A Mean Reversion Strategy from First Principles Thinking
从第一原则思维出发的均值回归策略
Using a problem-solving technique to deliver 24% annual return trading S&P 500 constituents
使用问题解决技巧在交易标准普尔 500 成分股中实现 24%的年回报
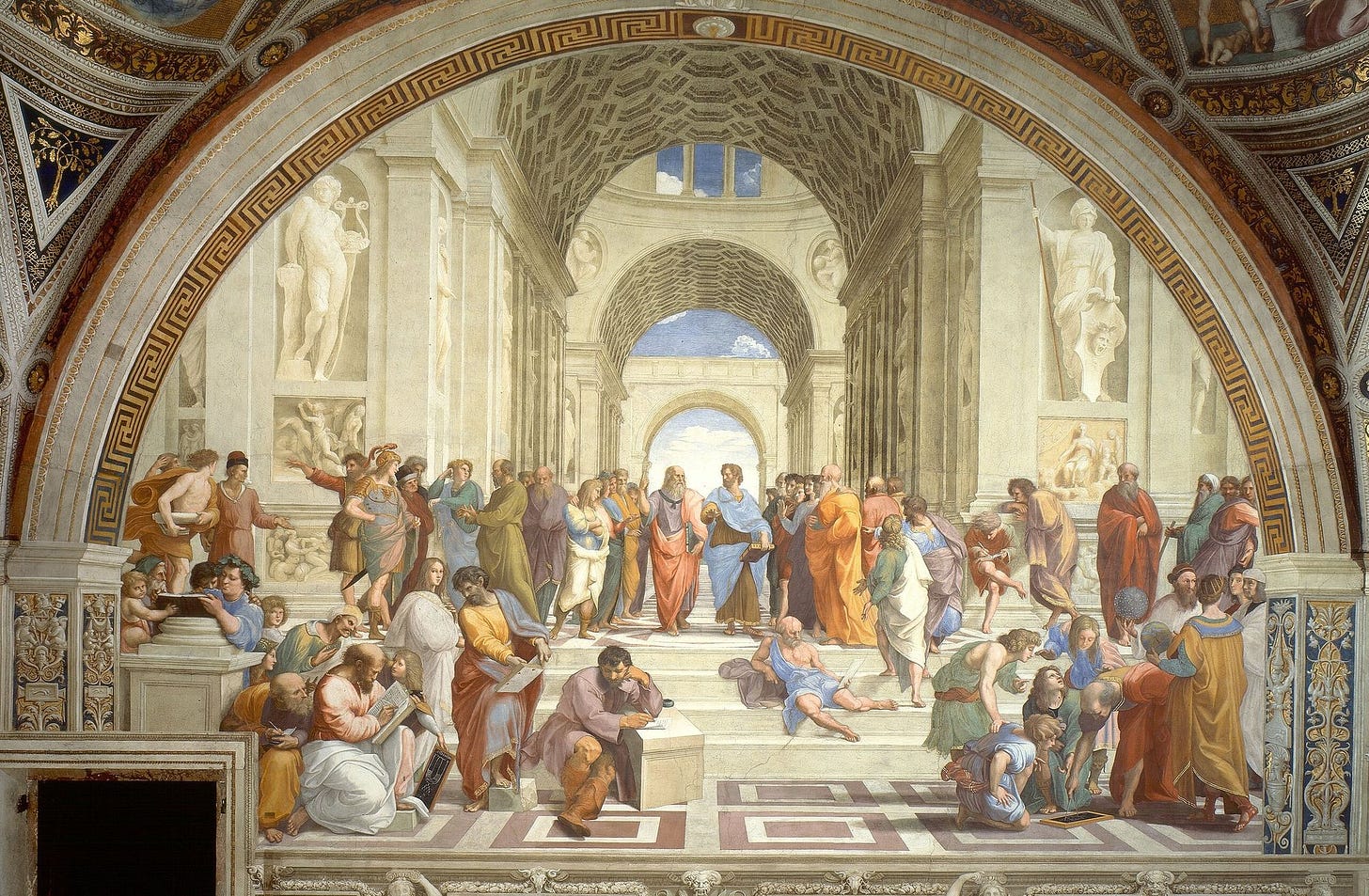
拉斐尔的《雅典学派》以柏拉图和亚里士多德为中心。亚里士多德是第一个正式阐述第一原则概念的人。
The idea 这个想法
“I think it’s important to reason from first principles rather than by analogy. The normal way we conduct our lives is we reason by analogy. [With analogy] We are doing this because it’s like something else that was done, or it is like what other people are doing. [With first principles] you boil things down to the most fundamental truths… and then reason up from there.” Elon Musk.
“我认为从第一原则进行推理比通过类比更重要。我们正常的生活方式是通过类比进行推理。[通过类比] 我们之所以这样做,是因为它像之前做过的事情,或者它像其他人正在做的事情。[通过第一原则] 你将事物归结为最基本的真理……然后从那里进行推理。”— 埃隆·马斯克
What does Elon Musk, Aristotle, Richard Feynman, Thomas Edison, Albert Einstein, Steve Jobs, Nikola Tesla, Charlie Munger, Leonardo da Vinci, Benjamin Franklin, Claude Shannon, and Rene Descartes, among other of the greatest thinkers and innovators who ever lived, had in common?
