What We Learned from a Year of Building with LLMs (Part III): Strategy
从一年的LLMs建设中我们学到的(第三部分):策略
To hear directly from the authors on this topic, sign up for the upcoming virtual event on June 20th, and learn more from the Generative AI Success Stories Superstream on June 12th.
要直接听取作者对这一主题的看法,请报名参加 6 月 20 日即将举行的虚拟活动,并在 6 月 12 日的生成式 AI 成功故事超级直播中了解更多。
Part I of this series can be found here and part II can be found here.
本系列的第一部分可以在这里找到,第二部分可以在这里找到。
We previously shared our insights on the tactics we have honed while operating LLM applications. Tactics are granular: they are the specific actions employed to achieve specific objectives. We also shared our perspective on operations: the higher-level processes in place to support tactical work to achieve objectives.
我们之前分享了在运营LLM个应用过程中磨练出的策略见解。策略是细致的:它们是为实现特定目标而采取的具体行动。我们还分享了对运营的看法:即为支持战术工作以实现目标而设立的更高级别的流程。
But where do those objectives come from? That is the domain of strategy. Strategy answers the “what” and “why” questions behind the “how” of tactics and operations.
但是,这些目标从何而来?这是战略的范畴。战略回答了战术和行动背后的“什么”和“为什么”的问题。
We provide our opinionated takes, such as “no GPUs before PMF” and “focus on the system not the model,” to help teams figure out where to allocate scarce resources. We also suggest a roadmap for iterating toward a great product. This final set of lessons answers the following questions:
我们提出有观点的见解,比如“在达到产品与市场匹配前不使用 GPU”和“关注系统而非模型”,以帮助团队确定稀缺资源的分配方向。我们还为迭代出优秀产品提出路线图。这最后一组课程回答了以下问题:
- Building vs. Buying: When should you train your own models, and when should you leverage existing APIs? The answer is, as always, “it depends.” We share what it depends on.
构建与购买:何时应该训练自己的模型,何时应该利用现有 API?答案是,一如既往地,“这取决于”。我们分享它取决于什么。 - Iterating to Something Great: How can you create a lasting competitive edge that goes beyond just using the latest models? We discuss the importance of building a robust system around the model and focusing on delivering memorable, sticky experiences.
迭代至卓越:如何创造超越仅仅使用最新模型的持久竞争优势?我们讨论围绕模型构建稳健系统以及专注于提供难忘、粘性的体验的重要性。 - Human-Centered AI: How can you effectively integrate LLMs into human workflows to maximize productivity and happiness? We emphasize the importance of building AI tools that support and enhance human capabilities rather than attempting to replace them entirely.
以人为本的 AI:如何将LLMs有效融入人类工作流程中,以最大化生产力和幸福感?我们强调构建支持和增强人类能力的 AI 工具的重要性,而不是试图完全取代它们。 - Getting Started: What are the essential steps for teams embarking on building an LLM product? We outline a basic playbook that starts with prompt engineering, evaluations, and data collection.
开始:团队开始构建LLM产品时,哪些是必不可少的步骤?我们概述了一个基本的指南,从提示工程、评估和数据收集开始。 - The Future of Low-Cost Cognition: How will the rapidly decreasing costs and increasing capabilities of LLMs shape the future of AI applications? We examine historical trends and walk through a simple method to estimate when certain applications might become economically feasible.
低成本认知的未来:快速降低的成本和LLMs的日益增强的能力将如何塑造人工智能应用的未来?我们研究历史趋势,并通过一种简单的方法来估算某些应用可能变得经济可行的时间。 - From Demos to Products: What does it take to go from a compelling demo to a reliable, scalable product? We emphasize the need for rigorous engineering, testing, and refinement to bridge the gap between prototype and production.
从演示到产品:从引人入胜的演示转变为可靠、可扩展的产品需要什么?我们强调需要严谨的工程设计、测试和改进,以弥合原型和生产之间的差距。
To answer these difficult questions, let’s think step by step…
为了解答这些难题,让我们一步步来思考..
Strategy: Building with LLMs without Getting Out-Maneuvered
策略:在不被LLMs超越的情况下建设
Successful products require thoughtful planning and tough prioritization, not endless prototyping or following the latest model releases or trends. In this final section, we look around the corners and think about the strategic considerations for building great AI products. We also examine key trade-offs teams will face, like when to build and when to buy, and suggest a “playbook” for early LLM application development strategy.
成功的产品需要深思熟虑的规划和艰难的优先级排序,而不是无休止的原型设计或追随最新的模型发布或趋势。在这一最后部分,我们将着眼于未来,思考构建优秀 AI 产品时的战略考量。我们还会探讨团队将面临的权衡,比如何时构建和何时购买,并为早期LLM应用开发策略提出一个“指南”。
No GPUs before PMF
在 PMF 之前没有 GPU
To be great, your product needs to be more than just a thin wrapper around somebody else’s API. But mistakes in the opposite direction can be even more costly. The past year has also seen a mint of venture capital, including an eye-watering six-billion-dollar Series A, spent on training and customizing models without a clear product vision or target market. In this section, we’ll explain why jumping immediately to training your own models is a mistake and consider the role of self-hosting.
要成为伟大的产品,仅仅作为别人 API 的薄包装是不够的。但相反方向的错误可能会更加昂贵。过去一年,风险资本大量涌现,包括令人瞠目结舌的 60 亿美元 A 轮融资,被用于训练和定制模型,而没有明确的产品愿景或目标市场。在这一部分,我们将解释为什么立即转向训练自己的模型是一个错误,并考虑自托管的角色。
为了成为卓越的产品,你的产品需要不仅仅是别人 API 的简单外壳。但向相反方向的失误可能代价更高。过去一年,我们见证了大量风险资本的涌入,包括令人咋舌的 60 亿美元 A 轮融资,被用于训练和定制模型,却没有明确的产品愿景或目标市场。在这一部分,我们将阐述为何立即着手训练自己的模型是个错误,并探讨自托管的作用。
(注意:由于原文有两段内容,我将其合并为一段进行翻译,以保持连贯性。)
Training from scratch (almost) never makes sense
从零开始训练(几乎)从来没有意义
For most organizations, pretraining an LLM from scratch is an impractical distraction from building products.
对大多数组织而言,从零开始预训练一个LLM是不切实际的,会分散构建产品时的注意力。
As exciting as it is and as much as it seems like everyone else is doing it, developing and maintaining machine learning infrastructure takes a lot of resources. This includes gathering data, training and evaluating models, and deploying them. If you’re still validating product-market fit, these efforts will divert resources from developing your core product. Even if you had the compute, data, and technical chops, the pretrained LLM may become obsolete in months.
