企业中 AI 和机器学习管理的专有特色的一部分
为什么你可以信任 ZDNET 我们的流程
'ZDNET Recommends': What exactly does it mean?
ZDNET's recommendations are based on many hours of testing, research, and comparison shopping. We gather data from the best available sources, including vendor and retailer listings as well as other relevant and independent reviews sites. And we pore over customer reviews to find out what matters to real people who already own and use the products and services we’re assessing.
When you click through from our site to a retailer and buy a product or service, we may earn affiliate commissions. This helps support our work, but does not affect what we cover or how, and it does not affect the price you pay. Neither ZDNET nor the author are compensated for these independent reviews. Indeed, we follow strict guidelines that ensure our editorial content is never influenced by advertisers.
ZDNET's editorial team writes on behalf of you, our reader. Our goal is to deliver the most accurate information and the most knowledgeable advice possible in order to help you make smarter buying decisions on tech gear and a wide array of products and services. Our editors thoroughly review and fact-check every article to ensure that our content meets the highest standards. If we have made an error or published misleading information, we will correct or clarify the article. If you see inaccuracies in our content, please report the mistake via this form.
什么是通用人工智能?
关于创建与人类一样聪明或甚至更聪明的人工智能的过程,您需要知道的一切。
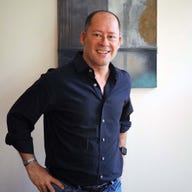
Table of Contents
目录

Yui Chino/Getty Images
什么是通用人工智能?
人工通用智能的概念已经使用了快三十年了。它通常指的是能够像人一样解决问题,甚至是比人更好的计算机系统。
术语宽泛且模糊,因此它获得了不同的含义。随着近年来生成式人工智能程序 (Gen AI) 的成功以及诸如 GPT-4 的大型语言模型的成功,一些专家尝试将 AGI 定义为能够超越单个生成式 AI 模型的狭窄问题解决能力。
然而,观察者对于通用人工智能应该具备什么功能存在分歧,这在谷歌 DeepMind 部门发布的一项概念调查中得到了明确体现。
另外:81%的工人使用 AI 后生产率更高。以下是实施方法
另一些人则认为,真正的 AGI 应该是具有“实体化”能力的,能够处理真实的物理任务。例如,苹果公司联合创始人史蒂夫·沃兹尼亚克将电脑智能测试确定为亲手煮一杯咖啡。他在 2010 年提出这个问题:“何时电脑才能达到这个水平?”但目前,今天的 AI 驱动的机器人系统还无法实现这一挑战。
另一种划分是自主意识体多大程度上应该模仿人脑。在“AGI”这一术语出现之前,哲学家约翰·塞尔就提出了类似 AGI 的理论——他称之为“强人工智能”。在塞尔看来,强人工智能程序不仅仅是完成人类所完成的任务,而是要复制人类的思维过程。
“适当地编程的计算机确实是一种心智。”塞尔写道,“计算机如果具有正确的程序,就能被真正地说成拥有理解力和其他认知状态。”
其他人则认为,如果计算机能够在测试(如艾伦·图灵著名的“模仿游戏”)中产生类似人类的行为,那么意识、知觉、思维过程和心理状态这些概念就无关紧要了。
例如,OpenAI 的成立宪章将 AGI 定义为“能够在大多数经济价值工作中超越人类的高度自主系统”,并没有提到机器的思考过程要模拟人类的思考过程。
通用人工智能何时会被发明?
除了精确定义,大多数 AI 专家都认为通用人工智能尚未存在。对于它何时可能存在的期望大相径庭,从不久的将来到永远都不可能。
DeepMind 的作者强调,通用人工智能也有不同的级别,就像自动驾驶一样,驾驶水平逐渐从定速巡航升到没有人在驾驶。
“就像采用一组标准的驾驶自动化等级允许对自动驾驶汽车的政策和进展进行清晰的讨论一样,我们提出定义'AGI 的等级'是有价值的,”他们写道。
通过这种衡量标准,作者认为今天的通用人工智能系统,如 ChatGPT,代表了“新兴的 AGI”,其能力与“没有技能的人类”相等。
从新兴的 AGI 来看,DeepMind 团队确定的等级从“胜任 AGI”、“专家 AGI”、“大师级 AGI”和“人工超智能”或“ASI”上升,从相当于中等技能成人的能力上升到超过人类 100%的能力。
同样:雇主想要 AI 技能还是 AI 增强技能?