埃隆·马斯克、亚里士多德、理查德·费曼、托马斯·爱迪生、阿尔伯特·爱因斯坦、史蒂夫·乔布斯、尼古拉·特斯拉、查理·芒格、莱昂纳多·达·芬奇、本杰明·富兰克林、克劳德·香农和雷内·笛卡尔等历史上最伟大的思想家和创新者有什么共同点?
They are all known for embodying “First Principles Thinking” in their work and approach to problem-solving.
他们都以“第一性原则思维”著称,在他们的工作和解决问题的方法中体现出来。
First Principles Thinking is like creating an original song from basic notes rather than being in a cover band that plays existing songs. It involves breaking down a problem into its fundamental components and building something new from scratch instead of replicating what has already been done.
第一性原则思维就像从基本音符创建一首原创歌曲,而不是在一个演奏现有歌曲的翻唱乐队里。它涉及将问题分解为其基本组成部分,然后从头开始构建新的东西,而不是复制已经完成的内容。
First Principles Thinking can be traced back to the ancient Greek philosopher Aristotle. Elon Musk recently popularized this problem-solving technique, as he used it to reduce the cost of space travel dramatically.
第一性原则思维可以追溯到古希腊哲学家 亚里士多德。埃隆·马斯克最近普及了这一解决问题的技术,因为他利用它大幅降低了航天旅行的成本。
In general, this is the step-by-step guide on how to apply First Principles Thinking to solve problems:
总的来说,这是如何应用第一性原则思维来解决问题的逐步指南:
Identify the Problem; 识别问题;
Break Down the Problem; 拆解问题;
Challenge Assumptions; 挑战假设;
Identify First Principles;
确定第一原则;Rebuild the Problem from First Principles;
从第一原则重建问题;Test and Iterate. 测试和迭代.
This week, we will build a profitable mean reversion strategy using First Principles Thinking. Here's the plan:
本周,我们将构建一个具有盈利能力的均值回归策略使用第一性原理思维。这是计划:
First, we will deconstruct a mean reversion strategy into its basic components and question the assumption of RSI-2 as the default indicator of mean reversion in stocks;
首先,我们将将均值回归策略拆解成其基本组件并质疑 RSI-2 的假设作为股票均值回归的默认指标;Then, we will rethink what deviation from the mean is in terms of its foundational elements - probabilities - and use them as building blocks to create an indicator to trigger entry signals;
然后,我们将重新思考偏离均值在其基础要素上的含义 - 概率 - 并将其作为构建块来创建一个触发入场信号的指标;We will statistically prove that this invented indicator has an edge;
我们将统计证明这个发明的指标具有优势;Finally, we will show how this new indicator can be used to inform a strong mean reversion strategy through a series of backtests.
最后,我们将展示如何通过一系列回测使用这个新指标来指导一个强有力的均值回归策略。
Deconstructing a mean reversion strategy
解构均值回归策略
Let's break down a mean reversion strategy into smaller, more manageable parts:
让我们把均值回归策略分解成更小、更易管理的部分:
Entry trigger. Every mean reversion strategy has a set of entry rules that, when triggered, tell us to go long (or short) if the instrument's price has deviated down (or up) from the mean.
入场触发。每个均值回归策略都有一套入场规则,当触发时,告诉我们如果工具的价格偏离均值向下(或向上),则做多(或做空)。Exit trigger. Also, every mean reversion strategy has a set of exit rules that, when triggered, tell us to close the position.
出场触发。同样,每个均值回归策略都有一套出场规则,当触发时,告诉我们关闭头寸。
So far, we have been using the Relative Strength Index (RSI) to inform us when to enter mean reversion trades. To exit, we generally either also use the RSI or a simple set of rules such as:
到目前为止,我们一直使用 相对强弱指数(RSI) 来指导我们何时进入均值回归交易。为了退出,我们通常还会使用 RSI 或一些简单的规则,例如:
The closing price is higher than yesterday's high (which indicates the reversion is completed);
收盘价高于昨天的高点(这表明反转已经完成);Time (number of bars since the trade started).
时间(自交易开始以来的条数)。
The Relative Strength Index (RSI) is a momentum oscillator that measures the speed and change of price movements. It was developed by J. Welles Wilder and popularized in mean reversion strategies by the work of Larry Connors and Cesar Alvarez. Their research showed that using RSI with a short window (2 days) delivers an edge, which can be profitably exploited. Strategies based on the 2-day RSI have worked well throughout the past decades.
相对强弱指数(RSI)是一种动量振荡器,用于测量价格变化的速度和变化。它是由 J. Welles Wilder 开发的,并通过 Larry Connors 和 Cesar Alvarez 的工作在均值回归策略中得到推广。他们的研究表明使用短窗口(2 天)的 RSI 提供了一个优势,这个优势可以被盈利性地利用。基于 2 日 RSI 的策略在过去几十年中表现良好。
Usually, we take a long (or short) position whenever a stock's 2-day RSI falls below (or reaches above) a threshold such as 10 (or 90) and hold the position until the exit rule is triggered.
通常,当股票的 2 日 RSI 低于(或超过)10(或 90)等阈值时,我们会采取多头(或空头)头寸,并在退出规则被触发之前保持该头寸。
Thinking in terms of probabilities
从概率的角度思考
Is the RSI the best mean reversion indicator to inform the entry signal, or is it just an accepted norm? What is a deviation from the mean?
RSI 是最好的均值回归指标来告知入场信号,还是仅仅是一个公认的标准?什么是均值的偏差?
