Designing for AI: beyond the chatbot
为人工智能设计:超越聊天机器人
Guidelines and strategies for meaningfully leveraging AI in your applications
在您的应用程序中有意义地利用人工智能的指导方针和策略
With the recent advancements in AI, we as designers, builders, and creators, face big questions about the future of applications and how people will interact with digital experiences.
随着人工智能的最近进展,我们作为设计师、建造者和创造者,面临着关于应用程序未来以及人们将如何与数字体验互动的重大问题。
Generative AI has unleashed huge possibilities with what we can do with AI. People are now using it to write articles, generate marketing and customer outreach materials, build teaching assistants, summarize large amounts of information, generate insights, etc.
生成式人工智能释放了人工智能所能做的事情的巨大可能性。人们现在使用它来写文章,生成市场营销和客户拓展材料,构建教学助手,总结大量信息,生成洞见等。
Given the lightning pace of innovation in this space, it’s not hard to imagine a future where instead of switching between various apps, users may interact with a central LLM operating system that acts as an orchestrator, where experiences will be much more ephemeral, personalized and contextual in nature.
考虑到这一领域创新的闪电速度,不难想象一个未来,用户可能不再在各种应用程序之间切换,而是与一个中央LLM操作系统进行交互,该操作系统充当协调者,其中体验将更加短暂、个性化和情境化。
The Mercury OS concept is a sneak peek into this possible future.
水星操作系统概念是对这种可能的未来的一个偷窥。
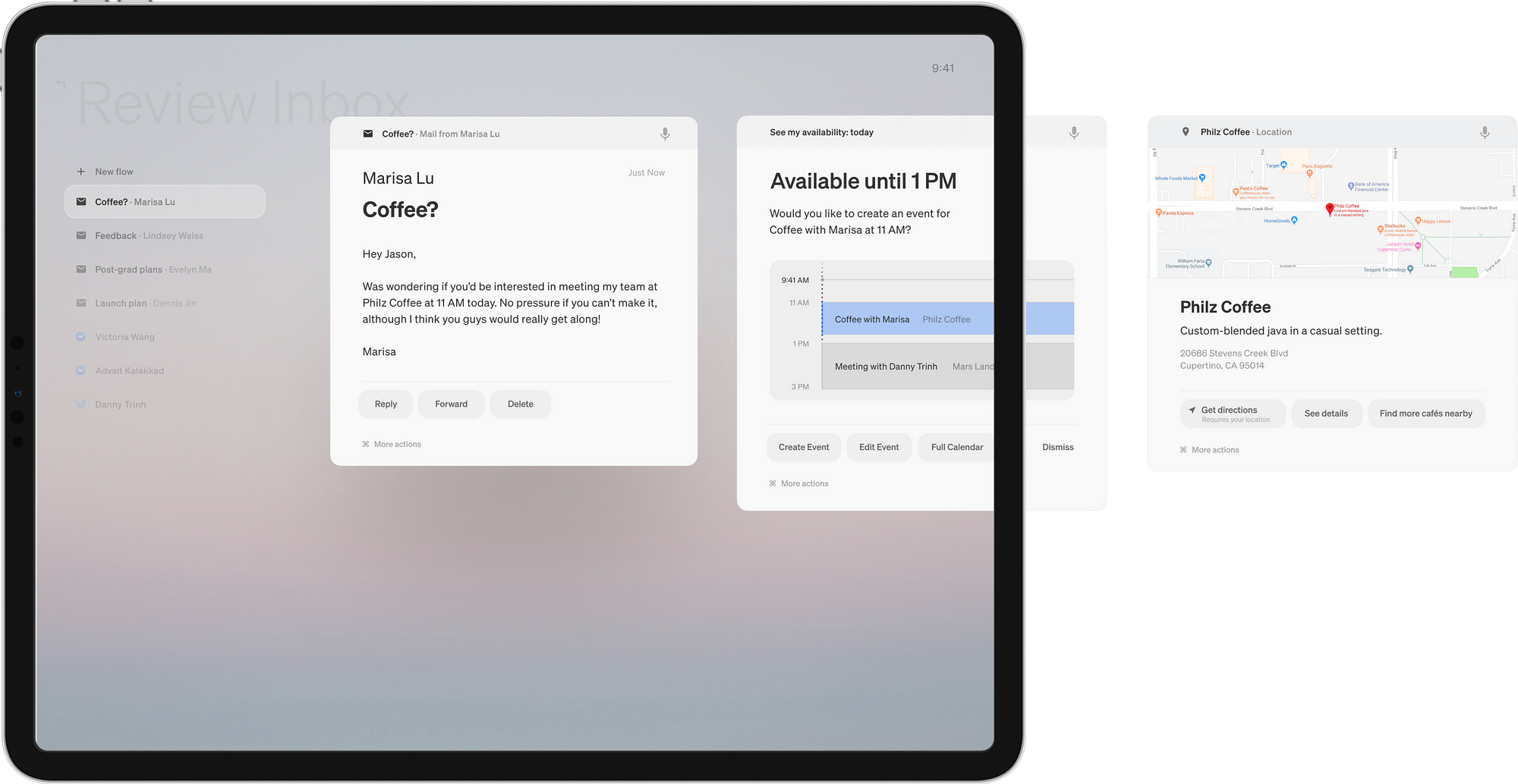
墨丘利操作系统概念,由袁杰森提出
Furthermore, we can anticipate the rise of multimodal experiences, including voice, gesture interfaces, and holographic interfaces, which will make technology more ubiquitous in our lives. Imran Chaudhri from HumaneAI recently demoed a possible screen-less future where humans interact with computers through natural language.
此外,我们可以预见多模态体验的兴起,包括语音、手势界面和全息界面,这将使技术在我们的生活中更加无处不在。HumaneAI 的 Imran Chaudhri 最近演示了一个可能的无屏幕未来,人类通过自然语言与计算机进行交互。
The possibilities for how people will interact with technology and AI in the coming years are boundless and exciting.