然而,很多观察者并没有依赖于这种分级定义,而是假定存在一个临界点或者阈值,在这个阈值上,人工智能将变得与人类的能力质上等同甚至超过人类。
一位坚定支持断崖式脱钩的倡导者是谷歌的工程总监雷·库兹韦尔。在他的最近出版的书《奇点临近》中,库兹韦尔重申了他对 AGI 将在 2029 年到来的判断。库兹韦尔定义 AGI 为“可以执行任何受过教育的人类可以完成的认知任务的人工智能”。2029 年,他声称,AGI 将通过一个“健壮版”的图灵测试。
库兹韦尔警告说,一些人类智能水平的能力需要更长的时间才能实现。“对于 AI 最终将最难掌握的哪些技能仍是一个开放性问题,”他写道。
例如,到 2034 年,人工智能可能能够创作出格莱美奖得主歌曲,但不能创作出奥斯卡奖得主剧本,并且在数学上可能能够解决千禧年奖的难题,但不能产生深刻的新哲理洞察。
有些人甚至预测 AGI 的出现会更早。曾经在 OpenAI 工作过的 Leopold Aschenbrenner 写过一篇论文,根据 OpenAI 的 GPT 模型在解决问题上的进步,他认为 AGI 在 2027 年出现的可能性“非常大”。据他声称,这些模型现在在解决问题的能力上与“聪明”的高中生相当。
到二零二五年/二六年,这些机器将超越大学毕业生,”阿申伯格预测道,“到本世纪末,它们将比我们更聪明;我们将拥有真正的超智能。
另外,首席人工智能官具体是做什么的?你如何成为首席人工智能官?
AI 批评人士 Gary Marcus 认为,当前的 AI 路径,包括大型语言模型,都不可能通向 AGI。“大型语言模型不是 AGI,而且(单靠它们)永远不会是,”Marcus 在 2024 年 6 月写道,“仅靠规模从来都不足以实现这一目标。”
在其他地方,马库斯宣布当前的“基础模型”,例如 GPT-4,只是“更像是一种小把戏,而不是真正的智能。它们有些时候表现得令人印象深刻,但也有时会失败,失败的方式是混乱的、无系统的,甚至有些愚蠢。”
在最近的一篇 Substack 中,马库斯指出,当今通用人工智能的错误方法,通过引用 OpenAI 首席技术官米拉·穆拉蒂在最近一次会议上发表的言论。穆拉蒂被引述说,OpenAI 内部正在开发的 AI 模型“并不比现在存在的情况领先太多”。
另一位同样对 AGI 的到来持怀疑态度的观察者是 Meta Properties 的首席科学家Yann勒布朗,在 2022 年对 ZDNET 的采访中,勒布朗宣称大多数当今的 AI 方法永远不会导致真正的智能,正如他所理解的那样。
“我们看到很多关于我们应该做什么来推进到达人类级 AI 的声明,”LeCun 说,“我觉得有些想法是南辕北辙的。”
“我们还没有达到智能机器拥有与猫一样常识的地步,”LeCun 观察到,“那我们为何不从那里开始呢?”
人工智能可以做些什么?
考虑到对 AGI 和划分的多种定义,因此关于 AGI 将是什么样子、以及它何时到达或被创造,也做出了许多预测。
在他们的调查中,谷歌 DeepMind 的学者同意不需要模仿人类思维过程的 AGI 定义。与 Searle 的强人工智能观点不同,Searle 认为 AGI 会像人脑一样有想法,DeepMind 的学者则主张能力,即 AGI 的实用成果或输出。
“这种对能力的关注意味着 AGI 系统不一定必须以类似人类的方式思考或理解(因为这集中在过程上),”Ringel Morris 及其同事写道。
“同样地,拥有意识(主观意识)等特质的系统,对于 AGI 来说并不是必要先决条件。”
库兹韦尔在书中也强调了这一点,他指出 AGI 应该复制人类大脑的能力,并不一定非要遵循大脑的过程。
还有: 成为 AI 大师,即使没有技术技能?为什么复杂系统需要多样化的学习
例如,AGI 将表现出类似于人类新皮层的创造力,这是人类大脑最年轻的部分,库兹韦尔认为。新皮层负责高级认知功能,如类比推理,以及在语言和图像等多个领域的操作能力。
特别是库兹韦尔认为,人工智能的顶峰在于复制新皮质中存在的一系列抽象层次。
“就像在硅硬件上运行的人工神经网络一样,大脑中的神经网络使用分层结构将原始数据输入(人类的情况下是感官信号)和输出(对于人类而言是行为)分离开来,”库兹韦尔写道,“这种结构允许逐渐提高抽象程度,最终形成我们所认识的人类认知的微妙形式。”
库兹韦尔也描述了今天 AI 系统中的多个“缺陷”,这些缺陷可以由 AGI 解决:“上下文记忆、常识和社交互动”。
例如,一个能理解对话中出现的所有话题的程序,换句话说,是一个很长的上下文,库兹韦尔声称它还具有“创作出情节连贯、逻辑清晰的小说”的能力。
为了弥合当前通用 AI 的差距,库兹韦尔认为,今天大型语言模型令人印象深刻的语言能力需要大幅提高。“如今,AI 在高效理解语言方面的有限能力成为其整体知识的瓶颈,”他宣称。
另外:超越编程:AI 催生下一代职业角色
与库兹韦尔的乐观说法相反,批评者马克斯有时会将 AGI 描述为他认为当前方法永远无法实现的东西。
“到 2029 年,AI 将无法观看电影并准确地告诉你正在发生什么 […] 角色是谁?他们的冲突和动机是什么?等等。”
然而,马库斯也提供了对 AGI 的正面定义。例如,在 2022 年,他在 X 上写道,“我个人将其作为任何灵活通用(可能有很多)智能的简略表达,这种智能在资源利用和可靠性方面与(或超过)人类智能相匹敌。”
马库斯强调的“灵活性”意味着 AGI 不仅要完成普通人可以完成的许多任务,还应该能够获取新的任务解决能力,一种“元”的能力。许多观察者,包括库兹韦尔都认同,一般智能的学习方面是 AGI 的关键元素。
不过,其他学者从传统的人类过程的角度来阐述 AGI 的讨论,与塞尔的做法类似。例如,纽约大学哲学教授大卫·查默斯提出,意识是人工智能发展的基本问题之一。
查尔默斯认为,“未来十年左右,我们可能在其中一些大型语言模型系统中具有‘大于 20%的可能性的意识’。”
你会如何创造一个人工通用智能?