Let's think in terms of probabilities:
让我们 从概率的角度来思考:
Imagine a stock from a company with solid fundamentals, whose fundamentals are unlikely to change overnight;
想象一个拥有稳健基本面的公司的股票,其基本面不太可能在一夜之间发生变化;For a stock like this, we could say the larger the short-term price drop, the more probable this stock's price is to bounce back;
对于这样的股票,我们可以说 短期价格下跌的幅度越大, 这只股票的价格反弹的可能性就越高;So, the probability of an event of a price drop of that magnitude or higher is the basic element upon which we should create an indicator to inform the entry signal!
因此, 发生如此幅度或更大价格下跌事件的概率是 我们应该创建指标以告知入场信号的基本要素!
Let's illustrate that with an example. On Jan-21, 2022, Apple stock had accumulated a 3-day price drop of -4.4%. This is rare. On that day, if we looked back 5 years, this would be the 3-day returns distribution for Apple stock:
让我们用一个例子来说明。在 2022 年 1 月 21 日,苹果股票累计了 3 天的价格下跌,幅度为-4.4%。这是很少见的。在那一天,如果我们回顾过去 5 年,这将是苹果股票的 3 天收益分布:
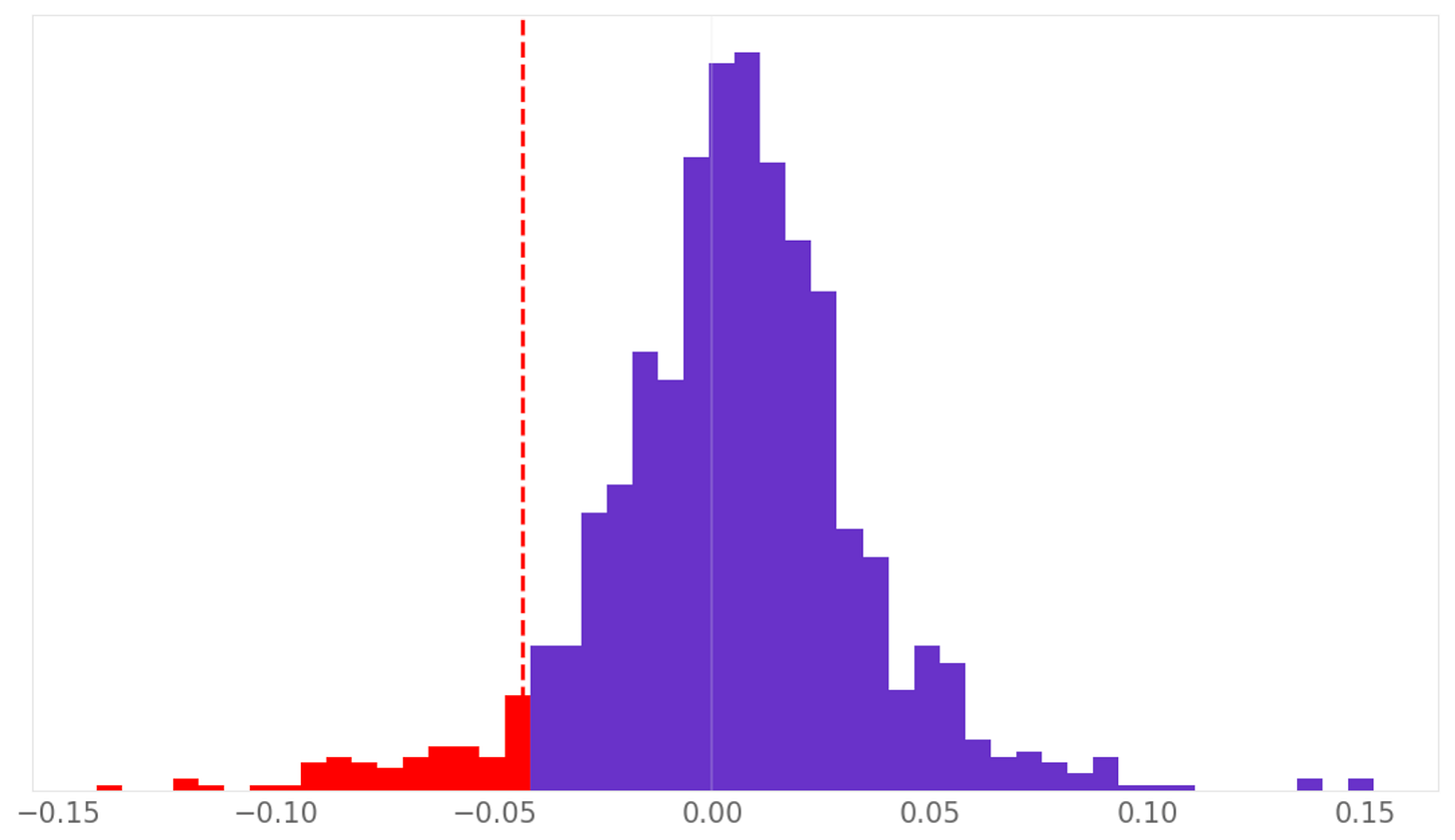
在 2022 年 1 月 21 日回顾过去 5 年,苹果的 3 天收益分布
The probability of a drop of that magnitude or higher, given it was a drop, is the area of the red region in the histogram divided by the area of the histogram at the left of zero. This value is 10.7% and represents how rare an event like this is.
该 大于或等于该幅度跌幅的概率,在出现下跌的情况下,是直方图中红色区域的面积除以零左侧直方图的面积。这个值是 10.7% 并且 代表了像这样的事件有多么稀少。
Not surprisingly, if we had bought Apple stock at the next open and held it for 5 days, we would have profited +6.4%.