未来几年人们与技术及人工智能互动的可能性是无限的,令人兴奋的。

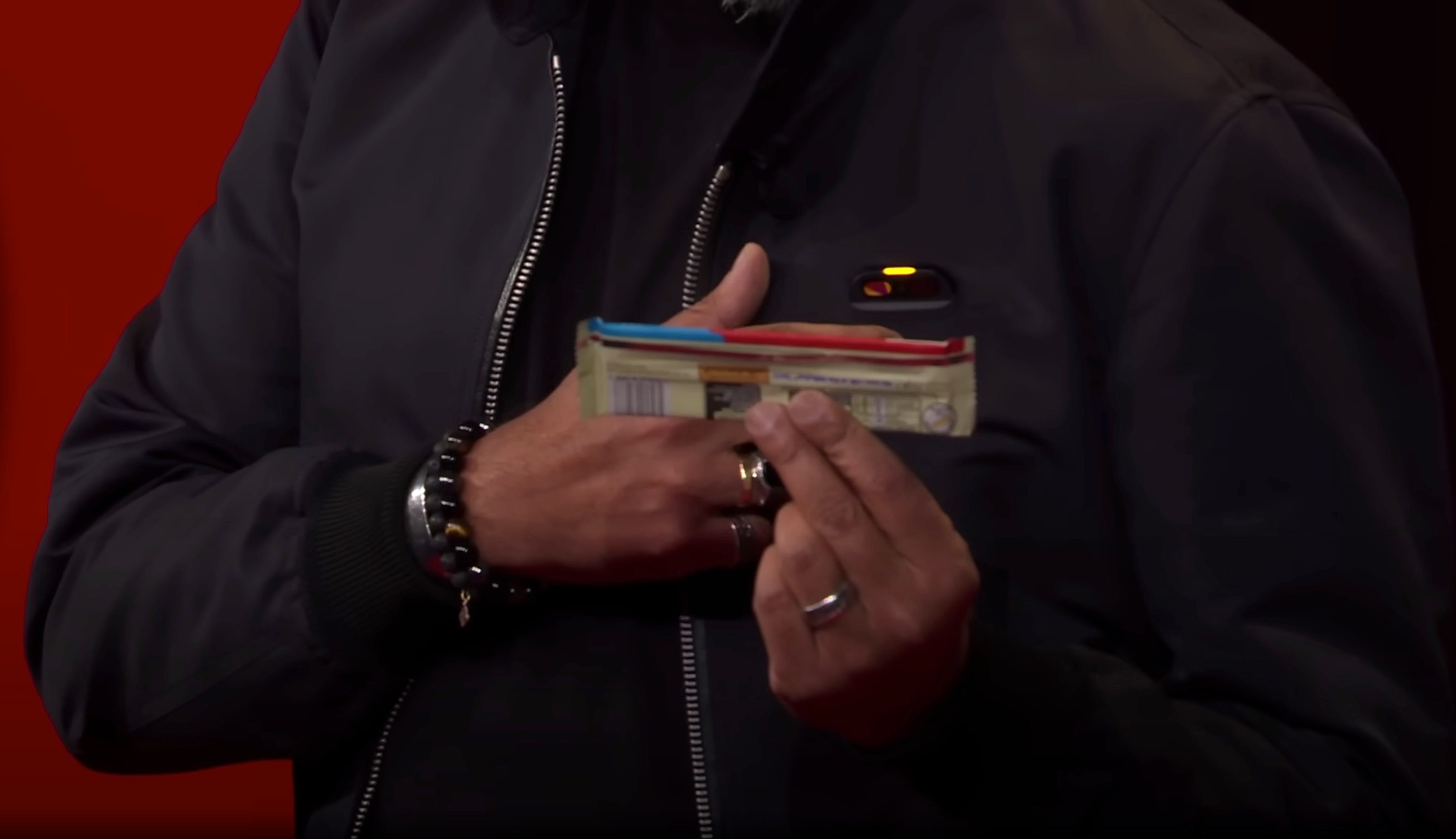
伊姆兰·乔德里在 TED 舞台上展示人文人工智能徽章概念
But coming back to the reality of the current state of AI — most businesses are still trying to figure out the best way to leverage this technology to provide value for their customers and are playing with ideas around how to explore their first integrations.
但回到人工智能当前状态的现实——大多数企业仍在努力找出利用这项技术为其客户提供价值的最佳方式,并在探索他们首次整合的想法。
Unfortunately, I see many products simply adding a free-form AI chat interface in their applications, with the hopes that people will be evoking the assistant when they need it, asking a question and hopefully getting a good response.
不幸的是,我看到许多产品只是简单地在他们的应用程序中添加了一个自由形式的人工智能聊天界面,希望人们在需要时会唤起助手,提出问题,并希望得到一个好的回应。
However, it still puts the onus on the user to switch their context, draft up a good prompt and figure out how to use the generated response (if useful) in their work.