创建 AGI 大致可以分为两大类:坚持当前的 AI 方法并将其扩展到更大的规模,或者开辟新的方向,这些方向还没有被充分探索。
占主导地位的人工智能形式是机器学习领域中的“深度学习”领域,其中神经网络在大型数据集上进行了训练。考虑到这种方法所取得的进步,例如 OpenAI 语言模型从 GPT-1 到 GPT-2 到 GPT-3 和 GPT-4 的进展,许多人认为我们应该坚持下去。
例如,库兹韦尔将 AGI 视为大型语言模型近期进展的延伸,比如谷歌的伽利略。“增加这些模型的规模,使其越来越接近人类大脑的复杂性,是这些趋势的关键驱动力,”他写道。
对库兹韦尔来说,扩大现有 AI 的规模就像著名的摩尔定律这一半导体法则,用它来表示芯片的逐渐增强。库兹韦尔写道,摩尔定律的进展是库兹韦尔提出的更广泛概念“加速回报”的一个实例。对通用人工智能的进展,库兹韦尔宣称,它比摩尔定律的速度更快,因为有智能算法的缘故。
就像 OpenAI 的 DALL*E 这样的程序,能够从头开始生成一幅图像,这是库兹韦尔眼中的人类创造力的开始。用文字描述一幅从未见过的图像,比如,“一个鸡尾酒杯正在和一块餐巾做爱”,将会促使程序生成一张原创的图片。
还有:生成式人工智能是 IT 部门感到压力最大的技术
库兹韦尔将这种图像生成视为“零次学习”的一个例子,即训练有素的 AI 模型可以生成其训练数据中不存在的输出。“零次学习是类比思维和智能本身的精髓,”库兹韦尔写道。
“这种创造力将改变最近看似只属于人类的创意领域,”他写道。
但是,神经网络必须从特定,狭窄的任务,如输出句子,发展到更大的灵活性,并具备处理多个任务的能力。谷歌的 DeepMind 部门在 2022 年创建了此类灵活 AI 模型的粗糙草稿,即 Gato 模型,随后在同年发布了另一个更灵活的模型 PaLM。
库兹韦尔认为,更大的模型最终甚至会在他目前认为通用人工智能不足的领域取得进展,比如“世界建模”,在这种情况下,AI 模型有一个“关于现实世界如何运行的稳健模型”。他坚持认为,这种能力将使 AGI 表现出常识。
库兹韦尔坚持认为,并不那么重要机器是如何到达人类行为的方式,只要输出是正确的。
“如果不同的计算过程导致未来 AI 产生突破性科学发现或写出令人动容的小说,我们为什么应该关心它们是如何产生的呢?”他写道。
重申一次,DeepMind 的调查报告作者们强调,强 AI 的发展是一个不断推进的过程,会达到不同的水平,而不是库兹韦尔暗示的某个关键点。
此外:减少 ChatGPT 幻觉的 8 种方式
其他人对当前的路径持怀疑态度,因为今天的通用人工智能主要集中在可能有用的应用上,而不管这些应用是否具有“类似人类”的质量。
加里·马库斯认为,今天的基于神经网络的深度学习与人工智能的长期传统符号推理结合起来是必要的。这种结合将是“神经符号”推理。
马库斯并非孤军作战。一家名为 Symbolica 的由风险投资支持的初创公司最近从隐身模式中脱颖而出,倡导了一种神经符号混合体。该公司的使命宣言暗示,它将超越它认为大型语言模型的局限性。
“当前所有最先进的大型语言模型,如 ChatGPT、Claude和 Gemini,都基于相同的底层架构,”该公司表示。“因此,它们都面临着同样的局限性。”
符号主义的神经符号方法触及了上述“能力”与“过程”之间的争论的核心。符号主义的创始人认为,像逻辑学家塞尔那样,摒弃过程是错误的。
“Symbolica 的认知架构模仿了人类专家使用的多层次生成过程,”该公司声称。
另外:Meta 的首席人工智能科学家表示 ChatGPT “不是特别创新”,“没有什么革命性”
对于现状心存怀疑的还有 Meta 的 LeCun。他在最近的发言中重申了他对常规通用 AI 方法的怀疑。LeCun 在 X 上的一篇帖子中提到了 Anthropic 的Claude未能解决一个基本推理问题。
相反,LeCun 主张消除依赖于测量概率分布的 AI 模型,几乎所有大型语言模型和相关多模态模型都依赖于此。
相反,LeCun 鼓励发展所谓的能量基模型,这类模型借鉴了统计物理学的概念 。他认为,这些模型 可以通往“抽象预测”的道路 ,使 AI 能够建立统一的、具备规划多阶段任务能力的世界模型。
此外:Meta 的 AI 领袖 LeCun 探索深度学习的能源前沿
查尔默斯认为,“未来十年左右,我们可能在其中一些大型语言模型系统中具有‘大于 20%的可能性的意识’。”
人工智能能战胜人类吗?