毫不奇怪,如果我们在下一个开盘时购买了苹果股票并持有 5 天,我们将获得+6.4%的利润。
Now, let's look at an example in the short side. On Nov 2nd, 2023, Tesla stock had accumulated a 3-day price surge of +10.7%. This is also rare. On that day, if we looked back 5 years, this would be the 3-day returns distribution for Tesla stock:
现在,让我们看一个做空的例子。在 2023 年 11 月 2 日,特斯拉股票累计了 3 天的价格飙升,幅度为+10.7%。这也很少见。在那一天,如果我们回顾过去 5 年,这将是特斯拉股票的 3 天收益分布:
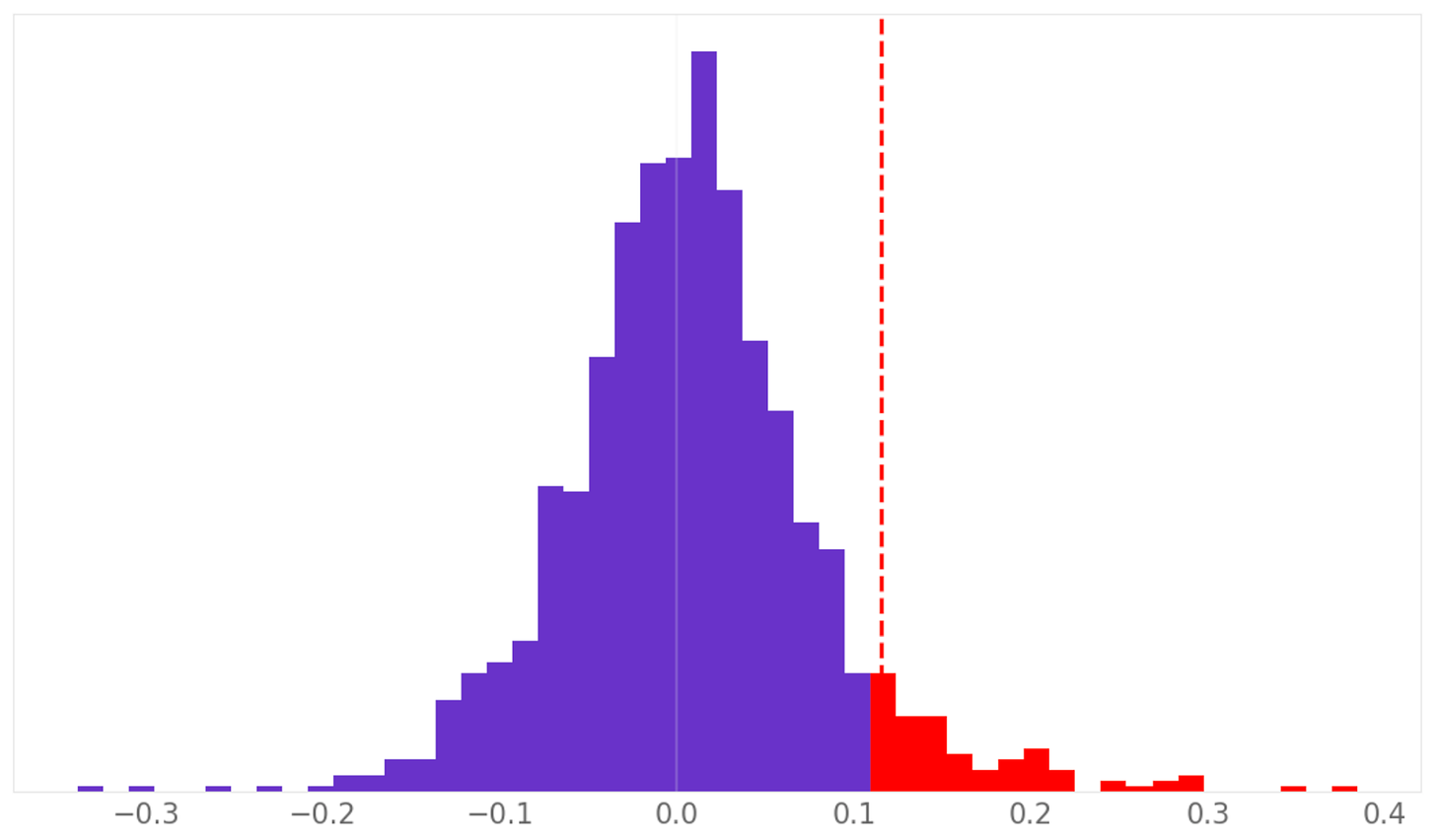
特斯拉的三天回报分布,回顾至 2023 年 11 月 2 日的五年数据
The probability of a surge of that magnitude or higher, given it was a price increase, is the area of the red region in the histogram divided by the area of the histogram at the right of zero. This value is 11.7%, and it represents how rare an event like this is.
在价格上涨的情况下,出现如此规模或更大规模的激增的概率是直方图中红色区域的面积与零右侧的直方图区域的面积之比。这个值是11.7%,它代表了像这样的事件有多罕见。
Also not surprisingly, if we had sold Tesla stock at the next opening and covered the position 5 days later, we would have profited +5.1%.
同样不出意外,如果我们在下一个开盘时卖出特斯拉股票,并在 5 天后平仓,我们将获利 +5.1%。
The QP indicator and its edge
QP 指标及其优势
For the lack of a better name, let's call this indicator QP (Quantitativo's Probability). It indicates how probable a price change of that magnitude or more extreme is, in a window of N days, looking back at Y years from the date in question.