然而,它仍然将责任放在用户身上,要求他们切换上下文,起草一个好的提示,并弄清楚如何在他们的工作中使用生成的响应(如果有用)。
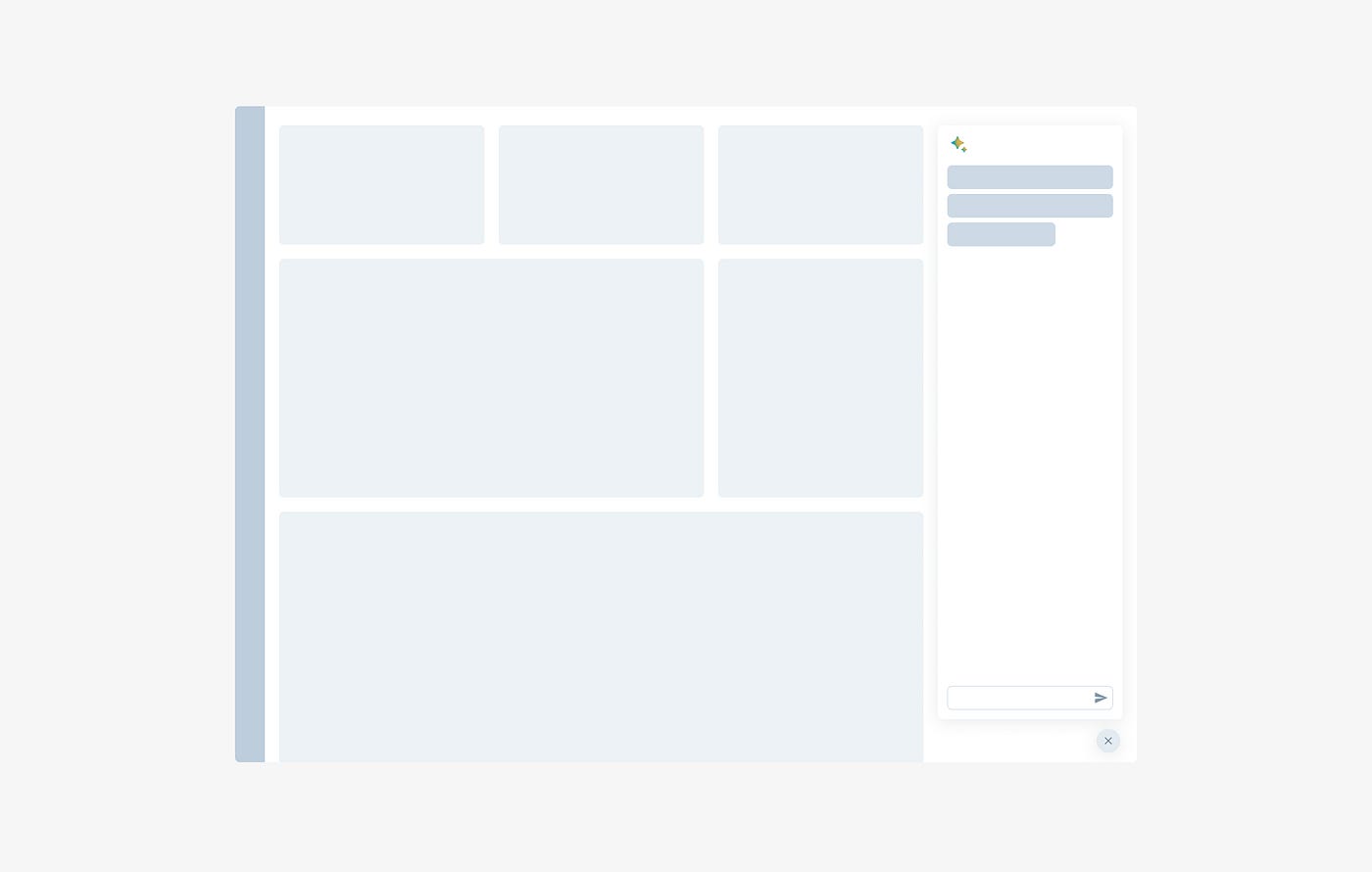
一个作为独立实体存在于应用程序内的 AI 聊天机器人
There are still a lot of unexplored territories where AI can be helpful in meaningful ways in the current state of the world.
在当前世界状态下,人工智能在许多尚未探索的领域中仍然可以以有意义的方式提供帮助。
It involves going deeper into our user’s problems, understanding the job they are trying to do, and having a keen awareness of the current possibilities and limitations of AI.
这涉及到更深入地了解我们用户的问题,理解他们试图完成的工作,并敏锐地意识到当前人工智能的可能性和局限性。
Having designed for machine learning experiences for some time now, I’ve had the opportunity to gather some strategies and best practices for meaningfully trying to integrate AI into user workflows.
经过一段时间的机器学习体验设计,我有机会收集一些策略和最佳实践,以有意义地尝试将人工智能整合到用户工作流程中。
My hope is that these strategies are useful for designers and product folks as they think about accelerating their user's workflows with AI.
我的希望是这些策略对于设计师和产品人员在考虑使用人工智能加速用户工作流程时是有用的。
#1 Identifying the right use cases and user value
#1 确定正确的用例和用户价值
照片由 Unsplash 上的 Kaleidico 拍摄
Before taking on an AI project, it’s important to take note that not every project needs AI and that AI should not be the answer to the value proposition you’re providing to your users.
在承担一个人工智能项目之前,重要的是要注意,并非每个项目都需要人工智能,人工智能也不应该是你向用户提供的价值主张的答案。
AI is merely a way to enhance your application’s value proposition by providing one or more of these benefits:
人工智能仅仅是通过提供以下一项或多项好处来增强您的应用价值主张的一种方式:
1) Reducing the time to task
1) 减少任务时间
2) Making the task easier
2) 使任务变得更容易
3) Personalizing the experience to an individual
3) 为个人定制体验
It’s also important to have a lot of clarity on what are users trying to do with your product. What does the ideal experience look like for them? What are the current limiters to that ideal experience? You can then identify which parts of that journey can be enhanced with AI.
了解用户使用您的产品时试图实现的目标也非常重要。他们理想的体验是什么样的?目前有哪些限制因素阻碍了这种理想体验?然后,您可以识别出哪些旅程的部分可以通过人工智能来增强。
Are there any parts that can be automated or made easier?
有没有可以自动化或简化的部分?
E.g. setting up automated alerts on a business dashboard, auto-categorization of emails into buckets, without having to set up rules in Gmail, and automatically detecting if there’s any suspicious activity by the home security cameras, are some good examples of where AI helps enhance the value proposition to the customer and makes their job easier.
例如,在商业仪表板上设置自动警报、自动将电子邮件分类到桶中,而无需在 Gmail 中设置规则,以及自动检测家庭安全摄像头是否有任何可疑活动,这些都是 AI 帮助增强对客户的价值主张并使他们的工作更轻松的一些好例子。
#2 Providing contextual assistance
#2 提供情境帮助
Since AI models can now understand language, context, and user patterns, they can be leveraged to offer users much more contextual suggestions, guidance, and recommendations.
由于人工智能模型现在可以理解语言、上下文和用户模式,它们可以被用来为用户提供更具上下文的建议、指导和推荐。
As an example, Grammarly Go does a good job of presenting relevant actions such as “shorten it”, “identify any gaps” etc. to users when they select a body of text. This is a great first step in providing contextual assistance.