尽管这看起来令人兴奋,似乎每个人都在这么做,但开发和维护机器学习基础设施需要大量资源。这包括收集数据、训练和评估模型以及部署它们。如果你还在验证产品与市场匹配度,这些努力会分散开发核心产品所需的资源。即使你拥有计算能力、数据和技术实力,预训练的LLM可能在几个月内就会过时。
Consider the case of BloombergGPT, an LLM specifically trained for financial tasks. The model was pretrained on 363B tokens and required a heroic effort by nine full-time employees, four from AI Engineering and five from ML Product and Research. Despite this effort, it was outclassed by gpt-3.5-turbo and gpt-4 on those financial tasks within a year.
考虑一下 BloombergGPT 的情况,这是一个LLM专门针对金融任务进行训练的模型。该模型在 363B 个令牌上进行了预训练,需要九名全职员工的英雄般努力,其中四人来自人工智能工程部门,五人来自机器学习产品和研究部门。尽管付出了这些努力,但在一年内,它在这些金融任务上被 gpt-3.5-turbo 和 gpt-4 超越了。
考虑到 BloombergGPT 的情况,这是一个LLM专门针对金融任务训练的模型。该模型在 363B 个标记上进行了预训练,需要九名全职员工的共同努力,其中四人来自 AI 工程部门,五人来自 ML 产品和研究部门。尽管付出了这些努力,但在一年内,它在金融任务上的表现被 gpt-3.5-turbo 和 gpt-4 超越了。
注:由于原文中的LLM不是一个明确的词汇,因此在翻译中保留了原文的标记。在实际翻译中,应根据具体上下文或客户要求处理此类标记。
This story and others like it suggests that for most practical applications, pretraining an LLM from scratch, even on domain-specific data, is not the best use of resources. Instead, teams are better off fine-tuning the strongest open source models available for their specific needs.
这个故事和其他类似的故事表明,对于大多数实际应用,从零开始预训练一个LLM,即使是在特定领域的数据上,也不是资源的最佳利用方式。相反,团队最好根据自己的具体需求,对可用的最强开源模型进行微调。
There are of course exceptions. One shining example is Replit’s code model, trained specifically for code-generation and understanding. With pretraining, Replit was able to outperform other models of large sizes such as CodeLlama7b. But as other, increasingly capable models have been released, maintaining utility has required continued investment.
当然也有例外。一个杰出的例子是 Replit 的代码模型,该模型专门用于代码生成和理解的训练。通过预训练,Replit 能够胜过其他大型模型,如 CodeLlama7b。但随着其他能力越来越强的模型的发布,保持实用性需要持续的投资。
Don’t fine-tune until you’ve proven it’s necessary
在证明有必要之前,不要进行微调
For most organizations, fine-tuning is driven more by FOMO than by clear strategic thinking.
对大多数组织而言,微调更多的是受到错失恐惧症(FOMO)的驱动,而不是清晰的战略思维。
Organizations invest in fine-tuning too early, trying to beat the “just another wrapper” allegations. In reality, fine-tuning is heavy machinery, to be deployed only after you’ve collected plenty of examples that convince you other approaches won’t suffice.
组织过早地在微调上投入,试图驳斥“只不过是另一个包装”的指责。实际上,微调是重型机械,只有在你收集了大量例子,使你确信其他方法不足以解决问题后,才应该部署。
A year ago, many teams were telling us they were excited to fine-tune. Few have found product-market fit and most regret their decision. If you’re going to fine-tune, you’d better be really confident that you’re set up to do it again and again as base models improve—see the “The model isn’t the product” and “Build LLMOps” below.
一年前,许多团队告诉我们他们对微调感到兴奋。但很少有团队找到了产品与市场的契合点,大多数团队都对自己的决定感到后悔。如果你要进行微调,你最好非常确信你已经准备好在基础模型改进时一遍又一遍地进行微调——请参阅下面的“模型不是产品”和“构建 LLMOps”。
When might fine-tuning actually be the right call? If the use case requires data not available in the mostly open web-scale datasets used to train existing models—and if you’ve already built an MVP that demonstrates the existing models are insufficient. But be careful: if great training data isn’t readily available to the model builders, where are you getting it?
在什么情况下,微调实际上可能是正确的选择?如果使用案例需要的数据在用于训练现有模型的主要是开放的网络规模数据集中不可用——并且你已经构建了一个最小可行产品(MVP),证明现有模型不足。但要小心:如果优秀的训练数据对模型构建者来说并不容易获得,你从哪里得到它?
Ultimately, remember that LLM-powered applications aren’t a science fair project; investment in them should be commensurate with their contribution to your business’ strategic objectives and its competitive differentiation.
最终,请记住LLM驱动的应用程序不是科学展览项目;对它们的投资应与它们对您企业战略目标和竞争优势的贡献相称。
Start with inference APIs, but don’t be afraid of self-hosting
从推理 API 开始,但不要害怕自托管
With LLM APIs, it’s easier than ever for startups to adopt and integrate language modeling capabilities without training their own models from scratch. Providers like Anthropic and OpenAI offer general APIs that can sprinkle intelligence into your product with just a few lines of code. By using these services, you can reduce the effort spent and instead focus on creating value for your customers—this allows you to validate ideas and iterate toward product-market fit faster.
通过LLM个 API,初创企业比以往任何时候都更容易采用和集成语言建模能力,而无需从头开始训练自己的模型。像 Anthropic 和 OpenAI 这样的提供商提供了通用 API,只需几行代码就能为您的产品注入智能。通过使用这些服务,您可以减少投入的努力,转而专注于为您的客户创造价值——这使您能够更快地验证想法并迭代向产品市场适应性迈进。
But, as with databases, managed services aren’t the right fit for every use case, especially as scale and requirements increase. Indeed, self-hosting may be the only way to use models without sending confidential/private data out of your network, as required in regulated industries like healthcare and finance or by contractual obligations or confidentiality requirements.
但是,就像数据库一样,托管服务并不适合所有使用案例,尤其是随着规模和需求的增加。事实上,在医疗保健和金融等受监管行业,或因合同义务或保密要求,自我托管可能是唯一在不将机密/私有数据传出网络的情况下使用模型的方法。
Furthermore, self-hosting circumvents limitations imposed by inference providers, like rate limits, model deprecations, and usage restrictions. In addition, self-hosting gives you complete control over the model, making it easier to construct a differentiated, high-quality system around it. Finally, self-hosting, especially of fine-tunes, can reduce cost at large scale. For example, BuzzFeed shared how they fine-tuned open source LLMs to reduce costs by 80%.
此外,自托管还规避了推理提供商施加的限制,如速率限制、模型淘汰和使用限制。此外,自托管使您能够完全控制模型,从而更容易围绕模型构建具有差异化、高质量的系统。最后,自托管,尤其是微调模型,在大规模下可以降低成本。例如,BuzzFeed 分享了他们如何对开源 LLMs 进行微调,从而将成本降低了 80%。
Iterate to something great
迭代至卓越
To sustain a competitive edge in the long run, you need to think beyond models and consider what will set your product apart. While speed of execution matters, it shouldn’t be your only advantage.