大多数关于 AGI 的理论都暗示,在某个时间点,程序不仅将与人类的认知和解决问题的能力相等,还将超越这些能力。
如前所述,DeepMind 调查作者暗示,AI 水平金字塔的最顶部,即第五级,意味着机器在任何一般智能挑战上都胜过人类。这是计算能力不断增强和数据越来越多的自然结果,正如作者所说,这是“解锁”能力的一种方式。
库兹韦尔看到了超人表现的前景。“任何一种技能都能产生清晰的性能反馈数据,都可以转化为推动人工智能超越所有人类能力的深度学习模型,”他写道。
另外:新的图灵测试:你是人类吗?
然而,库兹韦尔认为,超级人类的人工智能不一定已经掌握了所有知识,它可能只能在某些特定领域表现出色。“甚至有可能,在它掌握图灵测试中的常识社交细微差别之前,人工智能就能在自我编程方面达到超人类的水平技巧。”
因此,“我们还需要开发更复杂的方法来评估人类和机器智能相似和不同的复杂和多变的方式。”
确实,人类的现代活动,如浏览社交媒体账户,质疑了人类行为的基本概念,这意味着在 AGI 到来之前,人类的认知和智力也可能会被重新评估。
超智能是什么?
根据对 AGI 的不同定义, 对于如何在 AGI 的背景下看待人类智能也有不同的理解方式。
如前所述,DeepMind 的作者看到 AGI 层次的顶点是人工智能超级智能,机器“在任务中超越 100%的人类”。
然而,作者也提出,如果采用合适的人机界面设计, 超人工智能完全可以被设计为人类能力的补充。
“人类与人工智能交互研究的作用可以被看作是确保新的 AI 系统对人类可用并有益于人类,从而使 AI 系统成功地扩展了人类的能力(即智能增强)”他们写道。
其他人则完全当“超智能”是实指,就像前 OpenAI 员工 Aschenbrenner 所说的那样,将这个术语和机器超越人类的能力等同起来。
库兹韦尔关注的不是击败人类,而是增强人类的智力——他称之为“奇点”。
在库兹韦尔看来,奇点是一种将新皮层“延伸到云中”的扩展。
“当人类能够将新皮层直接连接到基于云的计算时,我们将解锁比有机大脑当前能自行支持的更抽象的思考的潜力,”他写道。
另外,ChatGPT 到底是怎么工作的?
一个与云相连的电子设备将仅与人类的新皮层部分相连,即它的“上层区域”,而人类认知活动的大部分仍将在人类的新皮层中进行。
这种整合可以采取多种方式,包括埃隆·马斯克支持的“神经链接”植入物。
然而,库兹韦尔预测的将会是一种更和缓的融合:"最终,脑-机接口将基本上是非侵入性的——这可能会涉及到通过血液注入大脑的无害的纳米级电极。"
一些怀疑论者认为,AGI 会分散人工智能给人类带来的好处。例如,Stability.ai 的创始人兼首席执行官 Emad Mostaque 告诉 ZDNET:“从道德上讲,我们应该把重点放在人工智能技术上,而不是放在 AGI 取代人类上,而是如何通过小型灵活的模型来增强人类。”
我们如何阻止通用 AI 违反其限制呢?
在 AGI 的众多伦理考量中,如何防止这些程序造成伤害的问题尤为突出。学者之间的争论不仅仅是关于如何防止伤害,也是关于如何定义伤害本身。
DeepMind 调查的作者强调,即使是最高等级的五级人工智能,即所谓的超人工智能,也不一定真的是“自主”的。它可能具有认知能力,但也可能因为安全原因而受到执行任务的限制。
作者将人工智能类比于用户界面设计和人机交互设计——最终是由 AGI 的创造者和社会来决定应赋予此类系统的自主权程度。
例如,考虑人工智能系统的用户界面所提供的可能性,“考虑人工智能系统的用户界面所提供的可能性,”Ringel Morris 及其团队写道。“增强的能力解锁了新的交互模式,但并不决定它们[作者强调]。相反,系统设计师和最终用户会选择人类与 AI 交互的方式。”
DeepMind 团队强调,AI 最终是有益还是有害不仅仅取决于 AGI 解锁的能力,还取决于构建在 AGI 中的交互方式。比如,与人类智能交互方式正确的设计,就可能带来人类超级智能这样的积极结果。
其他人看到了潜在的广泛而深刻的危害,对于这些问题目前还没有答案。
库兹韦尔写道:“超级智能 AI 包含一种根本不同的危险——事实上,是首要的危险。如果 AI 比其人类创造者更聪明,它可能会找到规避已经采取的预防措施的方法。不存在一种普遍的战略可以绝对克服这一点。”
同样,Gary Marcus 认为,目前没有应对可能会出现的 AGI 的计划。“如果生成式 AI 的灾难是任何迹象,自我监管就是一个笑话,”Marcus 最近在 Substack 上写道,“美国国会至今几乎没有在遏制硅谷方面取得进展,我们必须做得更好。”
企业中 AI 和机器学习管理的专有特色的一部分
为什么你可以信任 ZDNET 我们的流程
'ZDNET Recommends': What exactly does it mean?