例如,Grammarly Go 在用户选择一段文本时,很好地展示了相关操作,如“缩短它”,“识别任何空白”等。这是提供上下文帮助的重要第一步。
But we also need to take this further and think about how could we make these suggestions even more personalized and relevant for users.
但我们也需要进一步思考,如何使这些建议更加个性化和与用户相关。
E.g. in this case, if the user was sending out an email to their entire leadership chain vs a peer, what would they want to keep in mind? If a user was writing a research paper for a journal, what more information would be useful to them?
例如,在这种情况下,如果用户正在向他们的整个领导链发送电子邮件与向同行发送电子邮件相比,他们需要记住什么?如果用户正在为期刊撰写研究论文,哪些额外信息对他们有用?
Or if a user is writing a piece for the NYT, what information would be useful?
或者如果一个用户正在为《纽约时报》写一篇文章,哪些信息会是有用的?
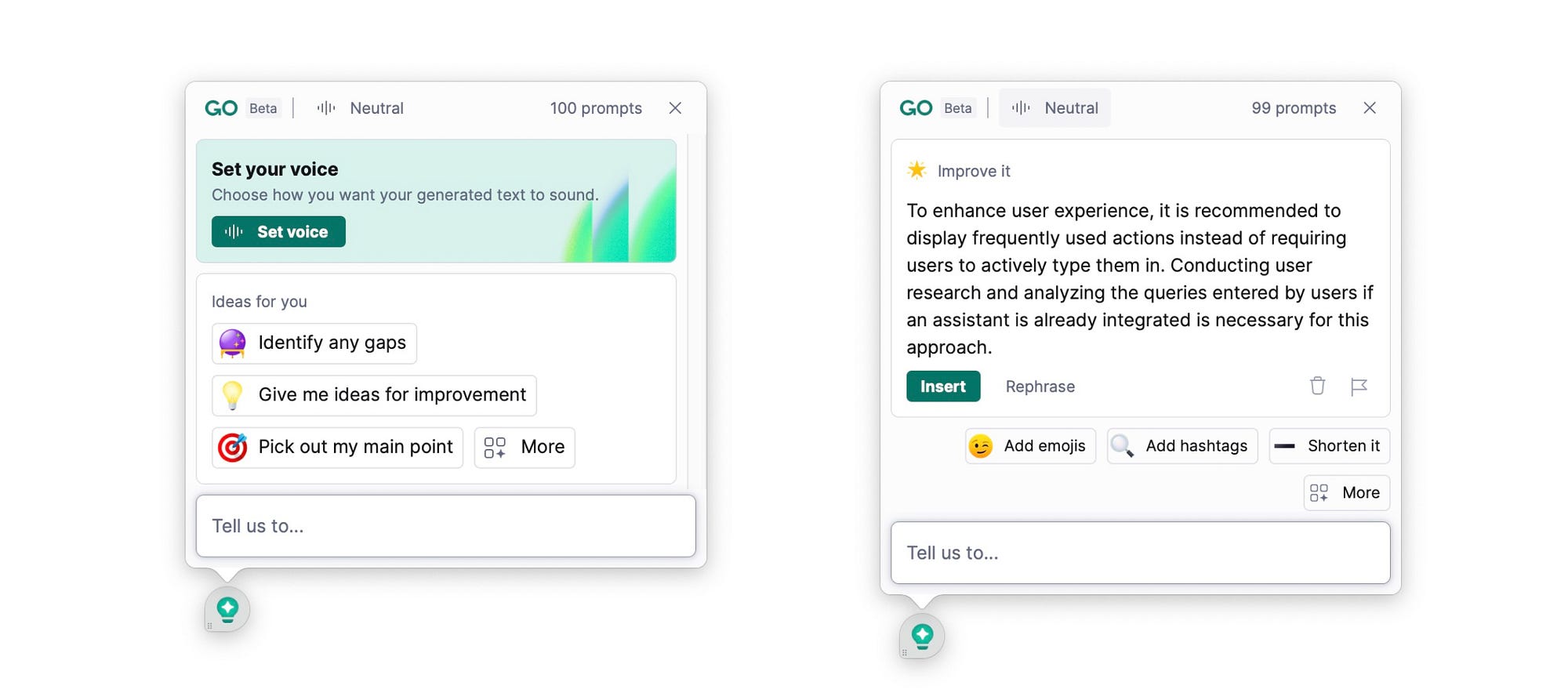
Grammarly 的上下文建议
E.g. both Notion and Coda also do a good job of recommending common actions using AI in the flow of their work, without having to shift to different contexts altogether.
例如,Notion 和 Coda 在工作流程中使用人工智能推荐常见操作方面也做得很好,无需完全切换到不同的上下文。
It makes working with AI, feel like a part of the user’s natural workflow and nicely blends with the rest of the experience, without drawing too much attention to itself.