要在长期内保持竞争优势,你需要超越模型思考,考虑什么将使你的产品与众不同。虽然执行速度很重要,但不应该是你唯一的竞争优势。
The model isn’t the product; the system around it is
模型本身不是产品;围绕它的系统才是产品
For teams that aren’t building models, the rapid pace of innovation is a boon as they migrate from one SOTA model to the next, chasing gains in context size, reasoning capability, and price-to-value to build better and better products.
对于那些不构建模型的团队来说,创新的快速步伐是一个福音,因为他们从一个最先进的模型迁移到下一个,追求上下文大小、推理能力和价格与价值的提升,以构建越来越好产品。
This progress is as exciting as it is predictable. Taken together, this means models are likely to be the least durable component in the system.
这种进步既令人兴奋又可预见。综合来看,这意味着模型可能是系统中最不耐用的组件。
Instead, focus your efforts on what’s going to provide lasting value, such as:
相反,将您的努力集中在能提供持久价值的事物上,例如:
- Evaluation chassis: To reliably measure performance on your task across models
评估底盘:为了在不同模型上可靠地测量您任务的性能 - Guardrails: To prevent undesired outputs no matter the model
护栏:无论模型如何,防止不希望的输出 - Caching: To reduce latency and cost by avoiding the model altogether
缓存:通过完全避免模型来减少延迟和成本 - Data flywheel: To power the iterative improvement of everything above
数据飞轮:为上述所有内容的迭代改进提供动力
These components create a thicker moat of product quality than raw model capabilities.
这些组件创造的产品质量更厚的护城河,比原始模型能力。
(For a more accurate translation: 这些组件创造的产品质量护城河比原始模型能力更厚。)
But that doesn’t mean building at the application layer is risk free. Don’t point your shears at the same yaks that OpenAI or other model providers will need to shave if they want to provide viable enterprise software.
但这并不意味着在应用层构建没有风险。不要将你的剪刀指向 OpenAI 或其他模型提供商需要剪羊毛的同一批牦牛,如果他们想要提供可行的企业软件的话。
For example, some teams invested in building custom tooling to validate structured output from proprietary models; minimal investment here is important, but a deep one is not a good use of time. OpenAI needs to ensure that when you ask for a function call, you get a valid function call—because all of their customers want this. Employ some “strategic procrastination” here, build what you absolutely need and await the obvious expansions to capabilities from providers.
例如,一些团队投资开发了自定义工具,用于验证专有模型的结构化输出;在这里进行最少的投资是重要的,但深入投资则不是时间的合理利用。OpenAI 需要确保当你请求函数调用时,你得到的是有效的函数调用——因为他们的所有客户都希望这样。在这里采用一些“战略性拖延”,构建你绝对需要的东西,并等待供应商能力的明显扩展。
Build trust by starting small
通过从小事做起建立信任
Building a product that tries to be everything to everyone is a recipe for mediocrity. To create compelling products, companies need to specialize in building memorable, sticky experiences that keep users coming back.
试图打造一款能满足所有人的产品,只会导致平庸。要创造出吸引人的产品,公司需要专注于构建令人难忘、让人上瘾的体验,这样才能让用户不断回流。
Consider a generic RAG system that aims to answer any question a user might ask. The lack of specialization means that the system can’t prioritize recent information, parse domain-specific formats, or understand the nuances of specific tasks. As a result, users are left with a shallow, unreliable experience that doesn’t meet their needs.
考虑一个通用的 RAG 系统,旨在回答用户可能提出的任何问题。缺乏专业化意味着系统无法优先处理最近的信息,无法解析特定领域的格式,也无法理解特定任务的细微差别。结果,用户只能得到一个肤浅、不可靠的体验,无法满足他们的需求。
To address this, focus on specific domains and use cases. Narrow the scope by going deep rather than wide. This will create domain-specific tools that resonate with users. Specialization also allows you to be upfront about your system’s capabilities and limitations. Being transparent about what your system can and cannot do demonstrates self-awareness, helps users understand where it can add the most value, and thus builds trust and confidence in the output.
为了解决这个问题,专注于特定的领域和使用案例。通过深入而不是广泛来缩小范围。这将创建与用户产生共鸣的领域特定工具。专业化还使您能够坦率地说明系统的功能和局限性。对于系统能做什么和不能做什么的透明度表现出自我意识,帮助用户理解它在何处能增加最大价值,从而建立对输出的信任和信心。
为了应对这一挑战,专注于特定的领域和应用场景。通过深耕而不是广泛涉猎来缩小范围。这将创造出与用户产生共鸣的领域专用工具。专业化也使你能够明确地说明你的系统的能力和局限性。对于你的系统能做什么和不能做什么保持透明,展现出自我认知,帮助用户理解它在何处能发挥最大价值,从而建立起对输出结果的信任和信心。
(注:机器翻译可能存在一定的误差,建议人工校对)
Build LLMOps, but build it for the right reason: faster iteration
构建 LLMOps,但要出于正确的理由:更快的迭代速度
DevOps is not fundamentally about reproducible workflows or shifting left or empowering two pizza teams—and it’s definitely not about writing YAML files.
DevOps 并非从根本上讲的是可重复的工作流程,或是向左移,或是授权两个披萨团队——当然,它肯定不是关于编写 YAML 文件的。
DevOps is about shortening the feedback cycles between work and its outcomes so that improvements accumulate instead of errors. Its roots go back, via the Lean Startup movement, to Lean manufacturing and the Toyota Production System, with its emphasis on Single Minute Exchange of Die and Kaizen.
DevOps 是关于缩短工作与其成果之间的反馈周期,以便改进不断积累而不是错误。它的根源可以追溯到精益创业运动,进而追溯到精益制造和丰田生产系统,这些系统强调单一分钟换模和持续改进(Kaizen)。
(注:由于原文中 "Single Minute Exchange of Die" 和 "Kaizen" 是专业术语,通常在行业内直接使用英文表达,因此在翻译中保留了原文。)
MLOps has adapted the form of DevOps to ML. We have reproducible experiments and we have all-in-one suites that empower model builders to ship. And Lordy, do we have YAML files.
MLOps 已经将 DevOps 的形式应用于 ML。我们有可重复的实验,我们有一体化套件,使模型构建者能够发布模型。而且,我们确实有大量的 YAML 文件。
But as an industry, MLOps didn’t adapt the function of DevOps. It didn’t shorten the feedback gap between models and their inferences and interactions in production.
但作为一个行业,MLOps 并没有采纳 DevOps 的功能。它并没有缩短模型与其在生产中的推理和交互之间的反馈差距。
Hearteningly, the field of LLMOps has shifted away from thinking about hobgoblins of little minds like prompt management and toward the hard problems that block iteration: production monitoring and continual improvement, linked by evaluation.