由于没有更好的名称,我们将这个指标称为QP(Quantitativo 的概率)。它指示在 N 天的时间窗口内,该幅度或更极端的价格变化的可能性有多大,以问题日期为起点回溯 Y 年。
Let's set a default window N of 3 days, as we want to capture short-term mean reversion, and a default look back of 5 years to retrieve the 3-day returns histogram. So, to compute the QP indicator for the stock S on a given date:
我们设定一个默认值 窗口 N 为 3 天,因为我们想捕捉短期均值回归,以及一个默认的 回溯期为 5 年来获取 3 天收益直方图。因此,要计算股票 S 在给定日期的 QP 指标:
We look back 5 years from the given date and get all 3-day returns in this period;
我们从给定日期回溯 5 年,获取这一期间内所有 3 天的收益;Then, we get the percentile of the 3-day price change on the given date if the 3-day price change is negative (or 100 minus the percentile if the 3-day price change is positive);
然后,我们在给定日期获取 3 天价格变化的百分位数,如果 3 天价格变化为负(如果 3 天价格变化为正,则为 100 减去百分位数);Finally, we divide this value by the area at the left of the zero in the histogram if the 3-day price change is negative (or at the right if it is positive) and multiply by 100.
最后,如果 3 天价格变化为负,我们将此值除以直方图中零左边的区域(如果为正,则除以右边的区域),并乘以 100。
That's it. This number will vary between 0 and 100. The closer it is to zero, the rarer the event. The closer it is to 100, the more common the price change.
就这样。这个数字将在 0 和 100 之间变动。越接近零,事件越稀有。越接近 100,价格变化越常见。
Now, let's check QP's edge. What would have happened if we had bought all events when a stock had had a price drop, when its 3-day QP indicator was below 15, with its closing price above its 200-day simple moving average (stock in an upward trend), and held it for 5 days?
现在,让我们检查 QP 的边际。假设我们在一只股票出现 价格下跌时购买了所有事件,当其 3 天 QP 指标 低于 15,且其 收盘价高于其 200 天简单移动平均线 (股票处于上升趋势),并且 持有 5 天会怎样?
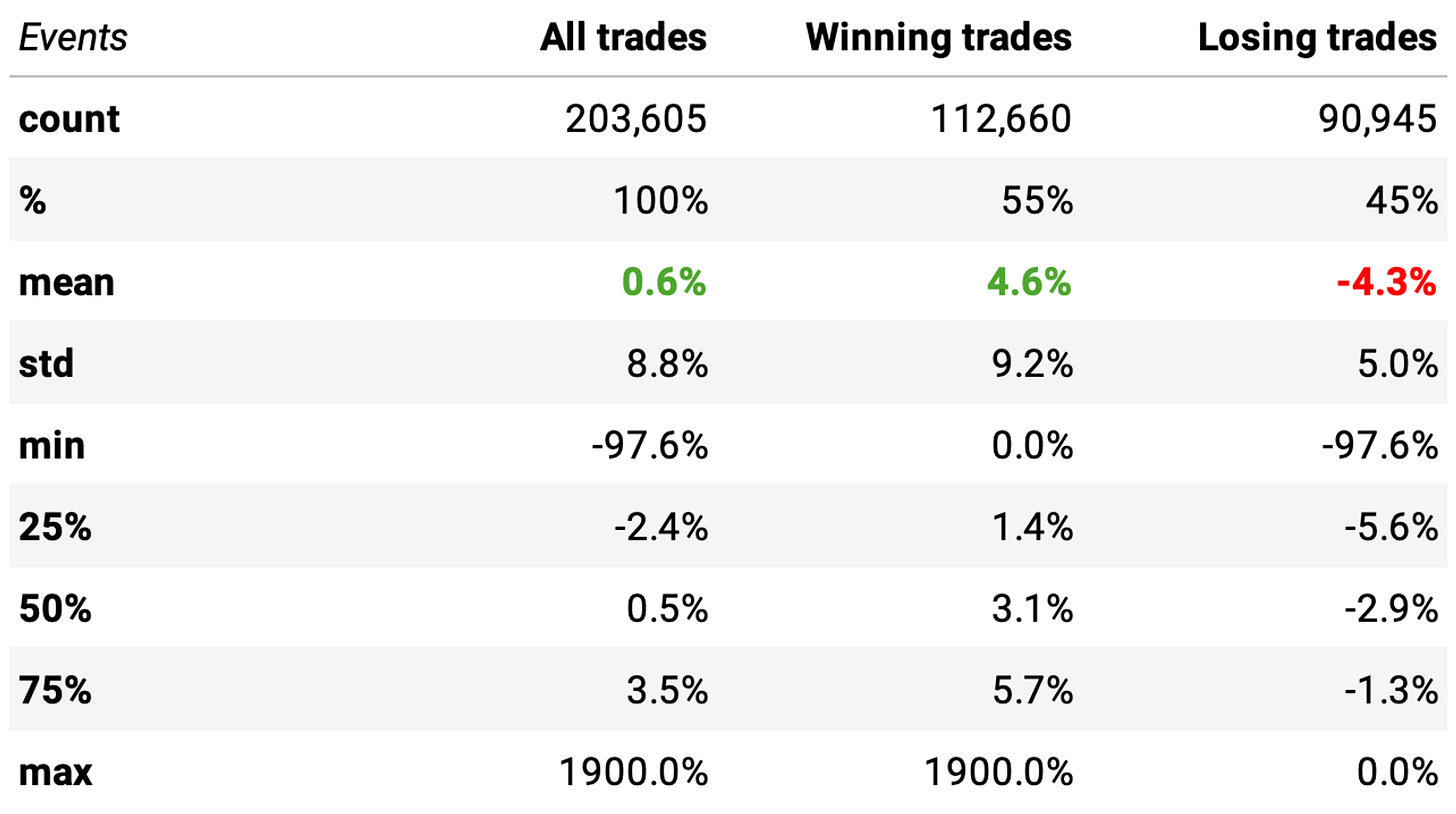
当股票价格下跌、其 QP 降至 15 以下并持有 5 天时的所有事件购买统计数据
We see a positive expected return:
我们看到一个积极的预期回报:
We can expect a +0.6% return/trade
我们可以预期每笔交易 +0.6% 的回报The win ratio is at 55%
胜率为 55%There's a positive payoff ratio: the expected return in winning trades is +4.6%, vs -4.3% on losing trades.