它让与人工智能的合作感觉像是用户自然工作流程的一部分,并且很好地与其余体验融合在一起,而不会过分吸引注意力。
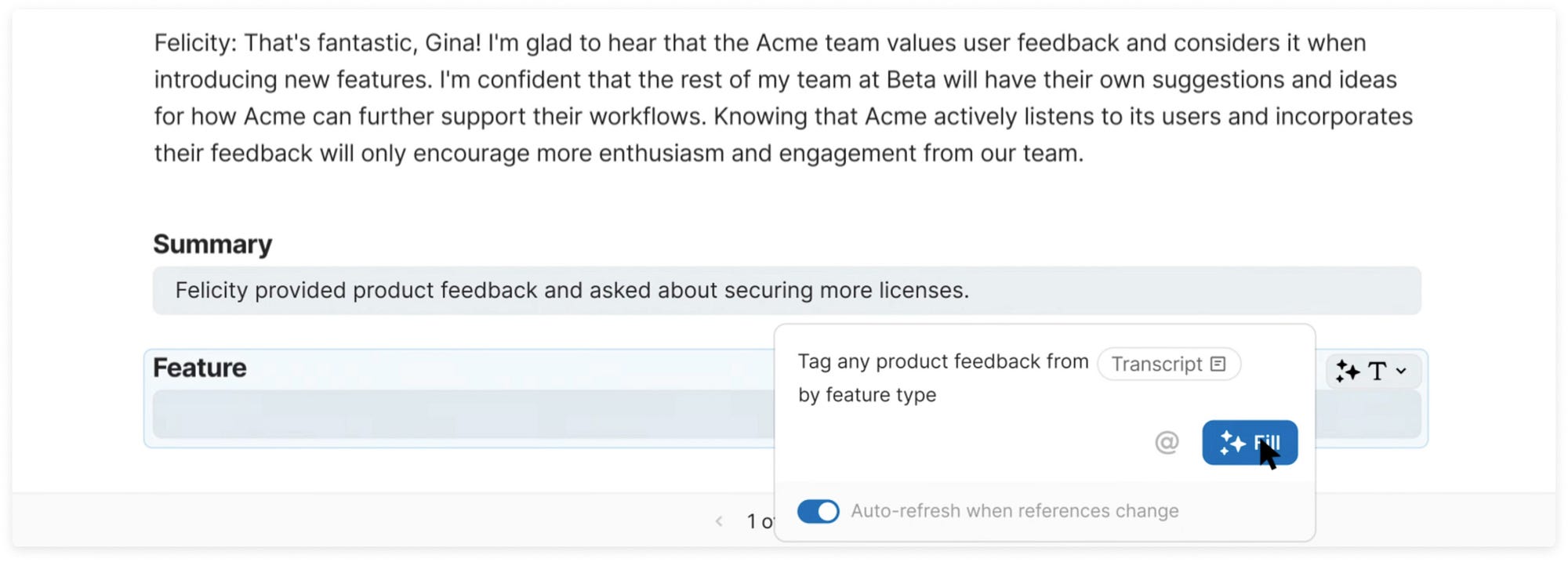
上下文LLM助手最近在 Coda 发布
Another great example of contextual integration is Github Co-pilot which again fits into the natural workflow of writing code and adds accelerators for users without interrupting their workflow and having them shift their attention to another window/part of the application to get reasonable results.
另一个上下文整合的绝佳例子是 Github Co-pilot,它再次融入了编写代码的自然工作流程,并为用户添加了加速器,而不会打断他们的工作流程,也不需要他们将注意力转移到另一个窗口/应用程序的另一部分以获得合理的结果。
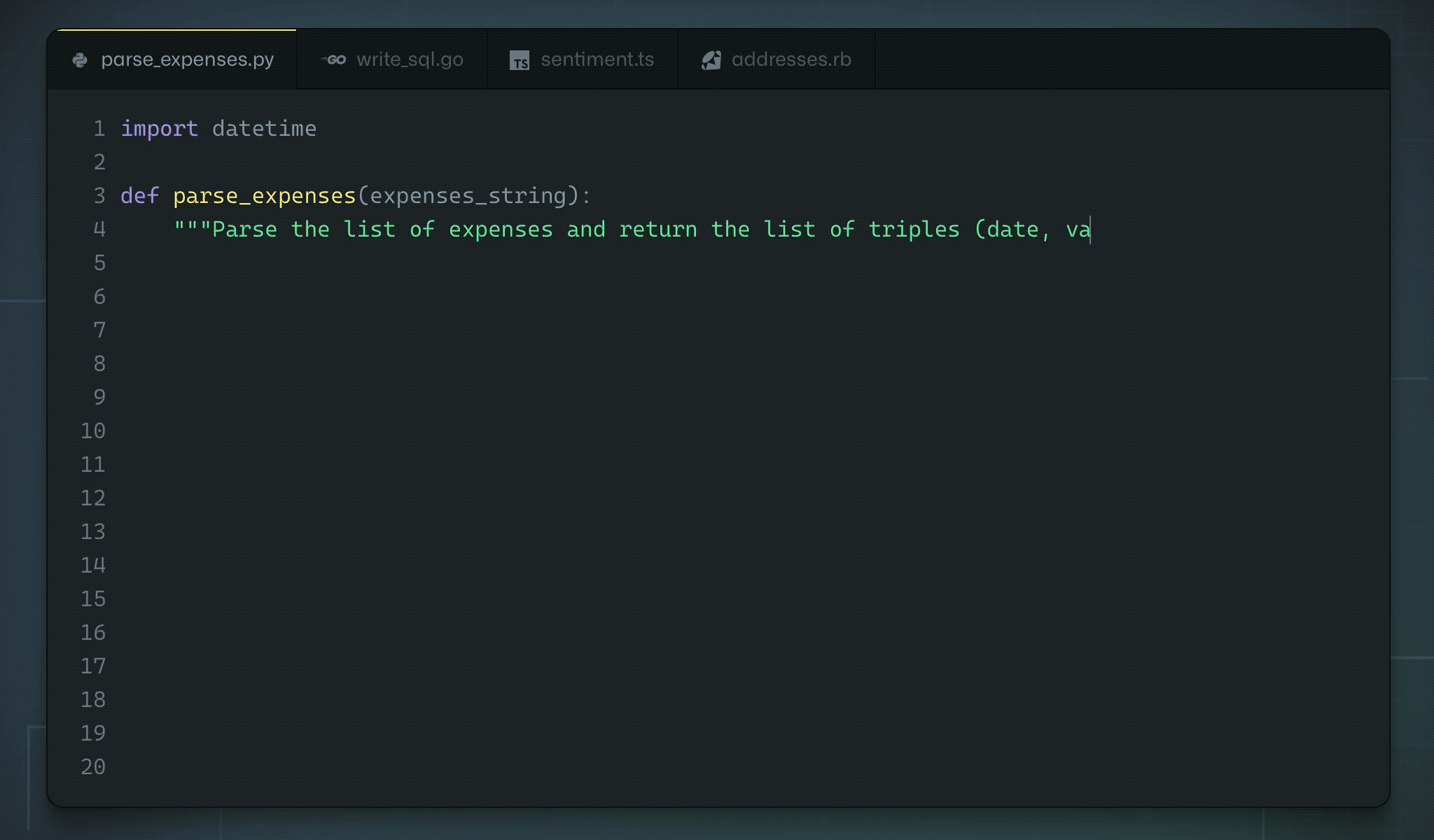
Github Co-pilot 体验。来源 Techcrunch
That being said, it’s important to also recognize the nature of assistance the user might require since not all experiences need to be fully contextual in nature.