令人鼓舞的是,LLMOps 领域已经不再关注像提示管理这样的琐碎问题,而是转向了阻碍迭代的难题:生产监控和持续改进,这两者通过评估联系在一起。
Already, we have interactive arenas for neutral, crowd-sourced evaluation of chat and coding models—an outer loop of collective, iterative improvement. Tools like LangSmith, Log10, LangFuse, W&B Weave, HoneyHive, and more promise to not only collect and collate data about system outcomes in production but also to leverage them to improve those systems by integrating deeply with development. Embrace these tools or build your own.
我们已经有了互动竞技场,用于中立、群众外包评估聊天和编码模型——这是一个集体、迭代改进的外部循环。像 LangSmith、Log10、LangFuse、W&B Weave、HoneyHive 等工具,不仅承诺收集和整理生产中系统结果的数据,而且还通过与开发深度集成,利用这些数据来改进这些系统。拥抱这些工具,或者自己构建。
Don’t build LLM features you can buy
不要构建你可以购买的LLM个功能
Most successful businesses are not LLM businesses. Simultaneously, most businesses have opportunities to be improved by LLMs.
大多数成功的企业都不是LLM企业。同时,大多数企业都有通过LLMs改进的机会。
This pair of observations often misleads leaders into hastily retrofitting systems with LLMs at increased cost and decreased quality and releasing them as ersatz, vanity “AI” features, complete with the now-dreaded sparkle icon. There’s a better way: focus on LLM applications that truly align with your product goals and enhance your core operations.
这对观察结果常常误导领导者匆忙地以更高的成本和更低的质量,将系统改造为LLMs,并将其作为假冒的、虚荣的“人工智能”功能发布,甚至还配上了现在令人讨厌的闪亮图标。有更好的方法:专注于LLM应用,这些应用真正与你的产品目标相吻合,并增强你的核心业务。
Consider a few misguided ventures that waste your team’s time:
考虑一些误导性的项目,这些项目浪费了你团队的时间:
- Building custom text-to-SQL capabilities for your business
为您的企业构建自定义的文本转 SQL 功能 - Building a chatbot to talk to your documentation
构建一个聊天机器人来与你的文档进行对话 - Integrating your company’s knowledge base with your customer support chatbot
将您公司的知识库与客户支持聊天机器人整合
While the above are the hellos-world of LLM applications, none of them make sense for virtually any product company to build themselves. These are general problems for many businesses with a large gap between promising demo and dependable component—the customary domain of software companies. Investing valuable R&D resources on general problems being tackled en masse by the current Y Combinator batch is a waste.
虽然上述应用是LLM的入门级示例,但几乎没有任何产品公司有必要自行构建这些应用。这些都是许多企业面临的一般性问题,这些问题在有前景的演示和可靠组件之间存在巨大差距,这正是软件公司的传统领域。将宝贵的研发资源投入到当前 Y Combinator 批次正在大规模解决的一般性问题上,是一种浪费。
If this sounds like trite business advice, it’s because in the frothy excitement of the current hype wave, it’s easy to mistake anything “LLM” as cutting-edge accretive differentiation, missing which applications are already old hat.
如果这听起来像是陈词滥调的商业建议,那是因为在当前炒作浪潮的浮躁兴奋中,很容易将任何“LLM”误认为是尖端的增值差异化,而忽略了哪些应用早已过时。
AI in the loop; humans at the center
人工智能在循环中;人类在中心
Right now, LLM-powered applications are brittle. They required an incredible amount of safe-guarding and defensive engineering and remain hard to predict. Additionally, when tightly scoped, these applications can be wildly useful. This means that LLMs make excellent tools to accelerate user workflows.
目前,由LLM驱动的应用程序很脆弱。它们需要大量的安全保护和防御性工程,并且仍然难以预测。此外,当严格限定范围时,这些应用程序可以变得非常有用。这意味着LLMs是加速用户工作流程的出色工具。
While it may be tempting to imagine LLM-based applications fully replacing a workflow or standing in for a job function, today the most effective paradigm is a human-computer centaur (c.f. Centaur chess). When capable humans are paired with LLM capabilities tuned for their rapid utilization, productivity and happiness doing tasks can be massively increased. One of the flagship applications of LLMs, GitHub Copilot, demonstrated the power of these workflows:
虽然想象LLM为基础的应用程序完全取代工作流程或代替工作职能可能很诱人,但目前最有效的范式是人机协作(参考半人马象棋)。当有能力的人与为快速利用而调整的LLM能力相结合时,执行任务的生产力和幸福感可以大幅提高。LLMs的旗舰应用之一,GitHub Copilot,展示了这些工作流程的力量:
“Overall, developers told us they felt more confident because coding is easier, more error-free, more readable, more reusable, more concise, more maintainable, and more resilient with GitHub Copilot and GitHub Copilot Chat than when they’re coding without it.”
“总体而言,开发者告诉我们,他们感到更加自信,因为使用 GitHub Copilot 和 GitHub Copilot Chat 编码比没有使用时更容易,错误更少,更易读,更易重用,更简洁,更易维护,更具弹性。”
—Mario Rodriguez, GitHub
— 马里奥·罗德里格斯,GitHub
For those who have worked in ML for a long time, you may jump to the idea of “human-in-the-loop,” but not so fast: HITL machine learning is a paradigm built on human experts ensuring that ML models behave as predicted. While related, here we are proposing something more subtle. LLM driven systems should not be the primary drivers of most workflows today; they should merely be a resource.
对于那些在机器学习领域工作了很长时间的人来说,你可能会立刻想到“人机协作”,但别急:人机协作的机器学习是一种建立在人类专家确保机器学习模型按预期行为的范式。虽然相关,但我们在这里提出的是更微妙的东西。LLM驱动的系统不应该是当今大多数工作流程的主要驱动因素;它们只应该是一种资源。
By centering humans and asking how an LLM can support their workflow, this leads to significantly different product and design decisions. Ultimately, it will drive you to build different products than competitors who try to rapidly offshore all responsibility to LLMs—better, more useful, and less risky products.
通过以人类为中心,探讨LLM如何支持其工作流程,这将导致截然不同的产品和设计决策。最终,这将促使你开发出与竞争对手不同的产品——更好、更有用、风险更低的产品,而这些竞争对手试图迅速将所有责任转移给LLMs。
Start with prompting, evals, and data collection
从提示、评估和数据收集开始
The previous sections have delivered a fire hose of techniques and advice. It’s a lot to take in. Let’s consider the minimum useful set of advice: if a team wants to build an LLM product, where should they begin?
前几节提供了大量的技巧和建议,内容很多。让我们考虑一下最少但最有用的建议集:如果一个团队想要构建一个LLM产品,他们应该从哪里开始?
Over the last year, we’ve seen enough examples to start becoming confident that successful LLM applications follow a consistent trajectory. We walk through this basic “getting started” playbook in this section. The core idea is to start simple and only add complexity as needed. A decent rule of thumb is that each level of sophistication typically requires at least an order of magnitude more effort than the one before it. With this in mind…
在过去的一年里,我们已经看到了足够的例子,开始有信心认为成功的LLM应用遵循一致的轨迹。在本节中,我们介绍了这个基本的“入门”指南。核心思想是从简单开始,只在需要时增加复杂性。一个不错的经验法则是,每一级的复杂性通常需要比前一级多至少一个数量级的努力。考虑到这一点……
Prompt engineering comes first
提示工程学优先
Start with prompt engineering. Use all the techniques we discussed in the tactics section before. Chain-of-thought, n-shot examples, and structured input and output are almost always a good idea. Prototype with the most highly capable models before trying to squeeze performance out of weaker models.