ZDNET's recommendations are based on many hours of testing, research, and comparison shopping. We gather data from the best available sources, including vendor and retailer listings as well as other relevant and independent reviews sites. And we pore over customer reviews to find out what matters to real people who already own and use the products and services we’re assessing.
When you click through from our site to a retailer and buy a product or service, we may earn affiliate commissions. This helps support our work, but does not affect what we cover or how, and it does not affect the price you pay. Neither ZDNET nor the author are compensated for these independent reviews. Indeed, we follow strict guidelines that ensure our editorial content is never influenced by advertisers.
ZDNET's editorial team writes on behalf of you, our reader. Our goal is to deliver the most accurate information and the most knowledgeable advice possible in order to help you make smarter buying decisions on tech gear and a wide array of products and services. Our editors thoroughly review and fact-check every article to ensure that our content meets the highest standards. If we have made an error or published misleading information, we will correct or clarify the article. If you see inaccuracies in our content, please report the mistake via this form.
人工智能是什么?关于人工智能的一切

Table of Contents
目录

What is artificial intelligence?
Artificial intelligence (AI) is a concept that refers to a machine's ability to perform a task that would've previously required human intelligence. It's been around since the 1950s, and its definition has been modified over decades of research and technological advancements.
Today, AI powers self-driving cars, laptops, chatbots like ChatGPT, and image generators. So what is it, and how does it work?
The phrase AI comes from the idea that if intelligence is inherent to organic life, its existence elsewhere makes it artificial. Computer scientist Alan Turing was one of the first to explore the idea that machines could use information and logic to make decisions as people do. He coined the Turing test, which compares machine ability to human ability to see if people can detect it as artificial (convincing deepfakes are an example of AI passing the Turing test).
Basic computing systems function because programmers code them to do specific tasks. AI, on the other hand, is only possible when computers can store information, including past commands, similar to how the human brain learns by storing skills and memories. This ability makes AI systems capable of adapting and performing new skills for tasks they weren't explicitly programmed to do.
Also: ChatGPT vs. Microsoft Copilot vs. Gemini: Which is the best AI chatbot?
Some experts define intelligence as the ability to adapt, solve problems, plan, improvise in new situations, and learn new things. Though these systems aren't a replacement for human intelligence or social interaction, today's AI systems demonstrate some traits found in human intelligence, including learning, problem-solving, pattern-finding, perception, and even a limited spectrum of creativity and social awareness.
Also: The best AI image generators to try right now
Of course, an important component of human intelligence is something that AI hasn't been able to replicate yet: context. For example, Google AI lacks real-world logic and can't discern human subtleties like sarcasm and humor, as evidenced by the technology advising you to add glue to pizza sauce to help the cheese stick or use gasoline to make spaghetti spicy. These examples are lower stakes, but an AI system taking action without semantic understanding can have major consequences in the wrong situation.
How can I use AI?
AI has a slew of possible applications, many of which are now widely available in everyday life. At the consumer level, this potential includes the newly revamped Google Search, wearables, and even vacuums. The smart speakers on your mantle with Alexa or Google voice assistant built-in are also great examples of AI.
Popular AI chatbots like ChatGPT, Microsoft's Copilot, and Claude can be used for conversational questions or tasks, like breaking down concepts, drafting emails or project outlines, and even writing creative stories. But because AI models cannot tell fact from fiction, these chatbots tend to hallucinate or make things up -- where applicable, always verify the statements a chatbot makes with independent research, especially if you're unsure about the quality of its citations.
Also: How does ChatGPT work?
A major function of AI in consumer products is personalization, whether for targeted ads or biometric security. This is why your phone can distinguish your face from someone else's when you're unlocking it with Face ID, for example -- it's learned what yours looks like by referencing billions of other people's faces and matching specific data points.
On a bigger scale, marketing and content teams can use AI to streamline production, while developers write and execute code with it. AI can also exponentially increase the speed and efficiency of medical research.
What is machine learning (ML)?
Machine learning (ML) refers to the process of training a set of algorithms on large amounts of data to recognize patterns, which helps make predictions and decisions. This pattern-seeking enables systems to automate tasks they haven't been explicitly programmed to do, which is the biggest differentiator of AI from other computer science topics. This capability is what many refer to as AI, but ML is a subset of AI.
When data is structured, or organized, a system can more easily detect an anomaly -- for example, when a transaction on your credit card is from a part of the world it's not used to seeing in your activity.
Also: What is machine learning? Everything you need to know
Examples of ML include search engines, image and speech recognition, and fraud detection. Similar to Face ID, when users upload photos to Facebook, the social network's image recognition can analyze the images, recognize faces, and make recommendations to tag the friends it's identified. With time, practice, and more image data, the system hones this skill and becomes more accurate.
How does machine learning work?
Machine learning is generally split into two main categories: supervised and unsupervised learning.
Supervised learning
This common technique for teaching AI systems uses annotated data or data labeled and categorized by humans. ML systems are then fed this data to learn patterns.