话虽如此,重要的是也要认识到用户可能需要的援助性质,因为并非所有体验都需要完全具有情境性。
E.g. Khan Academy built out Khanmigo as an AI assistant for students to help them get unstuck and work as a teaching assistant being present in the background but available when you need it.
例如,可汗学院开发了 Khanmigo 作为学生的人工智能助手,帮助他们摆脱困境,并作为一个教学助手在后台存在,但在需要时可用。
In this case, a chatbot-like experience seems like a great start to help students, without interrupting their learning flow.
在这种情况下,类似聊天机器人的体验似乎是帮助学生而不打扰他们学习流程的一个很好的开始。
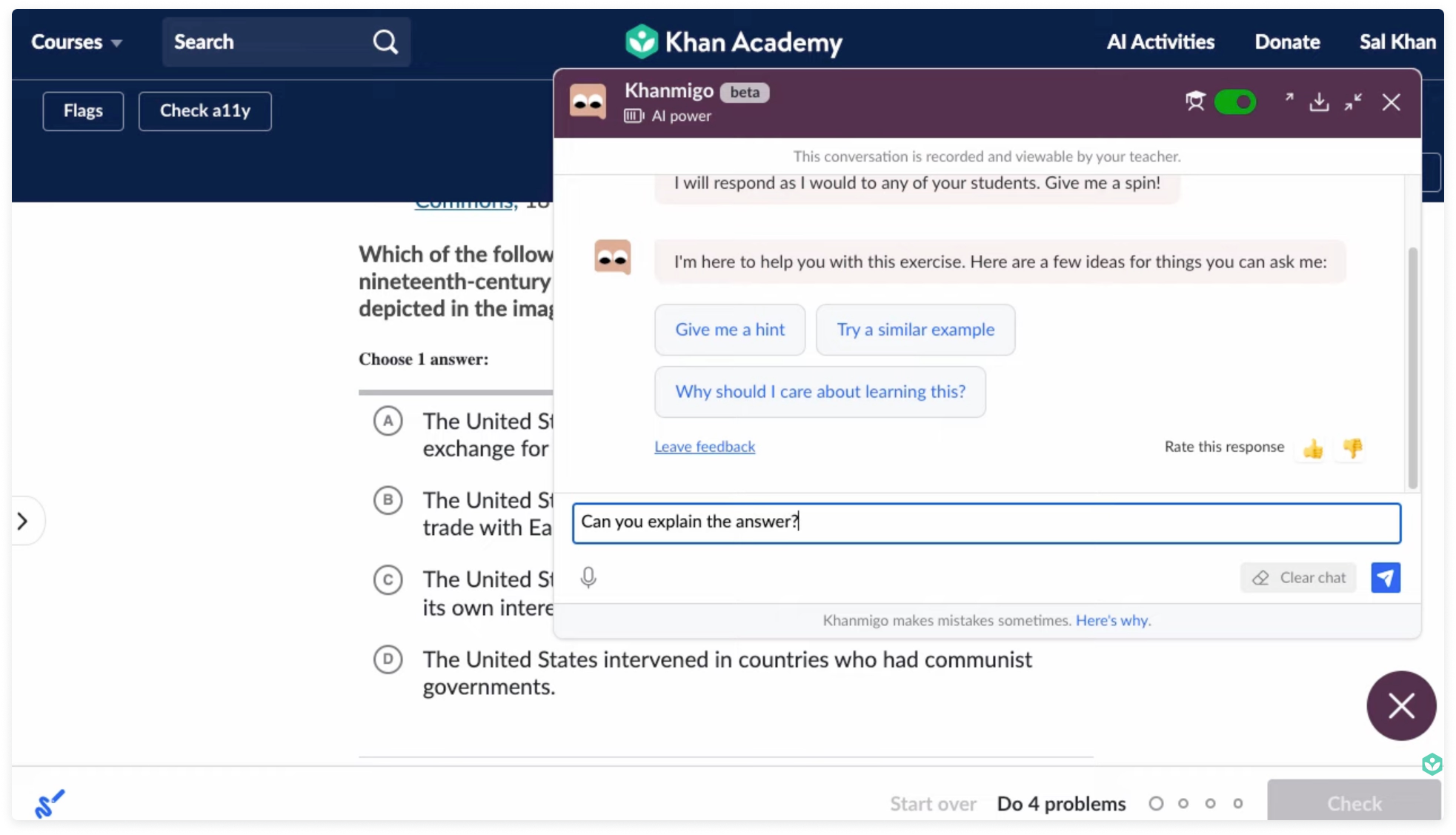
One thing to note when designing contextual experiences is that they are only useful if the AI model is aware of the user's current context and what they are working on right now or have previously worked on.
在设计情境体验时需要注意的一点是,它们只有在人工智能模型了解用户当前的情境以及他们现在或之前正在处理的工作时才有用。
Without this contextual understanding, we can only get so far in providing meaningful suggestions, recommendations, or guidance to the user.
没有这种情境理解,我们在为用户提供有意义的建议、推荐或指导方面只能走这么远。
#3 Optimizing for creativity and control
#3 优化创造力和控制
Another important thing to keep in mind when designing for creation experiences (i.e. when users are creating something like a blog, image, website, etc.) is considering the level of creativity and control users need, so they still feel control of the task the outcome.
在设计创作体验时(即当用户在创建博客、图片、网站等时),需要牢记的另一个重要事项是考虑用户所需的创造力和控制水平,以便他们仍然感觉对任务和结果有控制权。
While tools like Midjorney and Dall-E provide an incredible amount of creative expression to users, they can be limiting in terms of making edits to the generated image.
虽然像 Midjourney 和 Dall-E 这样的工具为用户提供了难以置信的创意表达能力,但在对生成的图像进行编辑方面可能会有所限制。
AI integrations for creation experiences should help users create a great starting point for their work, and give them all the tools they need to feel in control and make changes whenever needed.