从提示工程开始。使用我们在策略部分讨论的所有技术。思维链、n-示例、结构化输入和输出几乎总是一个好主意。在尝试从较弱的模型中挤出性能之前,先使用最强大的模型进行原型设计。
Only if prompt engineering cannot achieve the desired level of performance should you consider fine-tuning. This will come up more often if there are nonfunctional requirements (e.g., data privacy, complete control, and cost) that block the use of proprietary models and thus require you to self-host. Just make sure those same privacy requirements don’t block you from using user data for fine-tuning!
只有在即时工程无法达到预期性能水平时,才应考虑微调。如果存在阻止使用专有模型的非功能性要求(例如,数据隐私、完全控制和成本),从而需要您自行托管,这种情况会更常出现。只是要确保这些相同的隐私要求不会阻止您使用用户数据进行微调!
Build evals and kickstart a data flywheel
构建评估并启动数据飞轮
Even teams that are just getting started need evals. Otherwise, you won’t know whether your prompt engineering is sufficient or when your fine-tuned model is ready to replace the base model.
即使是刚刚起步的团队也需要评估。否则,你将无法知道你的提示工程是否足够,或者你微调的模型何时可以替换基础模型。
Effective evals are specific to your tasks and mirror the intended use cases. The first level of evals that we recommend is unit testing. These simple assertions detect known or hypothesized failure modes and help drive early design decisions. Also see other task-specific evals for classification, summarization, etc.
有效的评估针对您的任务,反映了预期的使用案例。我们首先推荐的评估级别是单元测试。这些简单的断言可以检测已知或假设的故障模式,并有助于推动早期设计决策。另请参阅其他针对特定任务的评估,如分类、摘要等。
While unit tests and model-based evaluations are useful, they don’t replace the need for human evaluation. Have people use your model/product and provide feedback. This serves the dual purpose of measuring real-world performance and defect rates while also collecting high-quality annotated data that can be used to fine-tune future models. This creates a positive feedback loop, or data flywheel, which compounds over time:
虽然单元测试和基于模型的评估很有用,但它们不能取代人工评估的必要性。让人员使用您的模型/产品并提供反馈。这既衡量了实际工作表现和缺陷率,又收集了可用于微调未来模型的高质量标注数据,从而实现了双重目的。这形成了一个良性反馈循环,或者说数据飞轮,其效果会随时间而增强:
- Use human evaluation to assess model performance and/or find defects
使用人工评估来评价模型性能和/或查找缺陷
- Use the annotated data to fine-tune the model or update the prompt
使用注释数据来微调模型或更新提示
- Repeat 重复
For example, when auditing LLM-generated summaries for defects we might label each sentence with fine-grained feedback identifying factual inconsistency, irrelevance, or poor style. We can then use these factual inconsistency annotations to train a hallucination classifier or use the relevance annotations to train a reward model to score on relevance. As another example, LinkedIn shared about its success with using model-based evaluators to estimate hallucinations, responsible AI violations, coherence, etc. in its write-up.
例如,在审核LLM生成的摘要中的缺陷时,我们可能会给每个句子贴上精细的反馈标签,以识别事实不一致、不相关或风格不佳。然后,我们可以使用这些事实不一致的注释来训练一个幻觉分类器,或者使用相关性注释来训练一个奖励模型,以评估相关性。另一个例子是,LinkedIn 在其报告中分享了使用基于模型的评估器来估计幻觉、负责任的人工智能违规、连贯性等的成功经验。
By creating assets that compound their value over time, we upgrade building evals from a purely operational expense to a strategic investment and build our data flywheel in the process.
通过创建随时间增值的资产,我们将建筑评估从单纯的运营支出升级为战略投资,并在此过程中构建我们的数据飞轮。
The high-level trend of low-cost cognition
低成本认知的高级趋势
In 1971, the researchers at Xerox PARC predicted the future: the world of networked personal computers that we are now living in. They helped birth that future by playing pivotal roles in the invention of the technologies that made it possible, from Ethernet and graphics rendering to the mouse and the window.
1971 年,施乐帕克研究中心的研究人员预测了未来:我们如今所生活的联网个人电脑的世界。他们通过在发明使这一切成为可能的技术中扮演关键角色,帮助催生了这一未来,从以太网和图形渲染到鼠标和窗口。
But they also engaged in a simple exercise: they looked at applications that were very useful (e.g., video displays) but were not yet economical (i.e., enough RAM to drive a video display was many thousands of dollars). Then they looked at historic price trends for that technology (à la Moore’s law) and predicted when those technologies would become economical.
但是,他们也做了一项简单的练习:他们研究了一些非常有用(例如,视频显示)但尚未经济的应用,(也就是说,驱动视频显示所需的 RAM 要花费数千美元)。然后,他们研究了该技术的历史价格趋势(按照摩尔定律),并预测了这些技术何时会变得经济。
但是他们也进行了一项简单的练习:他们审视了那些非常有用(例如视频显示)但尚未经济实惠(即驱动视频显示所需的 RAM 成本高达数千美元)的应用。接着,他们研究了该技术的历史价格趋势(遵循摩尔定律),并预测了这些技术何时会变得经济实惠。
注:第二个版本对原文进行了更贴近中文表达习惯的翻译。
We can do the same for LLM technologies, even though we don’t have something quite as clean as transistors-per-dollar to work with. Take a popular, long-standing benchmark, like the Massively-Multitask Language Understanding dataset, and a consistent input approach (five-shot prompting). Then, compare the cost to run language models with various performance levels on this benchmark over time.