Suppose you wanted to train an ML model to recognize and differentiate images of circles and squares. In that case, you'd gather a large dataset of images of circles (like photos of planets, wheels, and other circular objects) and squares (tables, whiteboards, etc.), complete with labels for what each shape is.
The algorithm would then learn from this labeled collection of images to distinguish the shapes and their characteristics: in this case, circles don't have corners, and squares have four equal-length sides. The system can then see a new image and determine the shapes.
Unsupervised learning
By contrast, unsupervised learning lets algorithms try to identify patterns in unlabeled data by looking for similarities that it can use to categorize the data.
The algorithms aren't programmed in advance to pick out specific types of data; they simply look for data with similarities that they can group -- for example, segmenting customers based on shopping behavior to target them with personalized marketing campaigns.
Also: Machine learning is going real-time: Here's why and how
Reinforcement learning
In reinforcement learning, the system is trained to maximize a reward based on input data, going through a trial-and-error process until it arrives at the best possible outcome.
Imagine training a system to play a video game. The system can receive a positive reward if it gets a higher score and a negative reward for a low score. The system learns to analyze the game and make moves, learning solely from the rewards it receives. It can eventually play by itself and learn to achieve a high score without human intervention.
Reinforcement learning is also used in research, where it can help teach autonomous robots the optimal way to behave in real-world environments. Robots learning to navigate new environments they haven't ingested data on -- like maneuvering around surprise obstacles -- is an example of more advanced ML that can be considered AI.
What are the different types of AI?
Artificial intelligence can be divided into three subcategories: narrow AI, general AI, and super AI.
What is narrow AI?
Artificial narrow intelligence (ANI) refers to intelligent systems designed or trained to carry out specific tasks or solve particular problems without being explicitly designed. This type of AI is crucial to voice assistants like Siri, Alexa, and Google Assistant.
ANI is sometimes called weak AI, as it doesn't possess general intelligence. But that doesn't mean it isn't powerful in its own right. In addition to voice assistants, image-recognition systems, technologies that respond to simple customer service requests, and tools that flag inappropriate content online are examples of ANI.
ChatGPT is also an example of ANI, as it is programmed to perform a specific task: generate text responses to prompts it's given.
What is general AI?
Artificial general intelligence (AGI), or strong AI, is still a hypothetical concept as it involves a machine understanding and autonomously performing vastly different tasks based on accumulated experience. This type of intelligence is more on the level of human intellect, as AGI systems would be able to reason and think more like people do.
Also: AI's true goal may no longer be intelligence
Like a human, AGI could potentially understand any intellectual task, think abstractly, learn from its experiences, and use that knowledge to solve new problems. Essentially, we're talking about a system or machine capable of common sense, which is currently unachievable with any available AI.
Developing a system with consciousness is still, presumably, a fair way in the distance, but it is the ultimate goal of AI research. OpenAI hints that its forthcoming GPT-5 will get us closer to AGI.
What is super AI?
Artificial superintelligence (ASI) would be a machine intelligence that surpasses all forms of human intelligence and outperforms humans in every function. A system like this wouldn't just rock humankind to its core -- it could also destroy it. If that sounds like something straight out of a science fiction novel, it's because it kind of is.
Also: Mechanics of the future: Meet the specialists assembling AI
An intelligent system that can learn and continuously improve itself is still a hypothetical concept. However, if applied effectively and ethically, the system could lead to extraordinary progress and achievements in medicine, technology, and more.
What are some recent examples of AI?
Some of the most impressive advancements in AI are the development and release of GPT 3.5 and, most recently, GPT-4o, in addition to lifelike AI avatars and deepfakes. But there have been many other revolutionary achievements in AI -- too many to include here.
Here are some of the most notable.
ChatGPT (and the GPTs)
ChatGPT is an AI chatbot capable of generating and translating natural language and answering questions. Though it's arguably the most popular AI tool, thanks to its widespread accessibility, OpenAI made significant waves in artificial intelligence by creating GPTs 1, 2, and 3 before releasing ChatGPT.
Also: 6 ways ChatGPT can make your everyday life easier
GPT stands for Generative Pre-trained Transformer, and GPT-3 was the largest language model at its 2020 launch, with 175 billion parameters. Then came GPT-3.5, which powers the free tier of ChatGPT. The largest version, GPT-4, accessible through the free version of ChatGPT, ChatGPT Plus, and Microsoft Copilot, has one trillion parameters.
Self-driving cars
Though the safety of self-driving cars is a top concern for potential users, the technology continues to advance and improve with breakthroughs in AI. These vehicles use ML algorithms to combine data from sensors and cameras to perceive their surroundings and determine the best course of action.
Also: An autonomous car that wakes up and greets you could be in your future
The autopilot feature in Tesla's electric vehicles is probably what most people think of when considering self-driving cars. But Waymo, from Google's parent company Alphabet, also makes autonomous rides -- as a driverless taxi, for example, or to deliver Uber Eats -- in San Francisco, CA, and Phoenix, AZ.
Cruise is another robotaxi service, and auto companies like Audi, GM, and Ford are also presumably working on self-driving vehicle technology.
