices and pricing variation.
当前市场 GPU 租赁价格及价格变动概述。LLM training and inference economics analysis, pricing trends and inference company profitability estimates.
LLM 培训和推理经济分析、定价趋势和推理公司盈利能力估计。
The model will also include one year of quarterly updates for additional features and improvements, an initial call with SemiAnalysis to explain the model and methodologies employed, as well as subsequent ad-hoc calls to answer any questions that arise from the use of the models. Contact us at Sales@SemiAnalysis.com for more details.
该模型还将包括一年的季度更新,以提供额外的功能和改进,首次与 SemiAnalysis 的电话会议以解释所采用的模型和方法论,以及后续的临时电话会议以回答使用模型过程中出现的任何问题。有关更多详细信息,请联系我们:Sales@SemiAnalysis.com。
Insightful analysis thank you.
深刻的分析,谢谢。
A key crucial lever to GPU economics is utilization (%). Your charts use the figure 80%. How accurate is that number across vendors? I've heard of ranges between 60%-80%. There seem to be two types of utilization - economic utilization which is (%) of GPU compute that is paid for, vs compute utilization which is (%) of GPU compute being effectively used. A lot of people buying out a year of reserved instances with compute utilization 50% or less. Would appreciate your insights.
GPU 经济学的一个关键杠杆是利用率(%)。你的图表使用了 80%的数字。这个数字在不同供应商之间的准确性如何?我听说过 60%-80%的范围。似乎有两种类型的利用率——经济利用率是指支付的 GPU 计算的(%),而计算利用率是指有效使用的 GPU 计算的(%)。很多人购买了一年的预留实例,但计算利用率在 50%或更低。希望能得到你的见解。
迪伦·帕特尔的 2 条回复
Thanks for the write-up Daniel & Dylan. What are you thoughts around increasing the Cost of capital from 13% to 18% for your "more realistic" analysis?
感谢你的写作,Daniel 和 Dylan。你们对将资本成本从 13% 提高到 18% 的“更现实”分析有什么看法?