正收益比率:获胜交易的预期回报为+4.6%,而亏损交易为-4.3%。
Now, let's look at non-events. We define non-events as buying stocks whose 3-day QP is above 15, with all other conditions the same as the events.
现在,让我们来看一下非事件。我们将非事件定义为购买 3 天 QP 高于 15 的股票,而其他所有条件与事件相同。
Here, we see worse stats: the expected return per trade is +0.3%, half of what we saw on the events, while the win rate is slightly worse as well.
在这里,我们看到更糟的统计数据:每笔交易的预期回报为 +0.3%,是我们在事件中看到的一半,而胜率也稍微差一些。
But most importantly, the P-value is well below 0.05: the means of the two distributions are significantly different. So, we have an edge.
但最重要的是, P 值远低于 0.05:两个分布的均值 显著不同。所以,我们有优势。
Now, let's check how many stocks trigger the entry rule (i.e., have their QP indicator below 15) throughout history:
现在,让我们检查有多少股票在历史上触发了入场规则(即,QP 指标低于 15):
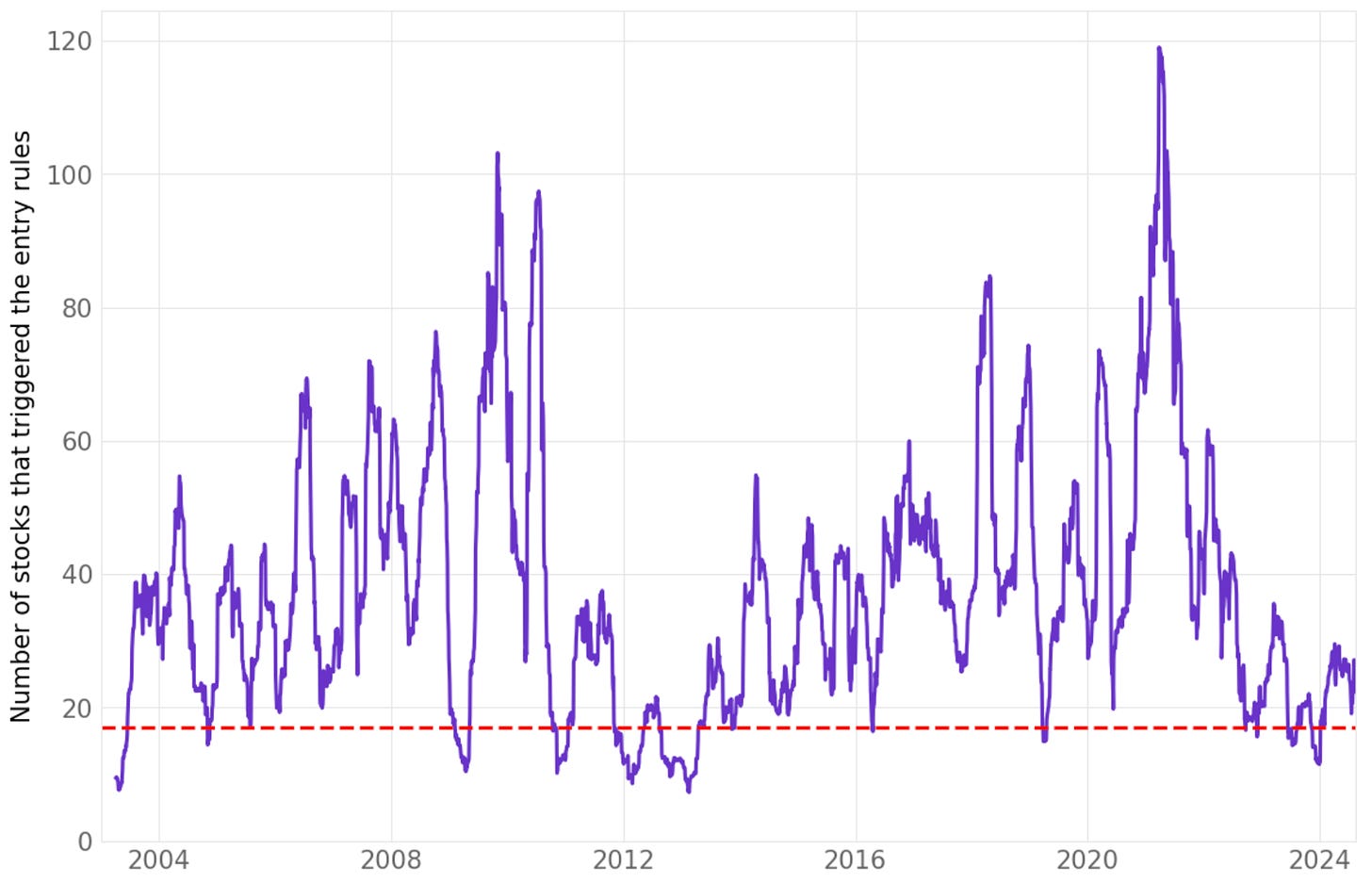
事件频率(63 天移动平均平滑曲线)。中位数为 17。
So, we reconstructed our understanding of a mean reversion strategy, rethinking the entry signal in terms of probabilities rather than the commonly used RSI. This led to the creation of a new indicator, which we called the QP indicator. Now, let's see how useful this indicator is.