Robotics
The achievements of Boston Dynamics stand out in the area of AI and robotics. Though we're still a long way from creating Terminator-level AI technology, watching Boston Dyanmics' hydraulic, humanoid robots use AI to navigate and respond to different terrains is impressive.
DeepMind
Google subsidiary DeepMind is an AI pioneer focusing on AGI. Though not there yet, the company made headlines in 2016 for creating AlphaGo, an AI system that beat the world's best (human) professional Go player.
Since then, DeepMind has created AlphaFold, a system that can predict the complex 3D shapes of proteins. It has also developed programs to diagnose eye diseases as effectively as top doctors.
Also: What is generative AI and why is it so popular? Here's everything you need to know
What are large language models?
A predominant example of AI is large language models (LLMs). These models use unsupervised machine learning and are trained on massive amounts of text to learn how human language works. Tech companies often scrape these texts from the internet for free to keep costs down -- they include articles, books, content from websites and forums, and more.
In the training process, LLMs process billions of words and phrases to learn patterns and relationships between them, enabling the models to generate human-like answers to prompts.
But again, keep in mind that these models are replicating common grammatical patterns and word pairings, albeit at a sophisticated level -- they aren't thinking like we do, in the sense that they cannot understand fact, logic, or common sense.
Also: AI will unleash the next level of human potential. Here's how
OpenAI's recently released GPT-4o tops the Chatbot Arena leaderboard as of now. The company's GPT-4 Turbo is considered one of the most advanced LLMs, while GPT-4 is the largest LLM at supposedly 1.78 trillion parameters. ChatGPT runs on both GPT-3.5 and GPT-4. Gemini is powered by an LLM of the same name developed by Google, and while its number of parameters hasn't been confirmed, it's estimated to be as many as 175 trillion.
Chatbot Arena Update!
— lmsys.org (@lmsysorg) June 3, 2024
1. Multilingual Arena -- four new languages (German, Spanish, Russian, Japanese).
GPT-4o is #1 in English, German, and Spanish. Gemini-1.5-Pro is #1 in Japanese, Chinese, and French. Claude-3 Opus is #1 in Russian. The competition is tight, and we need… pic.twitter.com/RlNqh0XmMM
What are neural networks?
The success of machine learning relies on neural networks. These are mathematical models whose structure and functioning are loosely based on the connections between neurons in the human brain, mimicking how they signal to one another.
Imagine a group of robots that are working together to solve a puzzle. Each is programmed to recognize a different shape or color in the puzzle pieces. A neural network is like a group of robots combining their abilities to solve the puzzle together.
Neural networks can tweak internal parameters to change what they output. Each is fed databases to learn what it should put out when presented with certain data during training.
Also: Six skills you need to become an AI prompt engineer
These networks comprise interconnected layers of algorithms that feed data into each other. Neural networks can be trained to perform specific tasks by modifying the importance attributed to data as it passes between layers. During the training of these neural networks, the weights attached to data as it passes between layers will continue to be varied until the output from the neural network is very close to what is desired.
At that point, the network will have 'learned' how to carry out a particular task. The desired output could be anything from correctly labeling fruit in an image to predicting when an elevator might fail based on its sensor data.
What is deep learning?
Deep learning is part of the ML family and involves training artificial neural networks with three or more layers to perform different tasks. These neural networks are expanded into sprawling networks with a large number of deep layers that are trained using massive amounts of data.
Deep learning models tend to have more than three layers at least and can have hundreds of layers at most. Deep learning can use supervised or unsupervised learning or both in training processes.
Also: What is deep learning? Everything you need to know
Because deep learning technology can learn to recognize complex patterns in data using AI, it is often used in natural language processing (NLP), speech recognition, and image recognition.
What is conversational AI?
Conversational AI refers to systems programmed to have conversations with a user and are trained to listen (input) and respond (output) in a conversational manner. Conversational AI uses NLP to understand and respond naturally.
Also: Why conversational AI is now ready for prime time
Some examples of conversational AI are chatbots like Gemini, smart speakers with a voice assistant like Amazon Alexa, or virtual assistants on your smartphone like Siri.
What AI services are available to use?
Consumers and businesses alike have a wealth of AI services available to expedite tasks and add convenience to day-to-day life -- you probably have something in your home that uses AI in some capacity.
Here are some common examples of AI available to the public, both free and for a cost:
- Voice assistants: Amazon Alexa on the Echo device on your shelf, Apple's Siri on your iPhone, and Google Assistant on a Pixel device all use natural language processing to understand and respond to your questions or commands.
- Chatbots: AI chatbots like ChatGPT, Copilot, and Perplexity are another form of virtual assistant that can interact with people and, in some cases, hold human-like conversations, even mimicking empathy and concern.
- Language translation: Services like Google Translate, Microsoft Translator, Amazon Translate, and ChatGPT use machine learning to translate text.
- Productivity: Microsoft Copilot for Microsoft 365 is a great example of an LLM used as an AI productivity tool embedded within Word, PowerPoint, Outlook, Excel, Teams, and more to automate tasks. For example, simply entering 'Email the team about the latest status on the project' will trigger Copilot to automatically gather information from emails and documents to generate a text with your request.