人工智能集成应为创作体验提供帮助,使用户能够为他们的工作创建一个良好的起点,并提供他们需要的所有工具,以便在需要时能够控制并进行更改。
E.g. when creating a visualization using natural language, it’s important to optimize for creating a useful visualization by default, but also equally important to give users all the tools for editing/manipulating the visualization when they need it.
例如,在用自然语言创建可视化时,重要的是默认情况下优化创建有用的可视化,但同样重要的是在用户需要时提供所有工具以编辑/操作可视化。
Recent releases such as Adobe Firefly, show a promising step in this direction where the tool still gives users control over the various aspects of the image to manipulate after the image is generated.
近期发布的 Adobe Firefly 等工具,展示了这一方向上一个有希望的步骤,其中该工具在生成图像后仍然允许用户控制图像的各个方面进行操作。
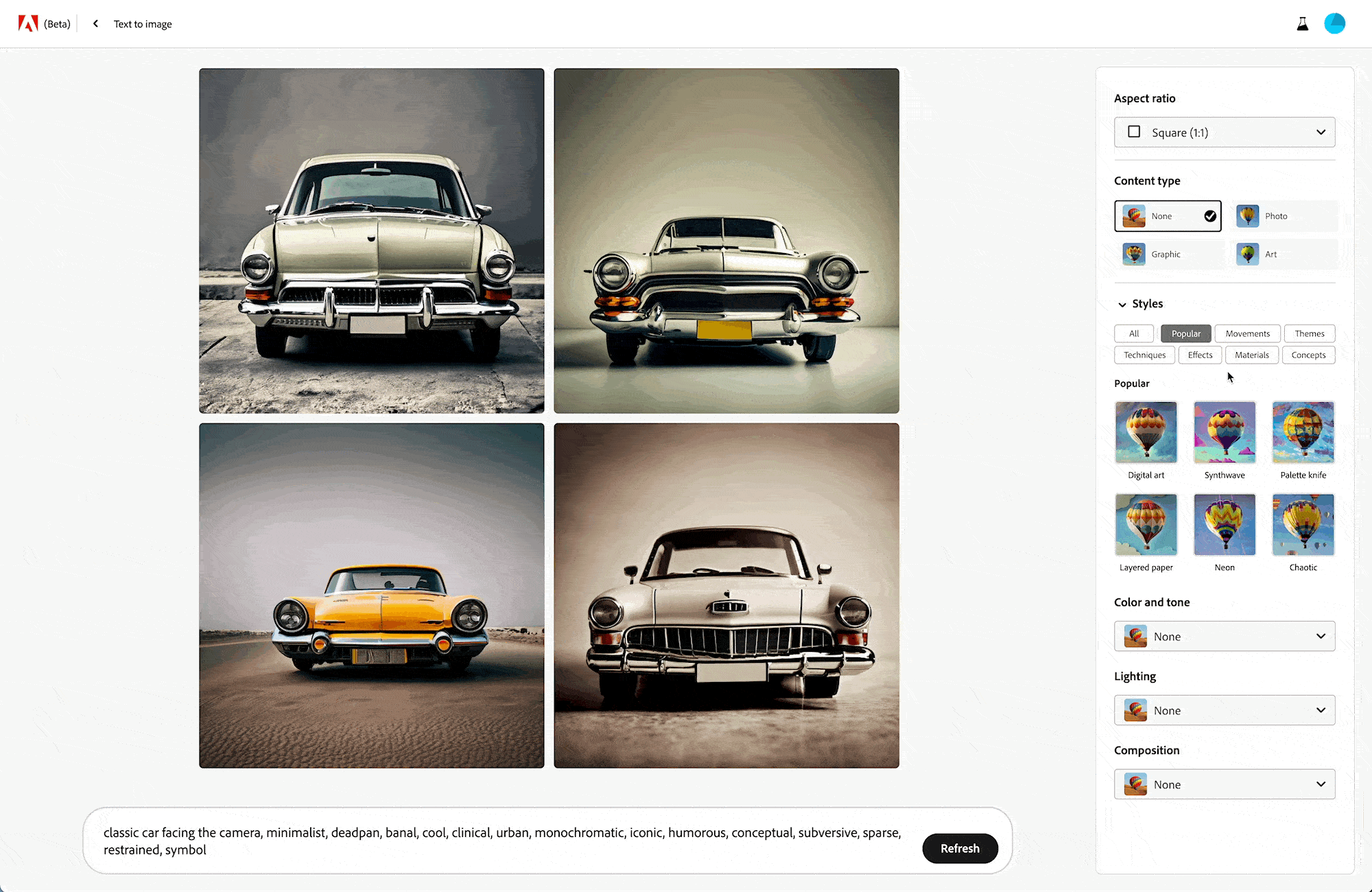
Adobe Firefly 为用户提供了一些对最终图像的控制
#4 Helping build good prompts
#4 帮助构建好的提示
Another barrier people face to getting helpful responses and making the best use of LLMs and other natural language AI models, is figuring out the right prompts to use.
人们在获得有益回应和充分利用LLMs及其他自然语言 AI 模型时面临的另一个障碍是,找出正确的提示来使用。
I see many posts and courses spring up on prompt engineering and “cheat sheets” on how to build out good prompts. There’s a need for education and awareness of what are the right ways to engage with these models to get better results, especially if the tasks are more specialized.
我看到很多关于提示工程的帖子和课程涌现,以及如何构建良好提示的“速查表”。需要教育和意识,了解如何正确地与这些模型互动以获得更好的结果,特别是如果任务更加专业化。
Some tools like Adobe Firefly present a great library of generated images and prompts when you first land on the tool. It encourages exploration of what’s possible and helps users get more ideas on building useful prompts.
一些工具,如 Adobe Firefly,在您首次使用该工具时提供了大量的生成图像和提示库。它鼓励探索可能性,并帮助用户获得更多关于构建有用提示的想法。

Adobe Firefly 中的提示和输出示例
Instead of showing various examples upfront, you can also consider leading with just a few to help people get started and later showing tips or suggestions progressively.