我们可以对LLM技术做同样的事情,尽管我们没有像每美元的晶体管数量那样清晰的指标可供参考。以一个流行且长期存在的基准测试为例,如大规模多任务语言理解数据集,并采用一致的输入方法(五次提示)。然后,随着时间的推移,比较在这个基准测试上运行具有不同性能水平的语言模型的成本。
我们可以对LLM技术做同样的事情,尽管我们没有像每美元的晶体管数量那样清晰的指标可供参考。以一个流行且长期存在的基准测试为例,如大规模多任务语言理解数据集,并采用一致的输入方法(五次提示)。然后,比较随着时间的推移,在这个基准测试上运行具有不同性能水平的语言模型的成本。
注意:由于原文中的"LLM"看起来像是一个占位符,我保留了它在翻译中。如果这是一个具体的数字或概念,可能需要根据上下文进行适当的翻译。
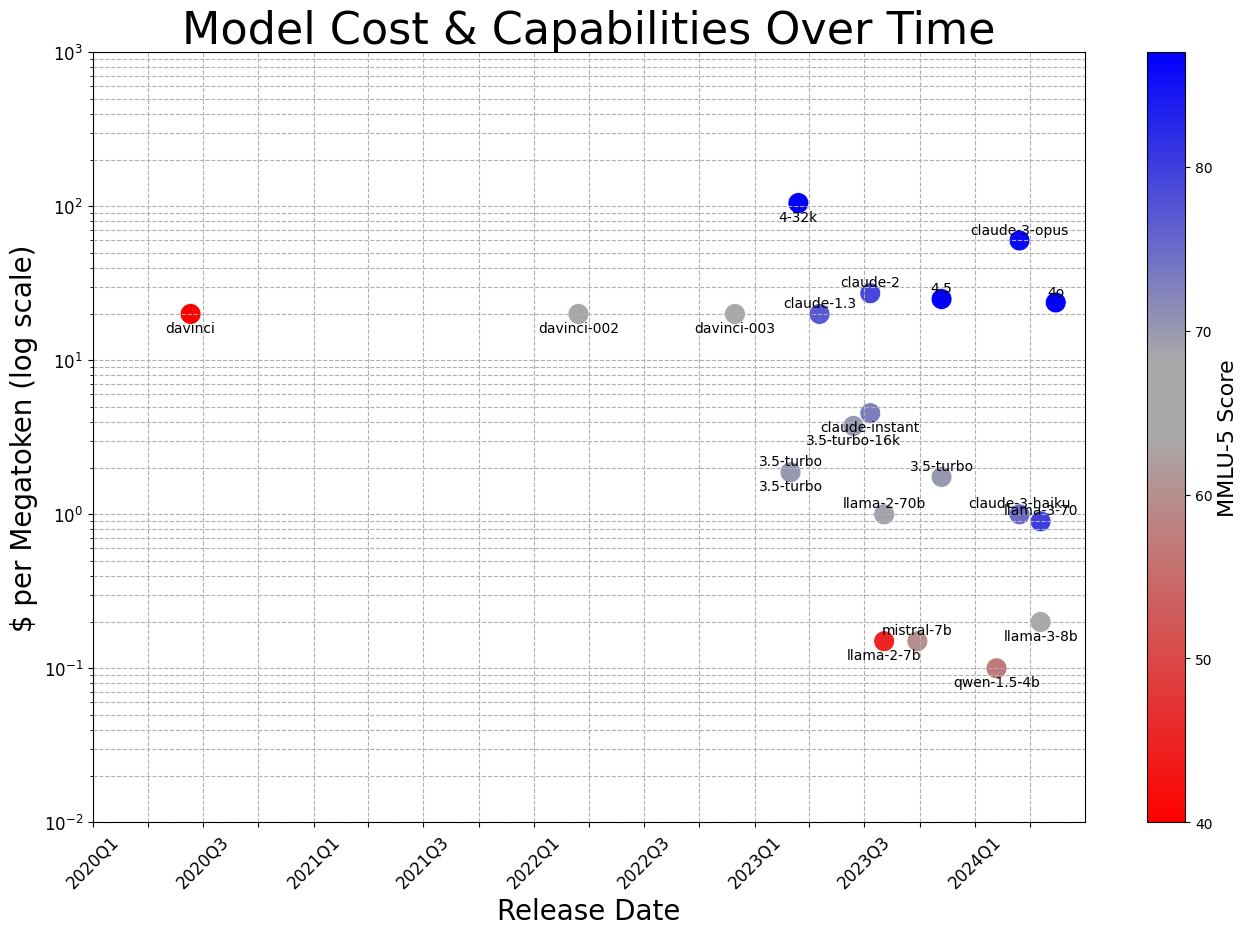
在固定成本下,能力正在迅速提高。在固定的能力水平下,成本正在迅速降低。由共同作者 Charles Frye 于 2024 年 5 月 13 日使用公开数据创建。
In the four years since the launch of OpenAI’s davinci model as an API, the cost for running a model with equivalent performance on that task at the scale of one million tokens (about one hundred copies of this document) has dropped from $20 to less than 10¢—a halving time of just six months. Similarly, the cost to run Meta’s LLama 3 8B via an API provider or on your own is just 20¢ per million tokens as of May 2024, and it has similar performance to OpenAI’s text-davinci-003, the model that enabled ChatGPT to shock the world. That model also cost about $20 per million tokens when it was released in late November 2023. That’s two orders of magnitude in just 18 months—the same time frame in which Moore’s law predicts a mere doubling.
自 OpenAI 的达芬奇模型作为 API 推出以来的四年里,以一百万令牌(大约相当于本文档的一百份副本)的规模运行具有相当性能的模型的成本,已从 20 美元降至不足 10 美分——成本减半的时间仅为六个月。同样,截至 2024 年 5 月,通过 API 提供商或自行运行 Meta 的 LLama 3 8B 的成本仅为每一百万令牌 20 美分,其性能与 OpenAI 的 text-davinci-003 相当,正是这个模型让 ChatGPT 在 2023 年 11 月底问世时震惊了世界。当时,该模型每一百万令牌的成本也约为 20 美元。在短短 18 个月内,成本下降了两个数量级——而这正是摩尔定律预测只会翻一番的时间框架。
Now, let’s consider an application of LLMs that is very useful (powering generative video game characters, à la Park et al.) but is not yet economical. (Their cost was estimated at $625 per hour here.) Since that paper was published in August 2023, the cost has dropped roughly one order of magnitude, to $62.50 per hour. We might expect it to drop to $6.25 per hour in another nine months.
现在,让我们考虑一个LLMs的应用,这个应用非常有用(如 Park 等人所做,为生成式视频游戏角色提供动力),但目前还不经济。(在这里,他们的成本估计为每小时 625 美元。)自从 2023 年 8 月该论文发表以来,成本已经下降了大约一个数量级,降至每小时 62.50 美元。我们可能预计在接下来的九个月内,成本会降至每小时 6.25 美元。
Meanwhile, when Pac-Man was released in 1980, $1 of today’s money would buy you a credit, good to play for a few minutes or tens of minutes—call it six games per hour, or $6 per hour. This napkin math suggests that a compelling LLM-enhanced gaming experience will become economical some time in 2025.
同时,当 1980 年吃豆人(Pac-Man)问世时,今天的 1 美元可以让你玩上几分钟或十几分钟——也就是说,每小时可以玩 6 局游戏,或者每小时花费 6 美元。这种粗略的计算表明,到 2025 年的某个时候,引人入胜的LLM增强型游戏体验将变得经济实惠。
注:原文中的LLM可能是特定的游戏名称或代号,由于没有具体信息,这里直接翻译为“LLM”。如果需要更准确的翻译,需要提供具体的游戏名称。
These trends are new, only a few years old. But there is little reason to expect this process to slow down in the next few years. Even as we perhaps use up low-hanging fruit in algorithms and datasets, like scaling past the “Chinchilla ratio” of ~20 tokens per parameter, deeper innovations and investments inside the data center and at the silicon layer promise to pick up slack.