- Image and video recognition: Different programs use AI to find information about the content in images and videos, such as faces, text, and objects. Clarifai, which employs machine learning to organize unstructured data from sources, and Amazon Rekognition, an AWS service that lets users upload images to receive information, are two examples of this.
- Software development: Many developers have been using ChatGPT to write and debug code for over a year, but many other AI tools are available to make a programmer's job easier. One example is the AI pair programmer GitHub Copilot by OpenAI Codex, a generative language model that can write code faster with less effort by autocompleting comments and code instantly.
- Building a business: Many companies are creating AI tools specifically for businesses, like OpenAI's GPT-4 API, or Amazon Bedrock, a suite of cloud-based AI tools for developers.
Also: The best free AI courses (and whether AI 'micro-degrees' and certificates are worth it)
What company is leading the AI race?
With generative AI taking off, several companies are working competitively in the space -- both legacy tech firms and startups. While each is developing too quickly for there to be a static leader, here are some of the major players.
OpenAI
Unsurprisingly, OpenAI has made a huge impact in AI after making its powerful generative AI tools available for free, including ChatGPT and Dall-E 3, an AI image generator.
Also: Have 10 hours? IBM will train you in AI fundamentals - for free
Anthropic
Anthropic created Claude, a powerful group of LLMs, and is considered a primary competitor of OpenAI. The company focuses on safety and ethical concerns in its AI research.
Alphabet
Google's parent company, Alphabet, has its hands in several different AI systems through companies including DeepMind, Waymo, and Google.
Also: Ready to upskill? Look to the edge (where it's not all about AI)
Google had a rough start in the AI chatbot race with an underperforming tool called Google Bard, originally powered by LaMDA. The company then switched the LLM behind Bard twice -- the first time for PaLM 2, and then for Gemini, the LLM currently powering it. With the last change, Google also renamed the bot Bard for Gemini.
Also: What is Gemini? Everything you should know about Google's new AI model
DeepMind continues to pursue AGI. It's developed machine-learning models for Document AI, optimized the viewer experience on Youtube, made AlphaFold available for researchers worldwide, and more.
Though you may not hear of Alphabet's AI endeavors in the news every day, its work in deep learning and AI in general has the potential to change the future for human beings.
Microsoft
Aside from creating Microsoft Copilot, the company provides a suite of AI tools for developers on Azure, such as platforms for developing machine learning, data analytics, conversational AI, and customizable APIs that achieve human parity in computer vision, speech, and language.
Also: Microsoft CEO Nadella: 'Expect us to incorporate AI in every layer of the stack'
Microsoft has also invested heavily in OpenAI's development. The tech giant uses GPT-4 in Copilot, formerly known as Bing chat, and in an advanced version of Dall-E 3 to generate images through Microsoft Designer.
Apple
Apple has also entered the space most recently with its AI-upgraded line of iPads and potential new announcements to come at WWDC.
Other companies
Other firms are making strides in artificial intelligence, including Baidu, Alibaba, Cruise, Lenovo, Tesla, and more.
How will AI change the world?
At the rate and scale it's being applied, AI will impact how we work, shop, and consume media, and our privacy, our health, and more. As with most historical shifts, the benefits, downsides, and potential harms are mixed.
Every advancement in technology has changed the nature of work. By automating certain tasks, AI is transforming the day-to-day work lives of people across industries, and creating new roles (and rendering some obsolete). In creative fields, for example, generative AI reduces the cost, time, and human input to make marketing and video content.
Also: 12 reasons AI taking on more work doesn't mean it replaces you
AI is increasingly playing a role in our healthcare systems and medical research. The technology could help make care more scalable and accessible. Doctors and radiologists could make cancer diagnoses using fewer resources, spot genetic sequences related to diseases, and identify molecules that could lead to more effective medications, potentially saving countless lives.
Also: How AI hallucinations could help create life-saving antibiotics
The tech is also creating new questions about how we keep all kinds of data -- even our thoughts -- private. AI has made facial recognition and surveillance commonplace, causing many experts to advocate banning it altogether. At the same time that AI is heightening privacy and security concerns, the technology is also enabling companies to make strides in cybersecurity software.
As models -- and the companies that build them -- get more powerful, users call for more transparency around how they're created, and at what cost. The practice of companies scraping images and text from the internet to train their models has prompted a still-unfolding legal conversation around licensing creative material.
Neural networks can be used to realistically replicate someone's voice or likeness without their consent, making deepfakes and misinformation a present concern, especially for upcoming elections.
Because AI makes automation so easy on a large scale, researchers and tech employees share concerns about its role in weapons manufacturing and warfare.
Also: The ethics of generative AI: How we can harness this powerful technology
Will an AI steal your job?
The possibility of artificially intelligent systems replacing a considerable chunk of modern labor is a credible near-future possibility.
While commonplace AI won't replace all jobs, what seems certain is that AI will change the nature of work, with the only question being how rapidly and profoundly automation will alter the workplace.
Also: These are the jobs most likely to be taken over by AI
However, artificial intelligence can't run independently. While many jobs with routine, repetitive data work might be automated, workers in other jobs can use tools like generative AI to become more productive and efficient.
There is a broad range of opinions among AI experts about how quickly artificially intelligent systems will surpass human capabilities.
Also: Can AI be a team player in collaborative software development?