因此,我们对均值回归策略的理解进行了重构,从概率的角度重新思考入场信号,而不是常用的相对强弱指数(RSI)。这导致了一个新指标的创建,我们称之为 QP 指标。现在,让我们看看这个指标有多有用。
The strategy 策略
The strategy we will trade is actually pretty simple. At the opening of every trading session:
我们将交易的策略其实非常简单。在每个交易时段开始时:
We will split our capital into 10 slots and buy stocks whose 3-day QP indicator from the previous day closed below 15;
我们将把资本分成 10 个部分 并购买前一天 3 天 QP 指标低于 15 的股票;We will only trade stocks whose price closed above its 200-day simple moving average;
我们只交易收盘价高于其 200 日简单移动平均线的股票;If there are more than 10 stocks in the universe with the entry signal triggered, we will sort them by turnover and prioritize the most liquid ones;
如果在 市场中有超过 10 只股票 触发了入场信号,我们 将按成交量对它们进行排序,并优先考虑流动性最高的股票;We will hold 10 positions maximum at any given moment.
我们将 最多持有 10 个头寸 在任何给定时刻。
When the stock closes above yesterday's high, we will exit on the next open.
当 股票收盘高于昨天的最高点时,我们将 在下一个开盘时退出.
We will only trade S&P 500 constituents. The test does not have either survivorship bias or selection bias. We use Norgate data (S&P 500 Current & Past).
我们将 仅交易标普 500 成分股。该测试没有生存偏差或选择偏差。我们使用 Norgate 数据(标普 500 当前和过去)。
That's it. Let's see how this strategy performs.
就这样。让我们看看这个策略的表现如何。
Experiments 实验
We start by backtesting the strategy exactly as defined above:
我们首先按照上述定义的方式对策略进行回测:
Wow! That was surprisingly positive for a first experiment:
哇!这对于第一次实验来说出乎意料地积极:
The annual return achieved is 15.5% vs. 8.5% the S&P 500 in the same period;
回报率年化收益 达到 15.5% 比 8.5% 在同一时期的标准普尔 500 指数;The strategy was positive in 21 out of 22 years;
该策略在 22 年中有 21 年为正;The maximum drawdown is at 30%, about half of the benchmark;
最大回撤为 30%,约为基准的一半;The risk-adjusted return is at 0.93 (Sharpe), vs 0.53 the benchmark;
风险调整后的收益为 0.93(夏普),相比基准的 0.53;The strategy trades 399 times/year, or about 7-8 times/week, with a win rate of 65.5%;
该策略每年交易399 次,或每周约 7-8 次,胜率为65.5%;The expected return per trade is +0.39%, with a payoff ratio of 0.71.
每笔交易的预期收益为 +0.39%,其 回报比为0.71。
How to improve it? 怎么改善它?
Reducing the number of slots
减少插槽数量
Instead of trading 10 instruments in parallel, let's reduce the number of slots to 4.
与其平行交易 10 个工具,不如将槽位数量减少到 4。
The highlights: 亮点:
The annual return increased to 19.9%, over twice the benchmark;
年度回报增加到 19.9%,超过基准的两倍;The Sharpe ratio also increased, now reaching 0.98;
夏普比率也有所提高,现在达到 0.98;The maximum drawdown remained about the same, at 31%, vs. 57% the S&P 500;
最大回撤保持在大约 31%,而标准普尔 500 指数为 57%;The number of trades/year dropped by more than half, now at 187 (3-4/week).
每年的交易次数减少了一半以上,目前为 187 次(每周 3-4 次)。
What else could we do to improve it?
我们还可以做些什么来改善它?
Long & short 长期和短期
Now, let's add a short leg to the strategy. It will work exactly as the long, but in reverse. The QP indicator threshold for going long or short will be 15. The difference between long and short is that:
现在,让我们 添加一个短期策略。它将与长期策略完全相同,但相反。进行长期或短期交易的 QP 指标阈值为15。长期和短期的区别在于:
Long opportunities are stocks whose price dropped in the past 3 days, whose QP is below 15 (i.e., the probability of a price drop of that magnitude or higher is 15%), and whose price has closed above its 200-day simple moving average;
长期机会是过去 3 天内价格 下跌的股票,其 QP 为低于 15(即,达到该幅度或更高幅度的价格下跌概率为 15%),并且其价格已收于200 日简单移动平均线之上;Short opportunities are stocks whose price surged in the past 3 days, whose QP is also below 15 (i.e., the probability of a price increase of that magnitude or higher is 15%), and whose price has closed below its 200-day simple moving average;
短期机会是过去 3 天内价格 上涨的股票,其 QP 也为低于 15(即,达到该幅度或更高幅度的价格上涨概率为 15%),并且其价格已收于200 日简单移动平均线之下;
Additionally: 此外:
For longs, we will continue with 4 slots as the maximum number of positions we will hold in the long portfolio at any given moment;
对于 多头,我们将 继续保持 4 个头寸,作为 我们在任何时候将在多头投资组合中持有的最大头寸数量;For shorts, we will set 12 slots as the maximum number of positions we will hold in the short portfolio at any given moment. That's because we want more diversification in the short side to protect ourselves from outliers;
对于 空头,我们将 设置 12 个头寸作为最大头寸数量,我们将在任何时候在空头投资组合中持有。这是因为我们想要 更多的多样化,以 保护自己 免受异常值的影响;We are sorting opportunities by turnover and restricting ourselves to trade S&P 500 constituents. This means we will be going long and short for the largest stocks in the market. To increase our protection on the short side even further, we will make the short portfolio 15% the size of the long portfolio.