这些趋势是新的,只有几年的历史。但在未来几年,几乎没有理由预期这一进程会放缓。即便我们在算法和数据集方面可能已经摘取了低垂的果实,比如超越了约 20 个标记每个参数的“毛丝鼠比率”,但在数据中心内部和硅层的更深层次的创新和投资有望接续上这一进程的松弛。
And this is perhaps the most important strategic fact: what is a completely infeasible floor demo or research paper today will become a premium feature in a few years and then a commodity shortly after. We should build our systems, and our organizations, with this in mind.
而这也许是最重要的战略事实:今天完全不可行的演示或研究论文,几年后将成为高端功能,然后很快就会变成商品。我们应该在这一点上构建我们的系统和组织。
Enough 0 to 1 Demos, It’s Time for 1 to N Products
足够的 0 到 1 演示,是时候进行 1 到 N 的产品开发了
We get it; building LLM demos is a ton of fun. With just a few lines of code, a vector database, and a carefully crafted prompt, we create ✨magic ✨. And in the past year, this magic has been compared to the internet, the smartphone, and even the printing press.
我们明白,构建LLM演示非常有趣。只需几行代码,一个向量数据库和一个精心设计的提示,我们就能创造✨魔法✨。而在过去的一年里,这种魔法被比作互联网,智能手机,甚至是印刷机。
Unfortunately, as anyone who has worked on shipping real-world software knows, there’s a world of difference between a demo that works in a controlled setting and a product that operates reliably at scale.
不幸的是,正如任何从事过实际软件开发的人都知道的那样,在受控环境下运行的演示与大规模可靠运行的产品之间存在着天壤之别。
Take, for example, self-driving cars. The first car was driven by a neural network in 1988. Twenty-five years later, Andrej Karpathy took his first demo ride in a Waymo. A decade after that, the company received its driverless permit. That’s thirty-five years of rigorous engineering, testing, refinement, and regulatory navigation to go from prototype to commercial product.
以自动驾驶汽车为例。1988 年,第一辆汽车由神经网络驱动。25 年后,安德烈·卡尔帕蒂(Andrej Karpathy)首次乘坐了 Waymo 的演示车。十年后,该公司获得了无人驾驶许可。这是从原型到商业产品,经过了 35 年的严格工程设计、测试、改进和法规导航。
Across different parts of industry and academia, we have keenly observed the ups and downs for the past year: year 1 of N for LLM applications. We hope that the lessons we have learned—from tactics like rigorous operational techniques for building teams to strategic perspectives like which capabilities to build internally—help you in year 2 and beyond, as we all build on this exciting new technology together.
在产业和学术界的各个领域,我们敏锐地观察到了过去一年的起伏:对于LLM应用来说,这是 N 年的第一年。我们希望,我们学到的经验教训——从建立团队的严格运营技巧等战术,到决定内部构建哪些能力等战略视角——能帮助你在第二年及以后的时间里,因为我们都在共同构建这项令人兴奋的新技术。
About the authors 关于作者
Eugene Yan designs, builds, and operates machine learning systems that serve customers at scale. He’s currently a Senior Applied Scientist at Amazon where he builds RecSys for millions worldwide and applies LLMs to serve customers better. Previously, he led machine learning at Lazada (acquired by Alibaba) and a Healthtech Series A. He writes & speaks about ML, RecSys, LLMs, and engineering at eugeneyan.com and ApplyingML.com.
尤金·燕设计、构建和运营服务于大规模客户的机器学习系统。目前,他是亚马逊的高级应用科学家,为全球数百万用户构建推荐系统,并应用LLMs来更好地服务客户。此前,他在 Lazada(被阿里巴巴收购)和一家健康科技 A 轮融资公司领导机器学习工作。他在 eugeneyan.com 和 ApplyingML.com 上撰写和演讲关于机器学习、推荐系统、LLMs和工程学的内容。
Bryan Bischof is the Head of AI at Hex, where he leads the team of engineers building Magic – the data science and analytics copilot. Bryan has worked all over the data stack leading teams in analytics, machine learning engineering, data platform engineering, and AI engineering. He started the data team at Blue Bottle Coffee, led several projects at Stitch Fix, and built the data teams at Weights and Biases. Bryan previously co-authored the book Building Production Recommendation Systems with O’Reilly, and teaches Data Science and Analytics in the graduate school at Rutgers. His Ph.D. is in pure mathematics.
布赖恩·比绍夫是 Hex 的 AI 负责人,他领导着构建 Magic 的工程师团队——数据科学和分析辅助工具。布赖恩在数据栈的各个领域工作过,领导过分析、机器学习工程、数据平台工程和 AI 工程团队。他在 Blue Bottle Coffee 创立了数据团队,领导了 Stitch Fix 的多个项目,并在 Weights and Biases 建立了数据团队。布赖恩之前与人合著了 O'Reilly 的《构建生产推荐系统》一书,并在罗格斯大学的研究生院教授数据科学和分析。他拥有纯数学博士学位。
Charles Frye teaches people to build AI applications. After publishing research in psychopharmacology and neurobiology, he got his Ph.D. at the University of California, Berkeley, for dissertation work on neural network optimization. He has taught thousands the entire stack of AI application development, from linear algebra fundamentals to GPU arcana and building defensible businesses, through educational and consulting work at Weights and Biases, Full Stack Deep Learning, and Modal.
查尔斯·弗莱教授人们构建人工智能应用。在精神药理学和神经生物学领域发表研究后,他在加利福尼亚大学伯克利分校攻读博士学位,论文主题是神经网络优化。他通过在“权重与偏差”、“全栈深度学习”和“Modal”等机构的教育和咨询工作,教授了数千人从线性代数基础到 GPU 奥秘,再到构建可防御的业务的整个人工智能应用开发栈。
Hamel Husain is a machine learning engineer with over 25 years of experience. He has worked with innovative companies such as Airbnb and GitHub, which included early LLM research used by OpenAI for code understanding. He has also led and contributed to numerous popular open-source machine-learning tools. Hamel is currently an independent consultant helping companies operationalize Large Language Models (LLMs) to accelerate their AI product journey.
哈梅尔·侯赛因是一位拥有超过 25 年经验的机器学习工程师。他曾与 Airbnb 和 GitHub 等创新公司合作,其中包括早期LLM研究,这些研究被 OpenAI 用于代码理解。他还领导并为许多流行的开源机器学习工具做出了贡献。哈梅尔目前是一位独立顾问,帮助公司实现大型语言模型(LLMs)的运营,以加速其 AI 产品的发展历程。
Jason Liu is a distinguished machine learning consultant known for leading teams to successfully ship AI products. Jason’s technical expertise covers personalization algorithms, search optimization, synthetic data generation, and MLOps systems.