而不是一开始就展示各种例子,你还可以考虑先引导人们开始使用一些例子,然后逐步展示提示或建议。
E.g. when working on generating an image, DALL-E presents some prompts and tips to users to encourage learning, while they’re waiting for the result to show up.
例如,在生成图像时,DALL-E 会向用户提供一些提示和建议,以鼓励学习,同时他们正在等待结果出现。

在 DALL-E 中的提示和灵感提示
Notion too, gives suggestions to users on how they can leverage the contextual assistant for language tasks, which can help spark user’s creativity for creating good prompts.
Notion 也为用户提供了如何利用上下文助手进行语言任务的建议,这可以帮助激发用户在创建良好提示方面的创造力。

在 Notion 中的 AI 任务建议
#5 Focusing on getting quality results
#5 专注于获得高质量的结果
It’s needless to say that an AI model is only so useful if it’s able to provide good and meaningful results to users. To achieve that, it’s important to train models on datasets that are close representations of the users' actual workflows.
不言而喻,一个 AI 模型只有在能够为用户提供良好且有意义的结果时才有用。为了实现这一点,重要的是在与用户实际工作流程紧密相关的数据集上训练模型。
It’s also important that the training data covers a wide variety of use cases that are likely to occur in the real world and not just a few happy paths.
同样重要的是,训练数据应该涵盖真实世界中可能出现的多种使用情况,而不仅仅是一些理想路径。
Designers can help product teams by not only creating prototypes and demos of what’s possible with AI but also being involved in the data conversations to help identity and sometimes even gather the right training data for that use case.
设计师可以通过创建人工智能可能实现的原型和演示,不仅帮助产品团队,还可以参与数据对话,帮助识别甚至有时收集正确的训练数据以支持该用例。
Designers can also help define what good quality results would look like for users which can influence the model development process. How to handle cases when bad results are generated?
设计师还可以帮助定义对用户来说什么是高质量的结果,这可以影响模型开发过程。当生成不良结果时该如何处理?
And the types of feedback mechanisms that need to be built to understand the model performance and for improving it over time.
需要构建的反馈机制类型,以理解模型性能并随着时间提高它。
#6 Clarifying the use of AI and its limitations
#6 阐明人工智能的使用及其局限性
The challenge with AI models like LLMs is that the results can seem so trustworthy, even though they might not always be accurate.
像LLMs这样的 AI 模型的挑战在于,结果可能看起来非常可信,尽管它们可能并不总是准确的。
It’s really important to build various mechanisms to remind users of the limitations of these AI models, especially if these results could influence very important decisions for users.
建立各种机制来提醒用户这些人工智能模型的局限性非常重要,特别是如果这些结果可能影响用户的重要决策。
For E.g. interacting with AI-generated recommendations might have lower consequences in a user’s life compared to using AI to detect cancer from a medical examination result.
例如,与人工智能生成的推荐互动可能在用户生活中的后果较低,与使用人工智能从医学检查结果中检测癌症相比。
At a high level, AI will play a huge role in shaping the future of how people interact with technology.
在高层次上,人工智能将在塑造人们与技术互动的未来方面发挥巨大作用。
However, as more and more businesses continue to integrate AI into their businesses, it’s important to consider how AI can be seamlessly integrated into a user's workflow, rather than it being a separate agent, living in its own separate space.
然而,随着越来越多的企业继续将人工智能整合到他们的业务中,考虑如何将人工智能无缝地整合到用户的工作流程中,而不是作为一个独立的代理,生活在自己的独立空间中,这一点非常重要。
While designing for AI was something that only a small number of design or product folks has the opportunity to work on previously, we’re now seeing an exponential rise of teams incorporating AI into their products.
虽然之前只有少数设计或产品人员有机会从事人工智能设计,我们现在看到越来越多的团队将人工智能整合到他们的产品中。
And so it’s become even more important for us to have conversations about how to build AI products responsibly.
因此,对我们来说,就如何负责任地构建人工智能产品进行对话变得更加重要。
This is certainly a rapidly evolving space and we’ll continue to discover more of these strategies and guidelines for meaningfully interacting with AI. I’d love to know your thoughts and any other examples or guidelines that would be useful to append to this list.
这确实是一个快速发展的领域,我们将继续发现更多这些策略和指导方针,以有意义地与人工智能互动。我很想知道你的想法,以及任何其他的例子或指导方针,这些可能会对补充这个列表有用。
If you’re interested in exploring this topic in more detail, here are some resources for further reading:
如果您对深入探讨这个话题感兴趣,以下是一些进一步阅读的资源:
- “How to not add AI to your product” — a startup’s journey of trying to integrate AI into an online form builder.
“如何不在你的产品中添加 AI”——一家初创公司尝试将 AI 整合到在线表单构建器的旅程。 - “Decoding The Future: The Evolution Of Intelligent Interfaces” — an article by Rachel Kobetz on the future of digital experiences with AI.
“解码未来:智能界面的演变” —— 瑞秋·科贝茨关于人工智能数字体验未来的文章。 - “The Essential Guide to Creating an AI Product” — a comprehensive guide by Rahul Parundekar on creating an AI product, all the way from planning to implementation.
《创建人工智能产品必备指南》——拉胡尔·帕伦德卡尔撰写的全面指南,涵盖了从规划到实施的人工智能产品创建全过程。 - “Language Model Sketchbook, or Why I Hate Chatbots” — A brilliant collection of ideas by Maggie Appleton on approaches to explore with LLMs.
“语言模型素描本,或为什么我讨厌聊天机器人” —— 麦琪·阿普尔顿关于探索LLMs方法的杰出思想集。 - “What is the role of an AI Designer ”— article by Amanda Linden on how designers will play a role in AI design
“人工智能设计师的角色是什么”——阿曼达·林登关于设计师在人工智能设计中将扮演的角色的文章 - The People + AI guidebook by the People+AI Research (PAIR) team at Google
谷歌 People+AI 研究(PAIR)团队编写的《人+人工智能指南》