我们正在按营业额对机会进行排序,并限制自己只交易标普 500 成分股。这意味着我们将对 市场上最大的股票进行多头和空头交易。为了进一步提高我们在空头方面的保护,我们将 将空头投资组合的规模设定为多头投资组合的 15%。
Now, let's see how these rules perform:
现在,让我们看看这些规则的表现:
Another significant improvement:
另一个重要的改进:
We achieved a 23.9% annual return, about 3x the benchmark;
我们实现了一个23.9%的年回报,约为基准的三倍;Sharpe ratio increased to 1.10, over twice the S&P 500;
夏普比率增加至1.10,超过标准普尔 500 指数的两倍;Maximum drawdown reduced from 30% in the previous experiments to 26%, less than half the benchmark;
最大回撤从之前实验的 30%减少至26%,不到基准的一半;The average return/trade increased to +0.65%;
平均每笔交易的回报增加至+0.65%;The win rate slightly decreased to 64%, while the payoff ratio improved to 0.81;
赢率略微下降至 64%,而收益比率改善至0.81;However, adding a short portfolio with 12 slots significantly increased the # of trades/year, now at 888 in total (about 17 trades/week, or 3-4 trades/day).
然而,增加一个有 12 个插槽的短期投资组合显著提高了每年交易的数量,现在总交易次数达到 888 次(约每周 17 笔交易,或每天 3-4 笔交易)。
Let's see the returns month by month:
让我们按月查看收益:
If we had traded this strategy since 2023:
如果我们从 2023 年开始交易这个策略:
We would have had only 1 down year in 22 years (2010);
我们将 只有 22 年中仅有 1 年下跌 (2010);We would have seen 64% of the months positive, with the best at +21.4% (Sep’11);
我们将看到 64%的月份是积极的, 最好在+21.4% (2011 年 9 月);We would have seen 36% of the months negative, with the worst at -11.2% (Jun'10);
我们将看到 36%的月份是消极的, 最差在-11.2% (2010 年 6 月);The longest positive streak would have been 9 months, from Feb'03 to Oct'03;
这 最长的 积极连续期 将是 9 个月, 从 2003 年 2 月到 2003 年 10 月;The longest negative streak would have been 6 months, from Apr'21 to Sep'21.
最长的负面持续时间为6 个月,从 2021 年 4 月到 2021 年 9 月。
Trading costs 交易成本
Finally, let's check the impact of trading costs on the results:
最后,让我们检查交易成本对结果的影响:
As expected, the higher the trading costs, the lower the returns.
正如预期的那样,交易成本越高,收益就越低。
Final thoughts 结论
First Principles Thinking is a powerful problem-solving approach. When applied to rethinking a mean reversion strategy, it enabled the creation of a completely new indicator from fundamental elements, resulting in an effective and profitable strategy.
基本原则思维是一种强大的问题解决方法。当应用于重新思考平均回归策略时,它促成了从基本元素中创建一个全新的指标,从而形成了一种有效且盈利的策略。
Should we trade this strategy as is? Hold your horses. There are improvements to be made in this strategy such that we get a better win ratio and a better payoff ratio. In fact, this strategy is the first step of a larger vision, to be shared with time. Baby steps. :)
我们应该按照现有策略进行交易吗?等一下。在这个策略中还有改进的空间,以便我们获得更好的胜率和更好的收益率。事实上,这个策略是一个更大愿景的第一步,将会随着时间的推移分享。一步一个脚印。 :)
I'd love to hear your thoughts about this approach. If you have any questions or comments, just reach out via Twitter or email.
我很想听听你对这种方法的看法。如果你有任何问题或意见, 可以通过 Twitter 或 电子邮件与我联系。
Also, if you want to implement this strategy (or any other strategy) and need help, just let me know.
另外, 如果你想实施这个策略(或任何其他策略)并需要帮助,请告诉我。
Cheers! 干杯!
Instead of doing short, can we apply market regime filter (for instance based on ATR or similar)?
我们能否应用市场制度过滤,而不是做短期操作(比如基于 ATR 或类似的指标)?
Curious if you are doing more RealTest - this QP indicator I can't figure out how to code in RealTest yet. Would be interested to know if it's possible or if you need to use more sequential/looping like in Amibroker or Python (or a dll)
好奇你是否在做更多的 RealTest - 这个 QP 指标我还不知道如何在 RealTest 中编码。想知道这是否可能,还是需要像在 Amibroker 或 Python(或 dll)中那样使用更多的顺序/循环。
2 条由 Quantitativo 和其他人的回复