Jason Liu 是一位杰出的机器学习顾问,以领导团队成功推出 AI 产品而闻名。Jason 的技术专长涵盖了个性化算法、搜索优化、合成数据生成和 MLOps 系统。
His experience includes companies like Stitch Fix, where he created a recommendation framework and observability tools that handled 350 million daily requests. Additional roles have included Meta, NYU, and startups such as Limitless AI and Trunk Tools.
他的经验包括在 Stitch Fix 等公司工作,他在那里创建了一个推荐框架和可观测性工具,处理了每天 3.5 亿次的请求。他的其他角色还包括在 Meta、纽约大学以及 Limitless AI 和 Trunk Tools 等初创公司工作。
Shreya Shankar is an ML engineer and PhD student in computer science at UC Berkeley. She was the first ML engineer at 2 startups, building AI-powered products from scratch that serve thousands of users daily. As a researcher, her work focuses on addressing data challenges in production ML systems through a human-centered approach. Her work has appeared in top data management and human-computer interaction venues like VLDB, SIGMOD, CIDR, and CSCW.
Shreya Shankar 是加州大学伯克利分校的机器学习工程师和计算机科学博士生。她是两家初创公司的首位机器学习工程师,从零开始构建人工智能驱动的产品,每天为数千名用户提供服务。作为一名研究人员,她的工作重点是通过以人为本的方法解决生产机器学习系统中的数据挑战。她的研究成果在 VLDB、SIGMOD、CIDR 和 CSCW 等顶级数据管理和人机交互领域发表。
Contact Us 联系我们
We would love to hear your thoughts on this post. You can contact us at contact@applied-llms.org. Many of us are open to various forms of consulting and advisory. We will route you to the correct expert(s) upon contact with us if appropriate.
我们非常想听听您对这篇文章的看法。您可以通过 contact@applied-llms.org 联系我们。我们中的许多人愿意接受各种形式的咨询和顾问工作。如果合适,您联系我们后,我们会将您转给合适的专家。
Acknowledgements 重试 错误原因
This series started as a conversation in a group chat, where Bryan quipped that he was inspired to write “A Year of AI Engineering”. Then, ✨magic✨ happened in the group chat (see image below), and we were all inspired to chip in and share what we’ve learned so far. 重试 错误原因
The authors would like to thank Eugene for leading the bulk of the document integration and overall structure in addition to a large proportion of the lessons. Additionally, for primary editing responsibilities and document direction. The authors would like to thank Bryan for the spark that led to this writeup, restructuring the write-up into tactical, operational, and strategic sections and their intros, and for pushing us to think bigger on how we could reach and help the community. The authors would like to thank Charles for his deep dives on cost and LLMOps, as well as weaving the lessons to make them more coherent and tighter—you have him to thank for this being 30 instead of 40 pages! The authors appreciate Hamel and Jason for their insights from advising clients and being on the front lines, for their broad generalizable learnings from clients, and for deep knowledge of tools. And finally, thank you Shreya for reminding us of the importance of evals and rigorous production practices and for bringing her research and original results to this piece. 重试 错误原因
Finally, the authors would like to thank all the teams who so generously shared your challenges and lessons in your own write-ups which we’ve referenced throughout this series, along with the AI communities for your vibrant participation and engagement with this group.
最后,作者想感谢所有团队,你们慷慨地在自己的文章中分享了挑战和经验,我们在本系列中多次引用了这些内容,同时感谢人工智能社区的各位,你们积极参与并与这个群体互动。
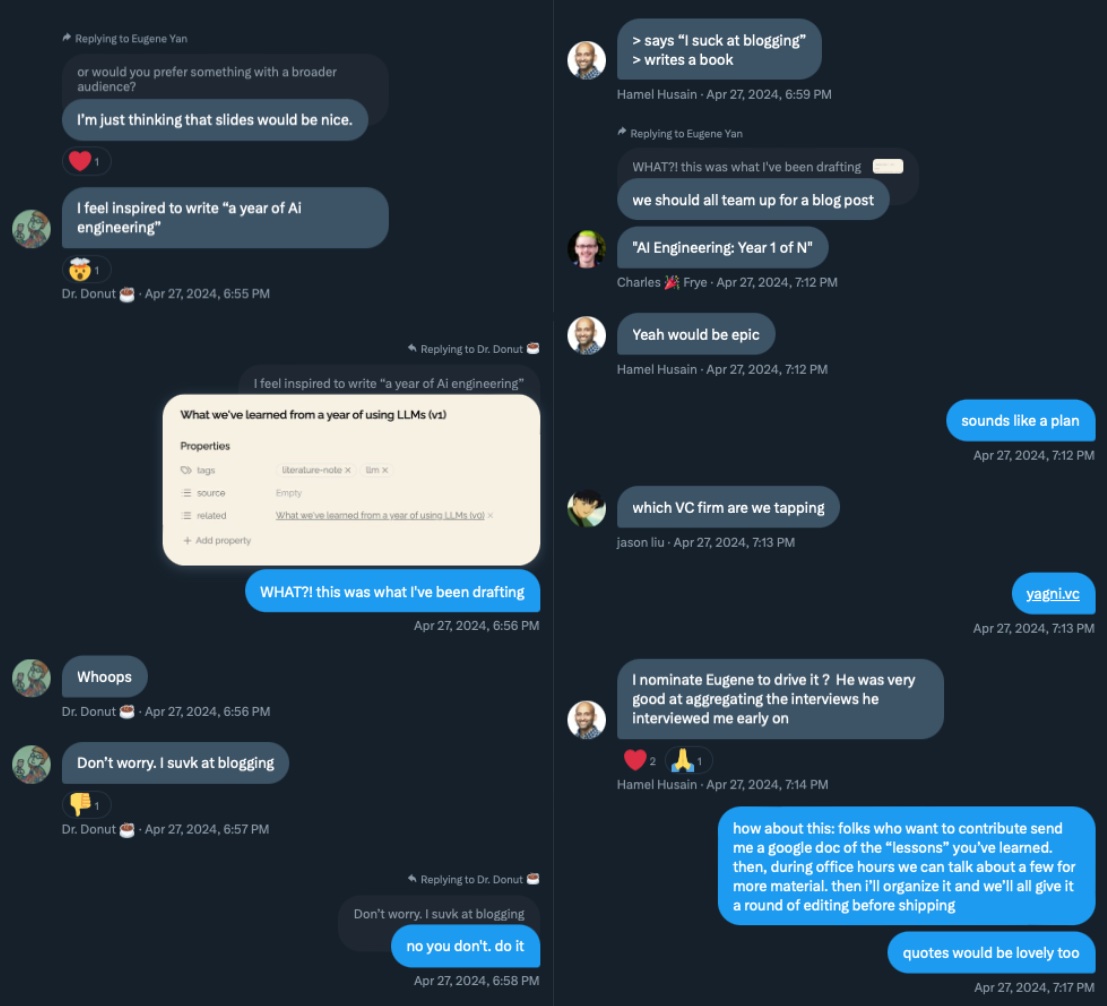